Transform your ideas into professional white papers and business plans in minutes (Get started for free)
AI-Powered Image Upscaling Enlarging Photos Without Quality Loss in 2024
AI-Powered Image Upscaling Enlarging Photos Without Quality Loss in 2024 - Neural Networks Revolutionize Pixel Generation
The landscape of image enhancement has been dramatically reshaped by neural networks, specifically in the realm of pixel generation. While older methods of upscaling, like simple interpolation, often lead to blurry or pixelated results when enlarging images, neural networks offer a superior approach. These sophisticated networks can intelligently analyze the intricate details within an image and generate new pixels that seamlessly integrate, rather than merely duplicating existing ones. This capability leads to significantly enhanced image quality without the common compromises of the past. We're seeing evidence of this shift in tools like ON1's Resize AI and Canon's neural network-based upscaling, which can deliver high-resolution results crucial for tasks like large-format printing and image cropping. The potential of neural networks in this space is substantial, paving the way for a future where upscaling preserves detail and enhances visual fidelity as a standard practice. There are still challenges, as perfect pixel generation is not quite achieved, but the improvements are substantial, especially considering the limitations that existed just a few years ago.
The core of this new wave of image upscaling lies within the intricate designs of neural networks. These networks, built upon layers that process spatial information, are able to learn complex relationships within images. They effectively decipher patterns and textures, enabling them to generate convincingly detailed pixels that weren't present in the original low-resolution input. This is a far cry from older methods that simply interpolated pixels, leading to a blurry and often unrealistic result.
The concept of "deep learning" is instrumental here. It's the ability of a network with many layers to analyze image data at various levels of abstraction. This hierarchical processing allows neural networks to extract intricate information, ultimately resulting in better reconstructions compared to what traditional approaches can achieve. We've also seen exciting progress with Generative Adversarial Networks (GANs). In this setup, two neural networks compete, one creating images and the other evaluating their realism. Through this adversarial relationship, the generated images get progressively more believable.
It's remarkable how neural networks can even capture physical aspects like lighting and shadows when generating new pixels. This allows for the generation of upscaled images that not only appear larger but also retain a sense of depth and realism. They're also increasingly adept at identifying and removing common artifacts found in low-resolution images, further improving the quality and authenticity of the final product.
Furthermore, the flexibility of neural networks allows for intriguing techniques like style transfer. In essence, this allows an image's content to be merged with the aesthetic qualities of another, leading to new avenues for artistic expression and pixel manipulation. However, the complexity of these networks can also pose a challenge. While the results are often stunning, figuring out exactly how they arrive at specific pixel enhancements remains an area of active research and engineering.
The performance of neural networks is also intricately linked to the breadth and quality of the data they're trained on. Larger, more diverse datasets enable the networks to develop a more general understanding of images, improving their ability to handle a variety of subject matter. There's a clear connection between the amount of training data and the effectiveness of the resulting upscaling algorithms.
Finally, advances in computational resources and algorithm design have brought down the processing time associated with neural network upscaling. We're now seeing applications where these methods can be used in real-time, a significant improvement over earlier generations that required a considerable amount of processing power. This makes them increasingly practical for a wider range of applications.
AI-Powered Image Upscaling Enlarging Photos Without Quality Loss in 2024 - Bilinear and Bicubic Interpolation Limitations
Bilinear and bicubic interpolation, while established methods for resizing images, have inherent drawbacks when it comes to enlarging them. Bilinear interpolation, based on averaging surrounding pixels in a 2x2 area, frequently leads to a noticeable blurring effect. Bicubic interpolation, employing a larger 4x4 grid, produces somewhat smoother outcomes, but still falls short of the detail preservation achievable with AI-driven methods. The core issue with these techniques is their reliance on estimations rather than the generation of entirely new pixels. This limitation ultimately compromises image sharpness and clarity, especially when upscaling. In contrast, the advancements in AI-powered image upscaling have ushered in a new era where the creation of novel pixels is possible, resulting in higher-quality, more detailed enlargements. As the need for high-resolution images continues to increase, these innovations mark a departure from traditional interpolation, presenting a superior path for maintaining and enhancing image fidelity during resizing.
While bilinear and bicubic interpolation are established methods for image resizing, they come with inherent limitations when it comes to achieving high-quality enlargements. One major drawback is their struggle with preserving fine details, like sharp edges or intricate textures. This often results in a loss of clarity, as these methods are essentially estimating missing pixels rather than creating new ones, a stark contrast to how neural networks operate.
Another challenge arises when dealing with images that have sparse pixel distributions or areas with irregular features. Interpolation techniques can introduce artifacts like ringing or blurring in these situations, making them less suitable for complex images where detailed preservation is crucial. This is a consequence of their reliance on a uniform distribution of weights across neighboring pixels, which can over-smooth areas that might require more nuanced detail retention. In contrast, AI-powered methods can intelligently assign different weights based on learned patterns.
Furthermore, these traditional approaches are particularly sensitive to noise present in low-resolution images. This leads to a more pronounced presence of artifacts in the upscaled results, making them less desirable for scenarios where noise reduction is essential. AI methods, on the other hand, actively learn to distinguish between actual image content and noise during training, resulting in cleaner outputs.
In real-world applications, particularly those requiring high-resolution outputs for professional tasks like printing and large-screen displays, bilinear and bicubic interpolation often fall short of the capabilities of AI methods. Their limitations in this space have raised questions about their continuing relevance in modern image processing.
A crucial difference lies in their lack of contextual awareness and understanding of imagery. While neural networks can leverage this context to create a more cohesive and visually appealing result, these older techniques lack this ability, resulting in output that might seem less natural or realistic.
Even though AI-driven methods can be computationally demanding, bilinear and bicubic methods aren't always a speedier alternative. When dealing with large images, their performance can still be slow, particularly as they can't leverage the powerful capabilities of modern GPUs that accelerate AI-driven upscaling. This aspect points to a possible future where AI's computational benefits overtake other considerations.
Furthermore, unlike AI methods, which are continuously learning and adapting through the use of massive datasets, these older interpolation methods are static and cannot improve over time with use. Their lack of a learning mechanism limits their ability to adapt to different image styles and subject matters, which further differentiates them from AI upscaling methods.
Lastly, traditional techniques can produce visually undesirable outcomes in certain situations. Bilinear interpolation is especially prone to creating a blocky appearance in areas with significant changes in color or gradients, while bicubic interpolation can exacerbate some artifacts, leading to unnatural-looking upscaled images. These issues are often addressed by more sophisticated AI approaches.
Finally, another major limitation of interpolation is their tendency to struggle with images that possess non-uniform properties, like varying textures and colors throughout. This limitation frequently calls for a transition to more advanced techniques capable of adapting to these diverse characteristics, solidifying the argument for neural network solutions in many scenarios.
These limitations emphasize that, while the techniques are foundational to image processing, their shortcomings become more pronounced when compared to the increasing sophistication of AI-powered upscaling methods. In many applications, the results produced by AI show a clear advantage, prompting a reconsideration of the role these older methods play in the future of image enhancement.
AI-Powered Image Upscaling Enlarging Photos Without Quality Loss in 2024 - PhotoRoom and AVCLabs Lead AI Upscaling Tools
PhotoRoom and AVCLabs are prominent players in the expanding field of AI-driven image upscaling in 2024. These tools leverage advanced artificial intelligence to enlarge images while preserving, and often enhancing, visual quality. They accomplish this by intelligently creating new pixels that seamlessly blend into the existing image structure, leading to improved resolution and detail. Beyond simply increasing size, both PhotoRoom and AVCLabs empower users with tools to adjust image elements like contrast and saturation, allowing for greater control over the final appearance. AVCLabs offers a user-friendly, free online upscaling service that handles a wide array of image formats. PhotoRoom provides a more specialized feature set, including batch processing for efficiently removing backgrounds from multiple images. While these tools represent significant advancements in image upscaling, they're not without limitations. The complexities of perfectly recreating pixel data in every circumstance remain a challenge, suggesting a continued need for further refinement and innovation within the field.
In the evolving field of image enhancement, PhotoRoom and AVCLabs have emerged as prominent examples of AI-powered image upscaling tools. These tools leverage the power of machine learning models trained on vast datasets to intelligently upscale images, a significant leap beyond the traditional techniques of bilinear or bicubic interpolation. Instead of simply interpolating existing pixels, these AI-driven tools generate new pixels, carefully considering their context within the image, leading to a remarkable preservation of the original details and essence.
The advancements in AI algorithms translate to tangible benefits for the user, including remarkably fast processing speeds. Upscaling images that might have taken minutes with older methods can now be accomplished in mere seconds, thanks to optimizations that work on a wide range of devices. Moreover, these AI tools are not static. They continuously learn from new datasets, steadily improving their ability to upscale images accurately and realistically over time. This continuous learning differentiates them from older interpolation approaches, which have a fixed set of rules and are unable to adapt.
PhotoRoom, in particular, demonstrates intriguing possibilities by recognizing different artistic styles embedded within images. Its algorithms can enhance images while maintaining the style of the original artist, a feature that adds a valuable creative element to the upscaling process. In a similar vein, AVCLabs excels in managing the complex challenges of intricate images, adeptly preserving the fine details and textures often lost or blurred by older interpolation methods. Their ability to effectively synthesize textures, such as patterns found in fabrics or landscapes, further showcases their strength in replicating image complexity.
Another notable aspect is the inclusion of integrated noise reduction alongside upscaling. PhotoRoom and AVCLabs tackle the dual problem of resolution enhancement and unwanted artifacts or noise. This is a significant improvement over traditional upscaling methods which often exacerbate noise or produce undesirable artifacts in the upscaled image. Further, users are given considerable flexibility in tailoring the upscaling process, with features that allow adjustments to sharpness and details, a control level not usually found in simpler interpolation methods.
The performance of these AI tools extends beyond just static images. Their architectural design permits real-time upscaling during applications like video streaming or live broadcasting. This marks a substantial shift, as earlier image processing technologies struggled to achieve such real-time capabilities. And, both PhotoRoom and AVCLabs are designed to be used on a wide range of devices and platforms, enhancing their practicality and user-friendliness.
While these tools offer a powerful approach to image enhancement, the field continues to evolve. Understanding exactly how these networks achieve their impressive results remains a subject of ongoing research and development. Nevertheless, the benefits of AI-driven upscaling are clear. These tools are capable of creating higher quality images that retain their original character in a manner that was not previously possible. As a result, tools like PhotoRoom and AVCLabs demonstrate the significant potential of AI to shape the future of image enhancement, making previously impossible tasks now possible and routine.
AI-Powered Image Upscaling Enlarging Photos Without Quality Loss in 2024 - 16x Enlargement While Preserving Image Integrity
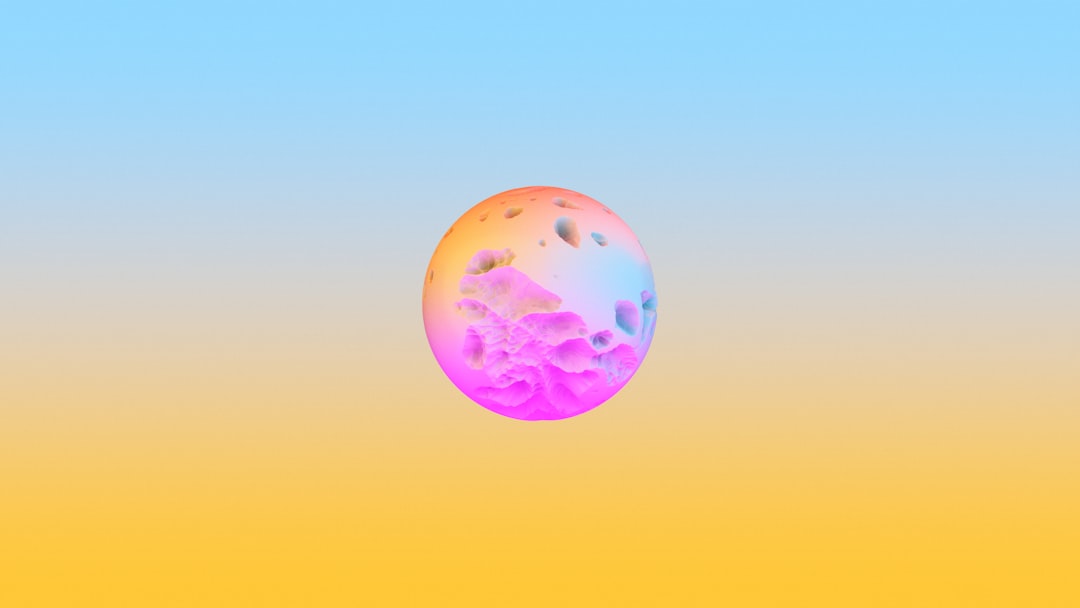
The ability to enlarge images 16 times their original size while maintaining image quality is now a reality thanks to the advancements in AI-powered image upscaling in 2024. Tools specifically designed for this purpose, like Deep Image AI and BigJpg, employ intricate AI algorithms to achieve this. These algorithms intelligently analyze image details and generate new pixels that seamlessly blend with the existing ones, significantly enhancing clarity and detail without introducing the common blurriness or pixelation that plagued traditional enlargement techniques. This signifies a notable shift in how images are processed, where the focus is not just on enlarging images, but on enhancing their overall quality during the process. The result is high-resolution images that retain the original characteristics of the image, a capability that was previously not possible.
While the results are truly impressive, creating perfectly recreated pixels in all situations continues to be a challenge. There's still a level of complexity and room for innovation to perfect the processes involved. However, for artists and those involved in professional work where high-quality image enlargements are crucial, AI image upscaling offers a solution for achieving significantly larger images without compromising on detail or fidelity. It represents a significant step forward in image processing technology.
Achieving a 16x enlargement while preserving image integrity presents a fascinating challenge in the field of image upscaling. The dynamics of pixel propagation become crucial at such a scale. Modern AI algorithms, unlike older approaches that simply average surrounding pixels, intelligently interpolate while considering the spatial relationships within the image. This leads to a remarkable enhancement in clarity and detail, particularly noticeable when comparing to the often blurry outcomes of older methods.
Furthermore, the ability of neural networks to learn and synthesize textures is a significant development. They can now reconstruct complex patterns, such as those found in fabric or natural environments, that weren't present in the original image. This 'learning-based' texture synthesis is key to achieving an authentic reproduction of even the finest details during the enlargement process.
Maintaining sharp edges and fine details during an enlargement is critical. AI upscaling tools demonstrate a remarkable ability to adaptively preserve details based on context. They seem to understand which areas require sharper focus and which can be smoothed, resulting in a more natural and aesthetically pleasing outcome. This is a substantial improvement over the past, where every area of an image would be treated equally, often leading to artificial-looking results.
Another challenge is handling images with non-uniform properties like diverse textures and color variations. Traditional techniques often struggle in such scenarios, leading to noticeable artifacts. AI-based upscaling methods have shown they can adapt to these complex cases, allowing for a more nuanced reconstruction of each pixel area during the enlargement. This highlights their inherent adaptability and ability to handle real-world image variety.
The rise of computational power allows for real-time upscaling in a growing number of applications. We're seeing this in video streaming and live broadcasts. This capability expands the possibilities for AI upscaling across diverse fields, including gaming, digital media, and broadcast production. This was simply not possible a few years ago, and it points to AI's growing influence on real-time multimedia.
In addition to enlarging images, these tools effectively handle noise reduction alongside the upscaling process. Older methods often worsen noise, leading to less desirable outcomes. The integration of noise reduction into the AI pipelines results in cleaner output with minimized distracting artifacts, further enhancing the integrity of the enlarged images.
It's also worth noting that even with the sophisticated calculations involved in 16x upscaling, these advancements have made the process much faster. The combination of specialized hardware acceleration and refined algorithms means that high-quality upscaling can be accomplished efficiently even on consumer-grade computers, challenging the perception that powerful hardware is essential for high-quality image manipulation.
Some AI tools also offer innovative artistic features like style transfer. This functionality allows users to improve image quality while preserving the artistic essence of the original work. For professionals in photography or graphic design, this opens up a range of new creative possibilities.
The ability of these AI models to continually learn and refine their upscaling capabilities is a significant advantage. The learning process is iterative and depends on expansive datasets. As these training sets grow in size and diversity, the algorithms gain a broader understanding of different image types and styles. This contrasts with static, traditional upscaling methods, which remain fixed and unable to adapt over time.
Furthermore, we now have quantitative quality metrics to assess image quality more rigorously. Researchers and engineers can now measure sharpness, detail retention, and overall image fidelity more accurately. These objective measures help guide the development and refinement of AI upscaling algorithms, pushing the boundaries of image quality in increasingly effective ways.
In summary, 16x image upscaling while maintaining image integrity is now possible due to the power of AI. It's an area of ongoing research and development, but it shows the remarkable potential of intelligent systems to transform image processing and enhance our ability to work with visual information.
AI-Powered Image Upscaling Enlarging Photos Without Quality Loss in 2024 - Online Platforms Accept Various Image Formats
Online platforms offering AI-powered image upscaling in 2024 have become more flexible in the types of images they accept. Common formats like JPEG, PNG, and BMP are widely supported, allowing users to easily upload a diverse range of photos for enhancement. The process typically involves uploading a lower-resolution image, selecting a desired upscaling level (often 2x, 4x, or even 16x), and letting the AI work its magic. This often results in significantly improved image clarity and detail without the blurry or pixelated results associated with older techniques. Some of the more prominent platforms, like PhotoRoom and AVCLabs, have integrated additional features such as noise reduction and the ability to enhance textures and detail, demonstrating the expanding capabilities of these tools. While it's impressive how well they work, perfectly recreating every pixel detail in complex images is still a work in progress. However, the ease with which users can upscale various types of images across diverse platforms represents a notable step forward for image processing in general.
Online platforms designed for AI image upscaling typically accept a range of common image formats, including JPEG, PNG, and BMP. JPEG, while prevalent, uses compression that can introduce quality degradation with repeated saving. In contrast, PNG, known for its lossless compression, might be a better choice for preserving finer detail, particularly in cases requiring high fidelity like graphic design or scientific visualizations. It's interesting that some platforms support less common formats like HDR for wider dynamic range, but not others, possibly due to niche usage and compatibility concerns. While GIF is mainly linked to simple animations, it also supports transparency, though its limited color palette prevents it from handling complex photographic imagery.
The SVG format is distinct in its vector-based nature, allowing for seamless scaling without loss of clarity, making it ideal for logos or other graphic elements requiring sharp detail across different sizes. WEBP, a newer format gaining traction, employs a combination of lossy and lossless compression to achieve both high quality and compact file sizes, which can be beneficial for faster website loading times. Professional users often utilize raw image formats like RAW or NEF, which hold all unprocessed image data. However, these formats aren't commonly supported by consumer-facing platforms, indicating a potential disconnect between professional needs and widely available services.
HEIF, a promising format capable of maintaining image quality while achieving file sizes half the size of JPEGs, highlights the continuous advancement in image compression. However, it hasn't achieved universal compatibility, which might limit its adoption for now. It's worth noting that while many platforms now utilize AI-powered upscaling, this processing can sometimes discard metadata embedded in the original file, potentially impacting future editing. Moreover, upload size limits imposed by platforms often influence the chosen format. Formats like JPEG and PNG, with their efficiency in compression, might enable users to upload larger files when compared to less compact formats, prompting some level of consideration in this aspect as well. The field of image formats continues to evolve as technology advances, and the compatibility and suitability of these formats across platforms and AI upscaling services requires attention and monitoring for researchers like ourselves.
AI-Powered Image Upscaling Enlarging Photos Without Quality Loss in 2024 - ImgGen AI Pushes Boundaries with 8K Upscaling
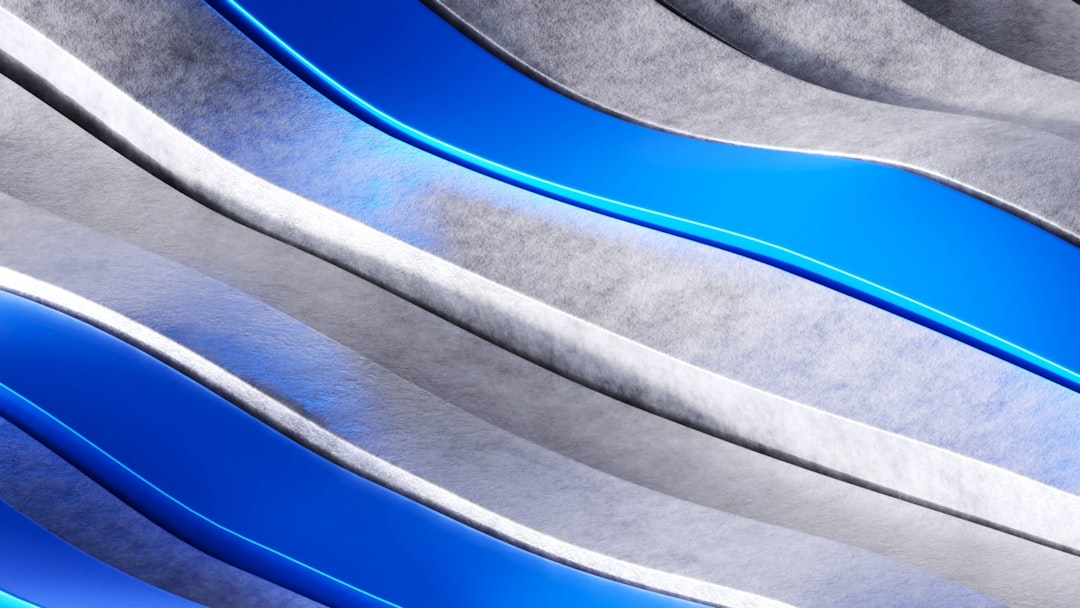
ImgGen AI is pushing the boundaries of image enhancement with its capacity to upscale images to 8K resolution. This free online tool leverages advanced AI to enlarge images without the typical trade-off in quality, resulting in exceptionally sharp and detailed output. The core of its effectiveness is the intelligent use of AI algorithms that are designed to analyze the complexities within an image and generate new pixels seamlessly. It's not just about simply making images bigger; ImgGen AI offers extra features, such as background removal and color adjustments, making it attractive for a broader range of users.
Traditional methods of upscaling, like interpolation, often result in blurry or pixelated images due to the limitations of their simplistic approach to generating new pixels. However, ImgGen AI, with its AI-powered algorithms, maintains fine details and textures throughout the upscaling process. As the demand for high-quality visuals expands across numerous applications, ImgGen AI is proving itself a significant tool for both individual users and professionals seeking high-quality enlarged images. While it may not be perfect in every scenario, its approach marks a positive step towards achieving truly lossless image upscaling.
ImgGen AI stands out in the current AI upscaling landscape by pushing the boundaries of resolution to 8K and beyond. This is quite impressive, showcasing the potential of AI to generate details and clarity that weren't possible with the older, more basic approaches. It's a testament to the ongoing progress, although achieving consistent quality across all kinds of images still has its challenges. The complexity of how pixels are put together in an image presents hurdles that AI developers are continually tackling.
The way ImgGen's AI handles pixels is different from the older methods. Rather than simply averaging neighboring pixels, it dives deeper, looking at the spatial relationships within an image to produce finer detail and sharper edges when enlarging an image. This is a significant leap forward.
This ability to adapt and learn is an important part of ImgGen AI. The models that drive it are constantly being improved by training them on huge amounts of data. This means the more data these models ingest, the better they get at upscaling images and generating high-quality results. It's a constant process of refinement that older methods simply couldn't achieve.
One of the notable aspects of ImgGen is its capacity to reconstruct textures. It can intelligently create intricate patterns and textures that weren't even present in the original image. This kind of learning-based texture synthesis is a unique advantage compared to older methods.
Another noteworthy aspect is the rise of real-time processing. This advancement in computational power means that users can see upscaled images instantaneously in a growing number of applications. Think about watching a live stream or playing a video game—the visuals can now be enhanced on the fly. This sort of speed was simply out of reach before, and it's a clear indication of the growing importance of AI in visual media.
ImgGen's AI algorithms not only improve resolution but also incorporate noise reduction into the upscaling process. This is a welcome change as older methods often made noise even worse during the enlargement process. The integration of noise reduction leads to clearer and cleaner images with minimal distracting artifacts.
The AI's ability to handle images with varying textures and color variations is a strong point. Older methods struggled with images that had diverse elements, but ImgGen's AI can adapt, providing more nuanced and intelligent reconstructions. This adaptability highlights a core strength of these new techniques.
Similarly, the AI can analyze an image and determine which areas need sharper focus versus areas that can benefit from some smoothing. This contextual understanding results in upscaled images that look more natural and realistic. It's a marked improvement over earlier methods that often made images appear artificial.
The field of AI upscaling is moving towards quantifiable measures of image quality. Things like sharpness and detail retention are being carefully tracked. This lets developers assess the quality of the output and use those measurements to further refine the algorithms. It's a powerful tool for pushing the boundaries of AI image enhancement.
Finally, the input format flexibility is noteworthy. ImgGen can handle a variety of image types from JPEG and PNG to even more specialized ones like HDR and WEBP. This flexibility is helpful, although it also shows that developers constantly need to think about how these different formats relate to the upscaling process. It's an ongoing balancing act that impacts the overall performance and applicability of these techniques.
Overall, ImgGen AI is demonstrating exciting capabilities in the world of image upscaling. While challenges remain, particularly in terms of fully replicating every intricate pixel detail, the improvements are evident. These developments indicate that the future of image processing will be driven by these intelligent systems, allowing us to interact with visual information in increasingly sophisticated ways.
Transform your ideas into professional white papers and business plans in minutes (Get started for free)
More Posts from specswriter.com: