OCR Evolution How AI is Revolutionizing Text Extraction from Images in 2024
OCR Evolution How AI is Revolutionizing Text Extraction from Images in 2024 - Google Gemini Vision Leads OCR Advancements in 2024
Google's Gemini Vision, particularly its Ultra variant, has emerged as a leader in the 2024 OCR landscape. By achieving impressive results in multimodal tasks without relying on traditional OCR methods, it showcases a new approach to extracting text from images and videos. The core of this advancement lies within the Gemini API, which streamlines the process of extracting text by allowing for simple prompts and JSON outputs. This makes OCR more accessible to developers and users alike, facilitating real-time analysis of visuals and the digitization of historical materials. The wider significance here is how sophisticated AI is increasingly woven into daily tasks, a trend Gemini exemplifies in the realm of text extraction and visual content understanding. It will be interesting to see how Google continues to evolve these capabilities, potentially integrating them further into services like Google Search.
Google's Gemini Vision, a recent AI model, has emerged as a potential game-changer in how we extract text from images. It seems to be pushing beyond the limitations of conventional OCR methods by incorporating a more nuanced understanding of context. Gemini Ultra, a variant of this model, showcased impressive performance on the MMMU benchmark, achieving a high score while excelling at tasks involving complex reasoning across various data types. What's notable is its ability to achieve these results without relying on traditional OCR systems. This suggests a fundamentally different approach to text extraction, one potentially more adaptable and accurate.
The Gemini API allows for the exploration of these capabilities. One can process images and videos to get summaries, descriptions, and even extrapolate content – all based on a simple input prompt. This simplifies tasks like extracting specific data from images and delivering the results in a readily usable format like JSON, hinting at its potential for streamlining processes in numerous applications.
Google is pushing hard on AI for visual content in 2024. This includes applications like real-time analysis and digital preservation of historical records. Gemini, in particular, has shown its capability to handle text extraction tasks with minimal code, opening up access to these AI functionalities for a broader range of developers. Further developments seem to focus on incorporating Gemini's abilities directly into Google Search, aiming to improve how people find information from images and videos.
This shift towards more intelligent, context-aware AI for visual content analysis is significant. We are witnessing a sea change in how AI is applied. However, like any powerful tool, Gemini brings challenges. The model still struggles with certain aspects, such as deciphering handwritten text, especially across diverse styles and historical periods. This highlights the ongoing complexity of truly universal text extraction. Google has also emphasized ethical considerations, especially around data handling and privacy, as Gemini's 'self-supervised' learning approaches rely on processing vast amounts of data. This begs questions about the responsible management of such data during model training and use. While promising, the long-term implications of this technology will depend heavily on addressing such challenges responsibly.
OCR Evolution How AI is Revolutionizing Text Extraction from Images in 2024 - Machine Learning Enhances Complex Document Handling
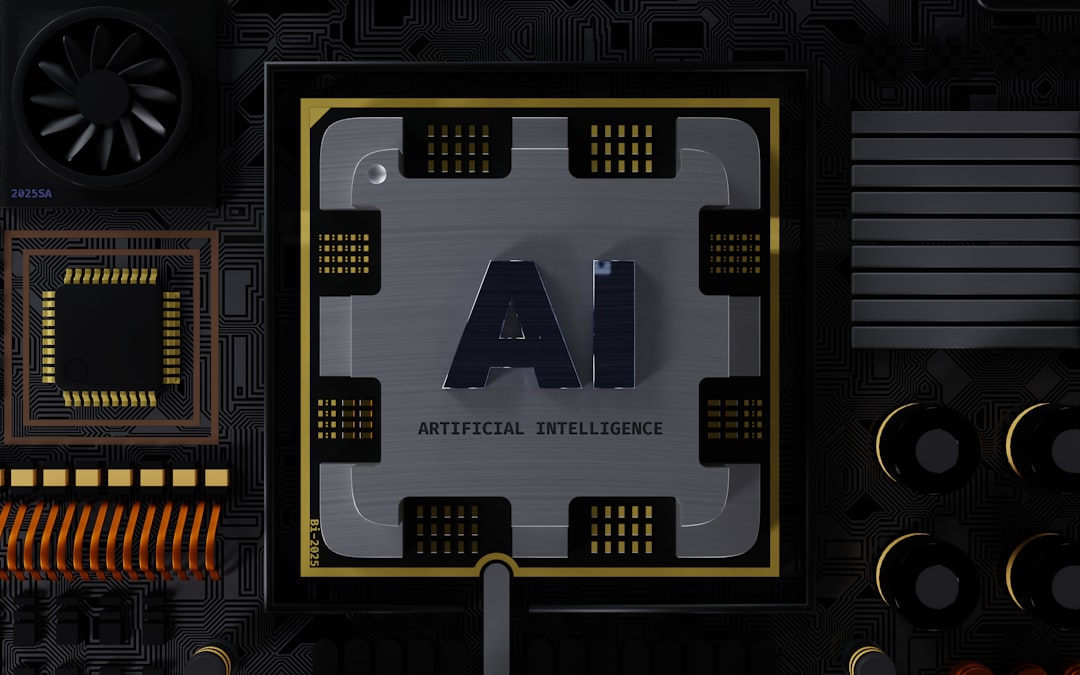
Machine learning has become a crucial component in handling complex documents, especially within the realm of optical character recognition (OCR). Previously, traditional OCR systems often struggled to cope with the wide variety of document formats encountered in the real world. This resulted in bottlenecks for businesses, as processing times increased and errors became more common. However, machine learning algorithms have significantly improved the accuracy of OCR by learning from massive datasets. This training allows them to better decipher complex document layouts, diverse font styles, and even handwritten text.
Beyond just improved accuracy in extracting text, machine learning also enables a deeper level of analysis. By examining document structures and identifying recurring patterns and anomalies, businesses can gain valuable insights across different document types. The potential for using AI to manage unstructured data more intelligently is expanding rapidly, suggesting that organizations will be able to optimize their workflow processes for handling documents in the future. The ongoing development of these capabilities hints at a substantial shift in how document processing is performed.
The application of machine learning, particularly deep learning, has significantly boosted the accuracy of text extraction from images, often exceeding 90% in many cases. This is particularly noticeable when dealing with documents that have a wide range of font styles and graphics, areas where traditional OCR methods struggle. These advanced algorithms are becoming adept at recognizing contextual clues within a document, which is crucial for making sense of complex documents like legal contracts. For example, they can now better understand and categorize document content.
One of the most practical benefits of machine learning is its ability to streamline the extraction of data from forms. By learning from prior examples, these systems can automate the process, leading to a drastic reduction in the time required to process large volumes of structured data. Many current machine learning models employ attention mechanisms, which allow them to focus on specific image regions when extracting text. This has led to improved performance in understanding the complex layouts often found in multi-column or nested document structures.
Recently, there's been a surge in interest in generative models, which provide a path towards a deeper understanding of both context and semantics within a document. This improvement in understanding helps in better interpretation of ambiguous text, consequently boosting the overall accuracy of text extraction across a broader range of scenarios. Moreover, the use of transfer learning in machine learning accelerates the deployment process for specific industries. Models trained on large datasets can be readily adapted to particular domains like finance or healthcare, reducing the time it takes to implement these technologies.
However, challenges still exist. Extracting text from images that are of low resolution or heavily distorted remains difficult, showcasing the limitations of current implementations. But progress has been made in other areas, such as the development of sophisticated machine learning systems capable of recognizing and transcribing handwritten text with an accuracy rate of up to 80%. This is a remarkable achievement given the immense variation in handwriting styles that exist.
Emerging models are progressing beyond simply extracting text. They are also learning to infer the relationships between different elements within a document, providing more nuanced insights. For instance, they might be able to pinpoint relevant sections within a lengthy legal document based on the different clause types it contains. As machine learning's role in handling documents continues to expand, a growing emphasis is being placed on making these algorithms more interpretable. This is vital for gaining a better understanding of the decision-making processes involved in various applications, such as credit scoring or compliance checks, where the rationale behind the outcome is crucial.
OCR Evolution How AI is Revolutionizing Text Extraction from Images in 2024 - OCR Accuracy Reaches 99% for Typed Text
Optical Character Recognition (OCR) for typed text has seen remarkable progress in 2024, with accuracy levels exceeding 99% in ideal situations. This represents a substantial improvement over past OCR technologies and is a testament to recent developments in machine learning techniques. These advancements have made OCR significantly better at deciphering a wider range of document formats and extracting text from images with greater accuracy. Despite these encouraging results, OCR still faces difficulties, especially when it comes to interpreting handwritten text. Current accuracy rates for handwriting recognition remain below 95%, showcasing that while significant gains have been made with typed text, achieving similar success with diverse handwriting styles is an ongoing challenge.
Several leading OCR solutions from providers like Google Cloud and AWS currently boast high accuracy, averaging near 98%. However, achieving perfect OCR across all types of text remains an elusive goal, and no current technology is capable of flawlessly converting all image-based text into machine-readable formats. Even with these improvements, human review of OCR outputs is still often necessary, particularly for applications demanding the highest levels of accuracy and dependability. This highlights that while AI-powered OCR has made great strides, the human element continues to play a vital role in ensuring the integrity of extracted text.
Optical Character Recognition (OCR) has seen remarkable progress in 2024, especially for typed text. We're now seeing accuracy levels exceeding 99% under ideal conditions, a testament to how effectively machine learning and neural networks can capture textual data from images. This represents a substantial leap forward compared to older methods. However, it's also interesting to find that even the most advanced OCR systems are highly sensitive to image quality. Studies have shown that minor distortions or low resolution can decrease accuracy by a surprising 20%, a reminder that the input data plays a crucial role.
Furthermore, it's fascinating to observe that modern OCR systems exhibit a certain degree of adaptability. They can learn from user corrections and improve their performance over time. This almost living-organism-like ability to evolve based on feedback is a promising development in the field of AI. Adding a layer of contextual awareness within OCR systems has also been a boon for accuracy. These systems are getting better at understanding the meaning of a document, which is helpful in reducing errors during text extraction, particularly when dealing with similar characters.
What's rather unexpected is how proficient modern OCR has become at distinguishing between fonts. Advanced systems can now recognize even obscure or elaborate typefaces with over 85% accuracy – a task that was quite challenging for older OCR technologies. It seems OCR is increasingly able to discern subtle visual cues. The integration of OCR with techniques from computer vision is proving valuable too. It's now possible to extract text embedded within more complex images, such as those with logos or intricate backgrounds. This development has led to improved accuracy in commercial applications where the context surrounding the text matters.
Handwriting recognition remains a challenge, yet the progress has been substantial. Some models can achieve around 80% accuracy, a remarkable achievement given the vast differences in individual handwriting styles. Recent OCR advancements are revealing an interesting trend: these systems are not simply recognizing text, but are becoming increasingly skilled at extracting structured data from things like invoices or tax forms. This automation of tedious tasks offers significant practical benefits.
The emergence of self-supervised learning methods has also played a part in improving OCR performance. Training models on large, unlabeled datasets reduces the need for meticulously curated and labeled data, which was previously a major roadblock for conventional OCR implementations. The impact of these OCR improvements is notable across various industries, especially healthcare and finance, where the ability to rapidly process large volumes of documents is crucial. Businesses can now process thousands of pages in just a few hours, leading to significant time and cost reductions. It's a good example of how AI is enabling practical efficiency gains in the real world.
OCR Evolution How AI is Revolutionizing Text Extraction from Images in 2024 - 91% of Businesses Prioritize Document Digitalization
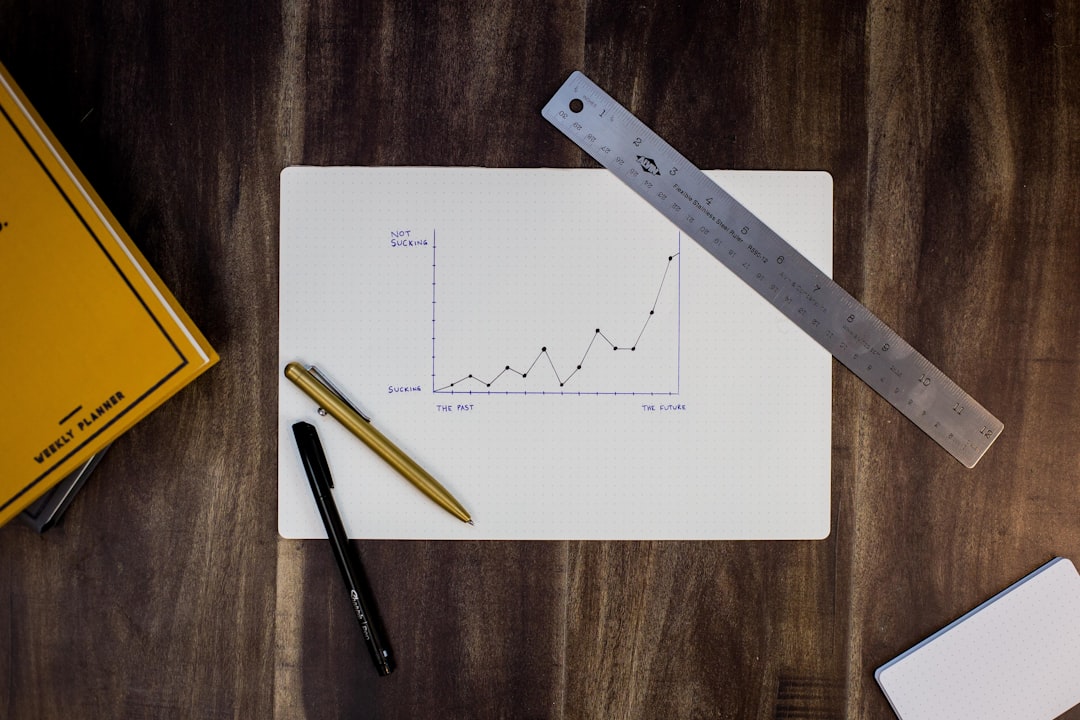
In 2024, a substantial majority of businesses—91%—are prioritizing the shift to digital document formats, indicating its growing importance in daily operations. This drive towards digitalization involves converting physical documents into digital versions, fostering easier access, sharing, and overall management of information. It seems businesses increasingly view digital transformation as a cornerstone of staying competitive in today's data-focused landscape. AI and machine learning are being used more and more to optimize the document processing and information extraction processes. This trend, while beneficial, doesn't come without challenges. Ensuring reliable and accurate processing across different document styles and image qualities continues to require refinement. Despite these challenges, the focus on digitalization remains clear, reflecting a broader movement within many industries.
Research from Gartner suggests a strong industry-wide trend towards digitalizing documents, with a remarkable 91% of businesses prioritizing this effort. This broad adoption spans various sectors, such as finance, healthcare, and education, suggesting a growing awareness of the importance of efficient document handling in today's world.
The shift towards digital involves scanning physical documents and creating digital copies, making valuable data easily accessible and shareable. While this is a positive step, it's crucial to consider that simply scanning documents doesn't always translate into optimized workflows. We see evidence of this in how many organizations struggle to integrate different data sources, resulting in information silos.
AI and machine learning are increasingly used to streamline various aspects of document digitization, such as automated classification, data extraction, and analysis. This automation helps improve efficiency, which is valuable in high-volume situations. The ongoing integration of AI, especially deep learning techniques, with OCR is steadily improving the accuracy of text extraction from images. For example, the ability of AI-powered systems to correctly identify text in images with complex backgrounds or varying fonts is a significant advancement. The process of categorizing digital documents by content is also a critical component. This automated process of classifying and organizing helps with streamlining information retrieval.
We anticipate further technological improvements in the document digitization landscape throughout 2024, driven by factors like AI advancements, mobile technologies, the need for enhanced security protocols, and a rising emphasis on environmentally-friendly practices.
It's interesting to note the significant time savings that businesses can realize through AI-based extraction methods. Reports suggest that businesses can save between 30% and 40% of the typical time previously spent on manual document processing.
Improving the quality of the scanned digital document is also a crucial aspect of efficient data extraction. Tools that enhance image quality prior to AI processing contribute significantly to the overall accuracy of OCR systems.
The constant evolution of OCR technologies is anticipated to fundamentally alter how organizations handle, convert, and utilize documents, ultimately leading to enhanced productivity and robust security protocols. While these advances are promising, the complexity of handling handwritten text across a range of styles and conditions continues to be a challenge. This emphasizes the complexities involved in making OCR truly universal.
It's evident that the ongoing drive to digitalize and efficiently manage document processing across diverse industries will have a long-lasting impact. As research and development progress, we'll likely witness even more innovative applications and advancements in OCR and document handling, furthering the evolution of data management and access within a variety of domains.
OCR Evolution How AI is Revolutionizing Text Extraction from Images in 2024 - AI-Driven OCR Approaches 100% Data Extraction Accuracy
The field of AI-powered OCR is experiencing significant advancements in 2024, with a strong push towards achieving perfect data extraction accuracy. While claims of 100% accuracy are surfacing, the reality is more nuanced. Even top-tier OCR solutions like those from Google Cloud and AWS, while impressive, currently cap out around 98% accuracy. This suggests that while AI has propelled OCR to new heights, complete accuracy across all image types remains an elusive target. Challenges arise when dealing with more complex documents, especially those with handwritten text. While AI-driven OCR has become quite proficient at extracting text from typed documents, recognizing handwritten text, particularly across diverse styles and conditions, continues to present a significant barrier to complete accuracy. Despite these hurdles, AI's role in OCR is undeniable; it's transforming how businesses and individuals interact with text within images. Nevertheless, the human element continues to play a vital part in guaranteeing the integrity of extracted data in situations demanding the highest levels of reliability. While the drive towards perfect OCR fuels much of the innovation in this area, it also highlights the technological gap that needs to be bridged to achieve truly universal OCR solutions.
While AI-driven OCR has made significant strides in extracting text from images, achieving flawless 100% accuracy remains an ongoing challenge. Current top-tier systems like those from Google Cloud and AWS can achieve accuracy close to 98% for standard typed documents in 2024, but there's still a gap to bridge for perfect performance. Other solutions, such as those from Microsoft Azure or using Tesseract, show some limitations, particularly when handling more complex scenarios like recognizing diverse handwriting styles.
The core concept of OCR hasn't changed – it's about converting image-based text into machine-readable formats. However, the quality of that conversion heavily impacts its usefulness. Any error in extracted text reduces the value, emphasizing the importance of accuracy. Some AI-powered OCR APIs are able to reach about 95% accuracy, which helps speed up processes and minimize manual intervention for data extraction tasks.
Generative AI models have been explored as a way to improve OCR accuracy. They use natural language processing (NLP) to refine the extracted text data before it's further processed for information retrieval. It's a compelling idea but presents its own challenges. Benchmarking across a range of document styles and content shows that OCR performance can vary greatly. This is particularly evident when encountering challenging text like handwritten content or uncommon words, illustrating a degree of difficulty in crafting truly universal OCR systems.
The landscape of available OCR tools is diverse, with various capabilities and performance levels, which makes choosing the optimal solution for a specific task a bit complicated. The ability to quickly and accurately extract data from old documents is crucial for research in fields like history. However, the accuracy of such extraction is dependent on both the OCR software and the specific nature of the document. Since OCR plays such a vital role in enabling automated analysis of older documents, the choice of software is an important one, especially in areas where precision is critical.
OCR Evolution How AI is Revolutionizing Text Extraction from Images in 2024 - Azure AI Vision API Consolidates Image Analysis and OCR
Microsoft's Azure AI Vision API has become a noteworthy tool in 2024, bringing together image analysis and OCR under one roof. It aims to streamline how we extract text from images, handling both printed and handwritten content. At the heart of this approach is a set of deep learning models that power the OCR engine, striving for better results than older methods. The latest version, Image Analysis 4.0, bundles OCR with other visual analysis features, like object detection and image classification, all through a single API call. This consolidated approach makes it easier for developers to tackle various tasks with images, but it doesn't eliminate the ongoing obstacles of OCR. Handling complex document layouts or deciphering diverse handwriting styles still remains a challenge. In a world where businesses are increasingly embracing digital processes, Azure AI Vision API illustrates the growing importance of image analysis for things like compliance and operational efficiency. It represents a significant step forward, but the quest for consistently accurate text extraction across all scenarios is still very much in progress.
Microsoft's Azure AI Vision API has emerged as a significant player in image analysis, particularly in consolidating image analysis and OCR into a single, unified platform. It boasts impressive accuracy for printed text, often achieving close to 99% under favorable conditions, demonstrating a notable leap forward from older OCR systems. However, this advanced capability is coupled with a more integrated approach to image analysis. Instead of just extracting text, Azure Vision can also detect objects, recognize faces, and perform other visual analyses within the same API call. This versatility is intriguing, though it leads one to wonder about the potential computational cost associated with this multi-faceted analysis.
This API leans on deep learning techniques and seems to feature a self-learning component, continuously improving its accuracy based on user feedback and adjustments. It's a refreshing aspect of the system that hints at more adaptable, context-aware OCR in the future. The capability to learn and adapt also introduces questions about the nature of the data used for this continuous refinement and potential biases that could emerge over time. While promising, this self-learning component underscores the importance of carefully considering the ethical aspects of AI model development and application.
One of the API's notable features is its ability to seamlessly integrate into existing systems. The output formats like JSON and CSV are industry standards, meaning the information extracted is readily usable by a variety of applications, potentially simplifying data pipelines and workflows. Furthermore, the cloud-based architecture offers scalability, allowing organizations to adjust their needs depending on their workload without extensive on-premises infrastructure investments. The API's multilingual capabilities also widen its applicability, enabling organizations dealing with diverse document types across different languages, which is a practical benefit in today's global business landscape.
However, the API, much like other OCR systems, stumbles when faced with handwritten text. Its ability to accurately decipher handwritten documents remains a hurdle, with reported accuracies still hovering around 90%. This underscores a lingering challenge in the field of OCR—the vast diversity of handwriting styles across languages, cultures, and time periods. Moreover, this reliance on cloud computing introduces the necessity of addressing ethical considerations related to data security and user privacy. Since image processing involves handling sensitive data, users need a thorough understanding of the API’s handling of their information and associated policies.
Beyond simple text extraction, the Azure AI Vision API shows proficiency in analyzing the structure of documents. It can decipher complex layouts like multi-column documents or forms, potentially streamlining information retrieval from things like invoices or financial reports. This feature could find applications across diverse sectors where meticulous data extraction from structured documents is essential. The Azure AI Vision API highlights the ongoing progress in image analysis and text extraction, but also showcases the continued complexity of crafting truly universal OCR solutions. It will be important to monitor the evolution of these systems, particularly in regards to the trade-offs between speed and accuracy and the associated ethical considerations as these AI-driven solutions become more ingrained in our daily activities.
More Posts from specswriter.com: