Transform your ideas into professional white papers and business plans in minutes (Get started for free)
Efficient MP4 Compression A Deep Dive into File Size Reduction Techniques for 2024
Efficient MP4 Compression A Deep Dive into File Size Reduction Techniques for 2024 - Video Codec Advancements for MP4 in 2024

The evolution of video codecs continues in 2024, with newer options like VVC, LCEVC, and EVC aiming to push the boundaries of compression efficiency. These codecs are designed to tackle the challenge of handling increasingly higher resolution videos while maintaining quality. However, the established H.264 codec, despite being relatively old, remains the dominant choice due to its wide compatibility and solid performance. H.265/HEVC, with its significant compression improvements compared to H.264, is becoming more relevant for situations requiring maximum storage efficiency. This ongoing evolution highlights the persistent tension between compressing video data for efficient storage and delivery, and preserving the original quality of the footage. While achieving high compression is important for modern digital media, it is critical to avoid excessive compression which can significantly impact the visual quality of videos.
The evolution of video codecs for MP4 in 2024 shows a strong push towards AI-driven optimization. We see encoding techniques that can dynamically adjust compression based on video content, potentially leading to file size reductions of up to 50% while preserving visual fidelity. Codecs like AV1 and VVC are being integrated into the MP4 format, offering more advanced prediction models that leverage redundancies within videos more efficiently. This translates into lower bitrates for the same or even higher resolution output.
Interestingly, newer codecs within MP4 are now capable of handling 12-bit color and HDR. This opens up a world of potential for content creators who desire greater color accuracy and detail in their videos. The shift from codecs like H.264 to H.265, and then newer alternatives, reflects a clear trend: significant reduction in average bitrates – around 30% – while achieving a similar level of quality, particularly noticeable in scenes with intricate textures.
The business landscape is also impacted. Cloud-based environments now incorporate features for auto-scaling video resolutions based on viewer bandwidth. This could revolutionize streaming services, especially during periods of high usage. Moreover, hardware advancements have improved encoding and decoding speeds, making it possible for even consumer devices to smoothly handle high-efficiency codecs like H.266 without excessive processing strain.
Another notable advancement is the development of vendor-agnostic codecs. This is a boon for content creators, allowing them to generate MP4 files optimized for performance across different platforms without encountering compatibility issues. This simplification of workflows is valuable. Efforts are underway to improve error correction, particularly for streaming over unreliable connections. This is crucial for maintaining a quality experience during live events or remote video viewing.
We are also witnessing the implementation of adaptive streaming within codec design. This feature involves analyzing how viewers interact with videos, subsequently optimizing the content delivery and quality based on individual preferences. Lastly, with the continuing rise of mobile video consumption, codecs are being optimized for energy efficiency on portable devices. This leads to longer playback times at high quality, an increasingly important factor for users. It will be interesting to see how these advancements shape the future of MP4 and the overall video streaming experience.
Efficient MP4 Compression A Deep Dive into File Size Reduction Techniques for 2024 - Adaptive Bitrate Streaming Techniques for File Size Optimization
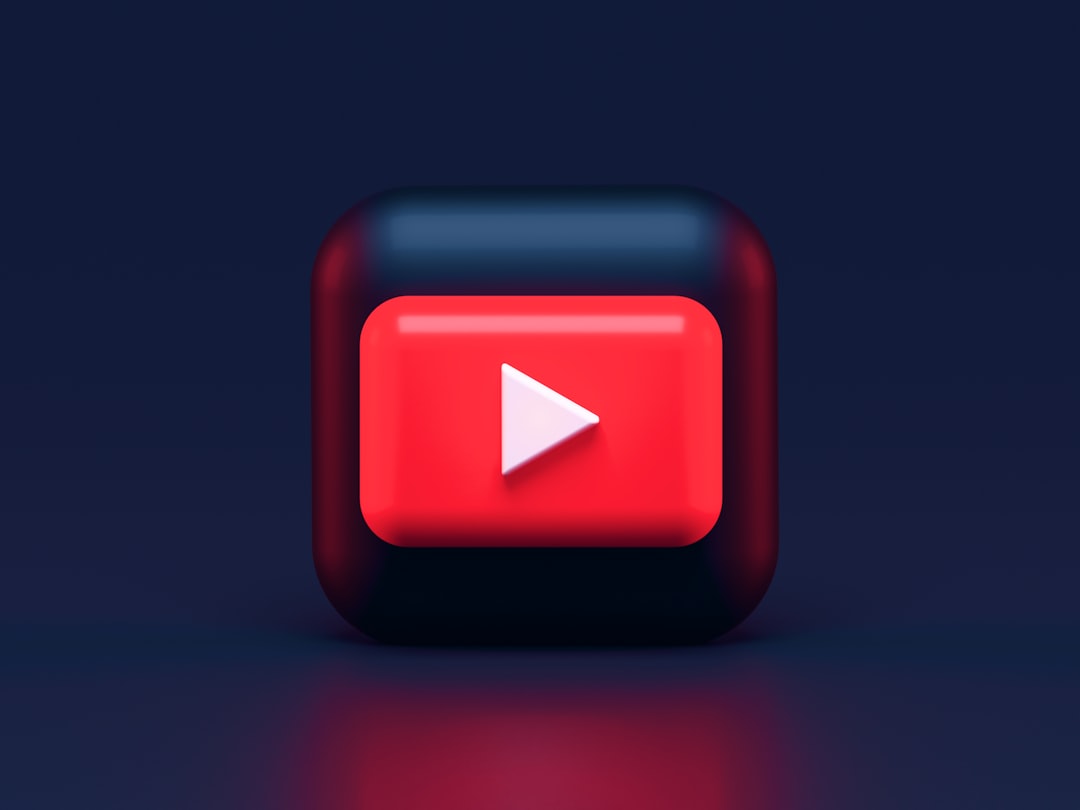
Adaptive Bitrate Streaming (ABR) has become a key method for optimizing MP4 file sizes and improving the overall streaming experience in 2024. Essentially, ABR intelligently adjusts the quality of the video stream in real time, based on factors like the viewer's internet connection speed and device capabilities. This dynamic approach ensures smooth playback, even with fluctuating network conditions, by delivering the highest quality video that the connection can support.
Further, ABR's capabilities extend beyond simply handling network changes. It can analyze the content of the video itself, classifying scenes as more or less complex, allowing for further fine-tuning of the stream quality. This ongoing effort to incorporate more advanced algorithms into the streaming process highlights a trend: a continuous drive to deliver high-quality viewing experiences while also managing file size efficiently. As viewer expectations for high quality streaming grow, employing techniques like ABR becomes increasingly important in today's competitive media environment.
Adaptive bitrate streaming (ABR) is a technique that dynamically adjusts the quality of a video stream based on factors like a viewer's internet connection, device capabilities, and even the complexity of the scene being shown. This means the video quality can change seamlessly during playback to ensure a smooth experience, without interruptions caused by buffering or slowdowns.
Essentially, ABR works by monitoring the network conditions and viewer's device and intelligently switching between different versions of the video encoded at varying bitrates. This clever adaptation can lead to significant reductions in data usage, potentially up to 50%, which benefits both the viewer and the streaming service by optimizing server resources and reducing bandwidth costs during peak times. ABR can be likened to how telecommunication networks manage traffic by using statistical multiplexing to share the available bandwidth across multiple streams.
Interestingly, research has shown that viewers can generally tolerate a reduction in video quality of about 30% without noticing a substantial difference. This tolerance level is crucial for ABR, as it enables the system to maintain a higher-than-expected quality for a longer duration compared to traditional fixed-bitrate streaming.
Further, ABR offers advantages in multi-device environments. Since different devices have varied screen sizes and resolutions, ABR adapts on the fly, delivering the best quality version for each device without requiring multiple, separate video files, which saves storage space and stream-loading times. The downside is that encoding a single file with multiple resolutions and bitrates can be computationally intensive.
However, there are drawbacks. ABR can sometimes lead to 'buffer bloat', where the buffering system doesn't adjust fast enough when network conditions change, causing delays and disruptions in the video playback. Furthermore, in live streaming, the process of handling and switching between different resolutions can introduce latency, which may be an issue for applications that require real-time interactions like gaming or live event broadcasts.
Despite these issues, the development of standards like CMAF aims to address some of these challenges. CMAF aims to standardize ABR encoding and delivery across various platforms, reducing the complexity for developers. Moreover, researchers are exploring the use of machine learning to further refine ABR, by using historical data about viewing patterns to predict individual preferences and tailor the streaming experience. For instance, if a viewer tends to prefer higher quality streams, the system could anticipate this and prioritize higher-quality versions when available.
This continuing evolution of ABR suggests that it will play an increasingly important role in the future of video delivery, particularly as the demand for high-quality streaming experiences continues to grow. It remains to be seen how these advancements will continue to improve the quality of service and user experience for those who watch streamed content.
Efficient MP4 Compression A Deep Dive into File Size Reduction Techniques for 2024 - AI-Powered Compression Algorithms and Their Impact

AI-powered compression algorithms are transforming video compression, offering the potential to significantly improve efficiency and potentially enhance quality while reducing file size. These algorithms use sophisticated machine learning techniques to delve deeper into video data than traditional methods, enabling the discovery of previously unnoticed redundancies. This allows for better compression ratios, resulting in smaller files without compromising, or even potentially enhancing, visual quality. However, ongoing research highlights a growing need for AI-driven algorithms that optimize both performance and computational efficiency, especially when dealing with resource limitations like those found on mobile devices. As AI integration within video compression systems expands, its impact on how digital media is managed and transmitted becomes increasingly evident. The future of video streaming experiences could be fundamentally altered as a result, and it's crucial to anticipate and manage the consequences of this evolving technology.
Conventional video compression methods largely relied on lossy algorithms, sacrificing some video quality to decrease file sizes. This approach, while effective, faced limitations in achieving truly optimal compression. However, AI-powered compression algorithms are revolutionizing the way video is compressed, offering a path towards significantly improved efficiency. These algorithms analyze video data in a far more nuanced way, allowing them to pinpoint and eliminate redundancy more precisely than traditional methods. This ability to identify what parts of the video are essential and which can be discarded with minimal quality loss can result in impressive file size reductions.
The emergence of deep learning has been particularly impactful. Recent research suggests that AI-designed video compression techniques are achieving, or even exceeding, the performance of manually-crafted algorithms. They achieve greater compression ratios by detecting intricate redundancies that might be missed by conventional compression methods. This leads to enhanced streaming efficiency, especially crucial for applications like large media repositories or internet streaming where minimizing data transfer is important.
Deep Neural Networks (DNNs) have become a core component of many modern video compression systems. Researchers are actively using them to optimize individual parts of the compression pipeline, as well as the overall process. Though promising, some research also emphasizes the need for more power and computationally-efficient AI-based solutions if they are to effectively replace existing codecs. One intriguing avenue is content-adaptive super-resolution techniques, which show potential for optimizing both quality and file sizes.
The development of intelligent compression methods through neural networks is still underway. While these techniques hold a lot of promise, they are still actively being developed. However, all video compression techniques ultimately build on core concepts like removing redundant data using transforms and applying entropy coding, principles that remain essential for achieving the best video size and quality trade-offs. Even with AI, optimizing the compression process comes down to using these fundamental approaches in more sophisticated ways.
The shift to AI compression is also impacting the industry. Companies that effectively integrate AI compression into their systems often see a tangible impact on user engagement due to the improved streaming experience. The ability to seamlessly and dynamically adjust quality based on network conditions and viewer behavior contributes to this positive outcome. But, a significant hurdle is the increased computational load these algorithms place on systems. It remains to be seen whether this will create a gap between larger organizations with substantial resources and smaller entities that may struggle to fully benefit from these advancements.
Efficient MP4 Compression A Deep Dive into File Size Reduction Techniques for 2024 - Hardware Acceleration in MP4 Compression
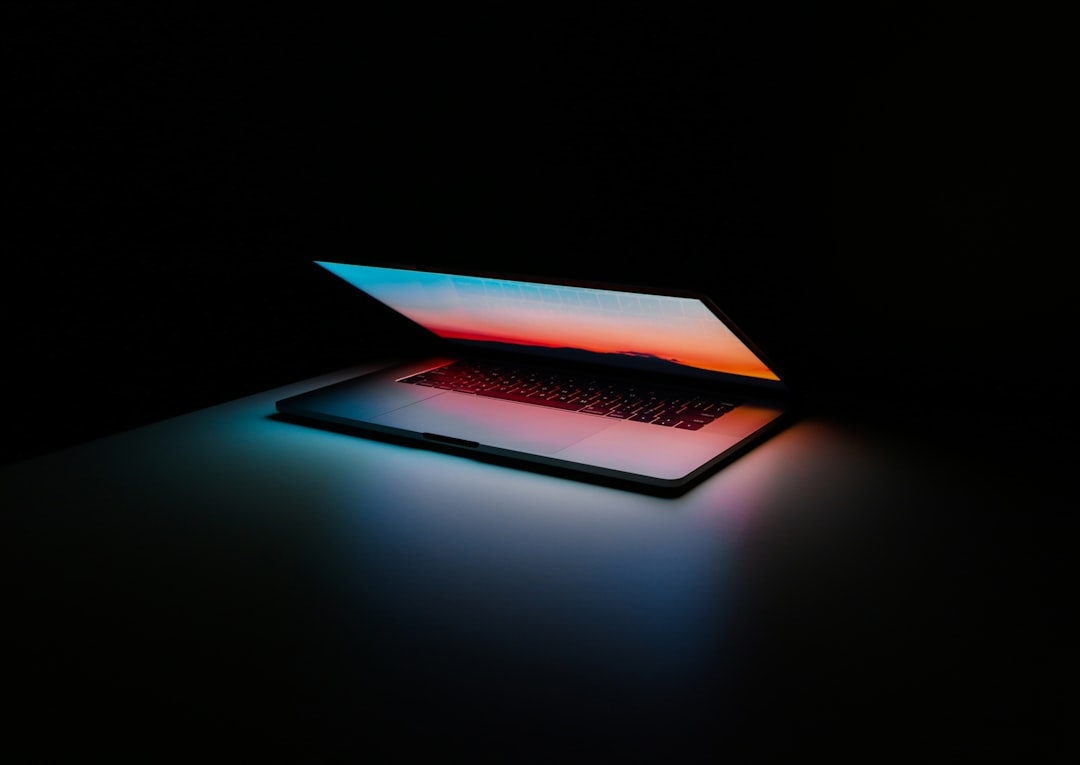
Hardware acceleration has become a critical element in MP4 compression, particularly as we deal with larger and larger video files and more demanding workflows. Using specialized processing units like GPUs and dedicated video encoders, hardware acceleration can dramatically speed up the encoding process. This makes it a valuable asset for anyone working with video, especially when time is a constraint.
However, it's not without limitations. Compatibility issues between different hardware and software can be a challenge. Also, the initial investment for specialized hardware can be a hurdle. Further complicating matters, the evolving landscape of compression algorithms, notably AI-powered codecs like AV1 and VVC, necessitates adaptable hardware capable of managing their increased computational loads.
Ultimately, the integration of hardware acceleration represents a double-edged sword in the search for the best MP4 compression. While it delivers considerable performance gains, it also brings new issues and requires careful consideration as video technologies continue to advance. It's a crucial aspect of modern video workflows, yet its efficacy hinges on its capacity to keep pace with the ever-increasing complexity of compression algorithms.
Hardware acceleration is becoming increasingly important in the realm of MP4 compression, primarily due to its ability to significantly boost performance and efficiency when handling large video files. This acceleration is primarily achieved by using specialized hardware like dedicated processors or GPUs to execute the computationally intensive tasks involved in MP4 encoding and decoding. We've seen that it can lead to a speed increase of up to 200% compared to software-based methods, which is quite remarkable, making it a very attractive option in numerous scenarios. However, it's also worth noting that this boost in performance often comes hand-in-hand with higher energy consumption, though hardware developers are constantly looking to optimize energy efficiency.
Another advantage of hardware acceleration is the possibility of handling high-resolution video, like 4K or even 8K, in real-time. This has huge implications for applications such as live streaming where encoding needs to occur rapidly. There is a notion that when we accelerate encoding, there may be a trade-off in quality, but with newer codecs and optimized hardware designs this is less of an issue. It seems the quality of video is preserved well and there are less compression artifacts during encoding. It is noteworthy that many modern GPUs from different companies now support hardware-accelerated MP4 encoding, which has made it much easier for software developers to use these features across a wide range of devices and operating systems. This ease of implementation across platforms represents a clear benefit for video developers who desire a consistent user experience.
There are, however, challenges associated with this method. One issue is latency, specifically in scenarios where resolutions are being dynamically changed, which can be a challenge for real-time applications where a consistent frame rate is critical. Interestingly, hardware acceleration is also increasingly being integrated with AI algorithms that optimize the encoding and decoding process. This is a potentially transformative development since it allows for more dynamic adjustments to the quality and file size of video on-the-fly.
Despite all the benefits, accessibility remains a challenge. Specialized hardware is needed to fully reap the rewards of hardware acceleration and this requirement can act as a barrier for smaller content creators who might not have access to such resources. It would be interesting to see if hardware acceleration might start to appear on mobile devices more often, since the majority of online video consumption occurs on smartphones and tablets. We can predict that as video resolutions continue to increase, hardware acceleration will play a more crucial role in supporting the newer compression codecs, especially with higher refresh rates and dynamic ranges being utilized more often. Finally, one aspect that needs to be considered in the design phase is the fact that initiating hardware acceleration can sometimes add a noticeable startup delay, something that engineers need to consider if they want to ensure a rapid and smooth user experience. It is evident that these developments continue to shape video encoding practices, and it is anticipated that the hardware and software needed to support this advancement will become more integrated and accessible in the near future.
Efficient MP4 Compression A Deep Dive into File Size Reduction Techniques for 2024 - Cloud-Based Compression Services and Their Efficiency
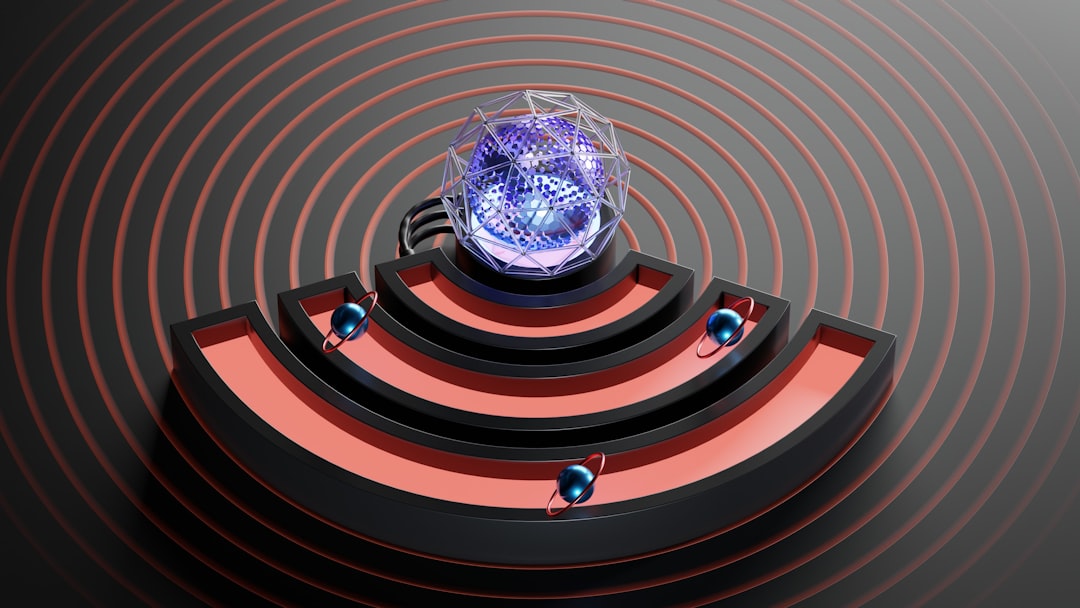
Cloud-based compression services are increasingly relying on sophisticated deep learning methods to achieve more efficient video compression compared to older, human-designed algorithms. Users can select between lossy and lossless compression, allowing them to find the balance between reduced file size and preserving the original quality of their video. The latest deep learning advancements have led to impressive reductions in bandwidth requirements, with some methods showing up to 46% less data needed to represent a video. While this is promising, it often comes with the trade-off of increased time to encode and decode.
Beyond standard video compression, deep learning is also being applied in specialized areas like compressing point cloud videos, which are used to represent 3D scenes. These AI-based methods show potential for significantly improved compression density, even surpassing older methods by a factor of nearly 3. However, these new methods often need substantial processing power and memory, which can be a drawback for those on older or less powerful hardware.
Artificial neural networks are finding applications in various aspects of compression, from better data representation to reducing common video artifacts. While these innovations are significant, they come with tradeoffs. For instance, optimizing video quality and file size using these methods requires careful balancing, especially since their implementation can involve a considerable increase in computing needs, something that must be considered in real-world scenarios. As deep learning and cloud services mature, we may see a major shift in the way we deliver and consume video, but it's crucial to understand and address the limitations of this approach in practical use.
Cloud-based compression services are becoming increasingly popular, leveraging the power of multiple servers and dynamic resource allocation. This approach, while offering potential benefits, also comes with its own set of considerations. One promising aspect is the ability to distribute the workload across multiple servers, which can speed up the compression process considerably. This is especially advantageous when dealing with massive video files that would otherwise strain a single machine. Cloud providers often manage resources in a way that adapts to the current workload, adjusting server usage based on demand. This is great for efficiency, as it reduces the chances of wasting resources during periods of low usage. However, it also highlights a potential issue: latency can be an unpredictable factor. While processing locally usually results in consistent latency, relying on cloud servers adds an element of unpredictability, largely due to factors like server loads and the general state of the network. This can be particularly problematic for live streaming, where delays can cause significant issues.
In the context of optimizing video quality, cloud services often employ machine learning techniques to evaluate the compression process using metrics like PSNR and SSIM. These metrics quantify how much of the original video quality is preserved during the compression, essentially giving us a way to fine-tune the compression settings. Furthermore, the use of APIs to access these cloud services is a common feature, allowing for easier integration with existing video editing tools and automation pipelines. This makes the use of cloud-based services simpler, especially when tasks need to be repeated or if automated integration is necessary.
Cloud platforms also have the capability to analyze video metadata, which includes information about the video like frame rate, resolution, and scene complexity. By having access to this information, the service can dynamically apply the best compression settings for each video. This is smart, but the underlying algorithms and implementation can be quite complex. Further, services use advanced load balancing techniques, not just to ensure servers aren't overloaded but to also account for the location of the users. In essence, if a user is physically closer to a particular server, the service attempts to route the compression and download tasks through that server. This is another clever approach to improve the speed of accessing video content.
Interestingly, cloud-based services often offer a wide range of compatibility with video formats, going back to older standards alongside newer codecs. This is a very practical feature, as it makes the migration to more modern formats easier. While advantageous, this broad compatibility comes at a potential cost in terms of scalability. While a major advantage of cloud-based platforms is their flexibility in scaling, the ability to handle large spikes in user demand and data transfer depends on the capacity of their network infrastructure. When scaling video compression projects, keeping track of bandwidth needs is quite crucial. The final area to consider is security. Using cloud-based services requires trusting the platform with the integrity of your video data. Although most services have robust encryption policies, security incidents with cloud storage can occur, emphasizing the need for responsible data management when using these platforms for sensitive content.
In summary, while cloud-based compression offers numerous advantages like speed, efficiency, and accessibility through APIs, there are several considerations to keep in mind, including potential latency variations, bandwidth scalability, and security concerns. The evolving nature of these services means that these considerations will likely continue to be refined and potentially redefined as these platforms advance.
Efficient MP4 Compression A Deep Dive into File Size Reduction Techniques for 2024 - Balancing Quality and File Size The Latest Encoding Parameters
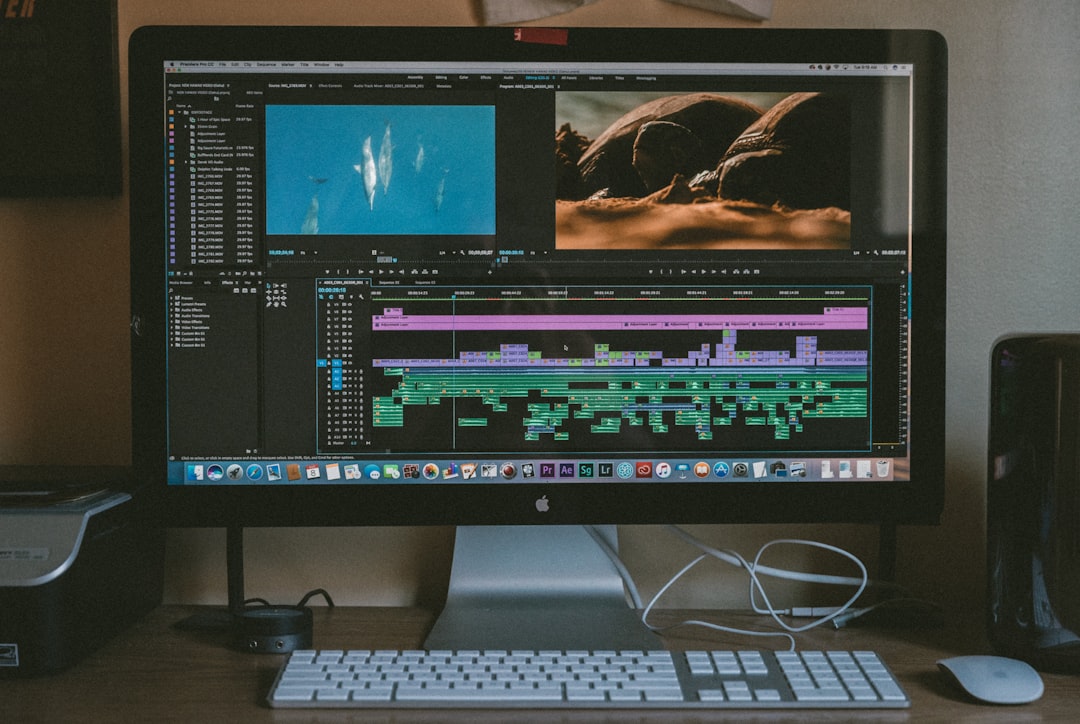
The goal of efficient video compression is to strike a balance between preserving visual quality and minimizing file size. Modern encoding techniques, utilizing parameters like Constant Rate Factor (CRF), encoding presets, and pixel formats, give users more control over this process. Tools like Smart Encoders, driven by AI, are emerging, which automatically choose encoding settings to optimize for specific videos, streamlining the task of finding that sweet spot between quality and file size. However, we should remain mindful of the inherent limitations of lossy compression, where some quality is inevitably sacrificed to reduce file sizes. In 2024, understanding and using these new encoding parameters becomes critical for anyone looking to create and deliver high-quality video content without unnecessary file bloat. This ability to fine-tune the compression process is a key step in delivering an optimal user experience.
Fine-tuning video encoding parameters like CRF, presets, and pixel formats within tools like FFmpeg is crucial for achieving a balance between high video quality and manageable file sizes while also considering encoding speed. It's a bit of an art to get it right. For example, a CRF value of 18 is often considered a good compromise for achieving "visually lossless" quality, but the standard default value in FFmpeg is 23, illustrating that striking this balance involves ongoing experimentation.
While tools like Smart Encoder can automate parameter selection, it's helpful to understand how these settings influence compression. MP4 continues to be a popular and versatile format due to its ability to find a reasonable balance between compression and quality. We've seen that the MP4 format has evolved to incorporate newer codecs like AV1 and VVC. This demonstrates the ongoing search for better compression techniques. These codecs, in conjunction with AI algorithms, can adapt the compression levels based on the content, enabling further optimization across a variety of devices and platforms.
Compression itself is a trade-off. Lossy compression, as we discussed earlier, reduces file size considerably, but inevitably leads to some degradation of quality, whereas lossless compression preserves every bit of the original video data, resulting in larger file sizes. This distinction becomes particularly important when you deal with newer features in MP4 like HDR or 12-bit color. As you'd expect, features like higher resolution and higher color fidelity translate into larger files. There is a constant struggle to balance increased quality with acceptable file sizes, and that involves sophisticated mathematical algorithms.
While many techniques offer significant file size reductions, sometimes up to 50% using AI-driven compression, it's essential to avoid compression levels that make the compression artifacts too noticeable. Variable Bitrate (VBR) encoding offers a way to enhance quality without overly increasing file size by adjusting the encoding based on content. There's an interesting correlation between human visual perception and how these compression algorithms are crafted. Some newer methods don't just focus on traditional compression metrics; they use techniques to make the most important parts of the video look as good as possible, effectively prioritizing parts that viewers are most likely to notice. This is a clever approach.
We also know that the demands for real-time streaming and low-latency viewing experiences push us to use adaptive bitrate streaming. However, the need for rapid switching between different bitrates can create challenges, sometimes leading to unwanted issues like higher processing requirements or buffering concerns. Interestingly, the transition to newer codecs often creates issues with older devices and platforms, so maintaining compatibility becomes a factor in how effectively the newest features can be implemented. This is a common problem. Luckily, the use of vendor-agnostic codecs can help make cross-platform compatibility simpler. This is a positive development.
Modern streaming demands also highlight the importance of resilient encoding. By designing encoding with more advanced error correction techniques, engineers can create video that is less susceptible to distortion from unreliable connections. These are especially vital when live streaming, as they often utilize real-time feedback mechanisms to ensure a smooth viewing experience even during challenges like network interruptions.
In the end, we see a constant push to optimize video compression. The search for the sweet spot between file size and visual quality continues to drive innovation. While the latest techniques using AI can produce considerable improvements, it's crucial to be aware of their limitations and to choose the encoding parameters that match your needs, while also understanding that a constantly evolving landscape of video standards and codecs will likely always be part of this discussion.
Transform your ideas into professional white papers and business plans in minutes (Get started for free)
More Posts from specswriter.com: