How H265 Codec Reduces Video File Size While Maintaining Quality in 2024
How H265 Codec Reduces Video File Size While Maintaining Quality in 2024 - 60 Percent Storage Reduction Through Improved Block Partitioning and Motion Prediction
H.265's ability to shrink video file sizes by up to 60% is largely due to advancements in how it breaks down and predicts video content. These improvements focus on how video frames are divided into blocks (partitioning) and how the codec anticipates changes between frames (motion prediction). Techniques like quadtree structures allow for more flexible and adaptive block sizes, enabling the codec to tailor its encoding approach to the specifics of each scene.
Furthermore, refining the methods used to predict motion between frames allows the codec to more efficiently represent changes, thus reducing the amount of data needed to store the video. This sophisticated inter-frame prediction combined with flexible partitioning enables H.265 to handle intricate video sequences effectively without sacrificing visual quality. The impact is a considerable reduction in storage requirements, addressing the ongoing challenge of managing and storing the ever-increasing volume of video data generated and consumed in our digital age. While these advancements represent a significant step forward, there's always potential for further optimization and refinement as video compression technology continues to evolve.
The way video frames are divided into smaller blocks, known as block partitioning, has been significantly refined in newer codecs like H.265. This refinement allows for more precise encoding of the visual information within each frame, particularly details in areas of complexity, without sacrificing image quality. This improved partitioning directly leads to less redundancy in the encoded data.
Coupled with this improved partitioning is a more accurate prediction of how parts of a video sequence move from one frame to the next. This motion prediction effectively leverages the inherent similarities between consecutive frames, a concept known as temporal coherence. By accurately predicting the movement, we can minimize the amount of new data that needs to be encoded for each frame, thereby contributing significantly to compression.
The combined effect of the enhanced block partitioning and refined motion prediction is notable. Some estimations suggest a storage reduction of up to 60% compared to older codecs such as H.264, especially for content with fast-paced motion. This substantial reduction is particularly impactful as it helps mitigate the growing challenge of managing and storing large volumes of video data.
Modern codecs like H.265 are capable of adjusting the size and shape of these blocks depending on the characteristics of the video content. Simpler sections can now be compressed even more efficiently than was possible before. This dynamic adjustment is akin to using a fine-tuned brush for painting a picture, applying the right level of detail only where it is needed.
Furthermore, the more intelligent allocation of bits based on the complexity of the video allows for the preservation of visual quality, especially when compared to older codecs working under the same bitrate constraints. This ensures that visual fidelity is maintained, even in scenes with a lot of action or changes.
One specific technique that enhances motion prediction in H.265 is Adaptive Motion Vector Prediction (AMVP). AMVP focuses on minimizing errors in motion prediction, leading to a reduction in unnecessary data being transmitted. This reduction is important for both storage and bandwidth efficiency.
Interestingly, the improved block partitioning mechanism in H.265 is also better at accommodating a range of video resolutions, from standard 1080p to high-resolution formats like 8K. This signifies the scalability of the codec, adapting to the evolving landscape of video content.
Moreover, H.265 uniquely allows for various block partitioning strategies for encoding the same video sequence. This means that the encoder can choose the most efficient approach depending on the nature of the content, leading to optimized compression tailored to the video.
The addition of new prediction modes in H.265 further enhances the codec's capabilities. These modes provide more flexibility in leveraging both spatial and temporal redundancies within video sequences, a refinement over its predecessors.
Finally, H.265 introduces a structural unit called a Coding Tree Unit (CTU). This approach structures frame encoding in larger cubic regions, offering more intuitive and efficient compression methods tailored for modern video content. These refinements and advancements are a testament to the continuous evolution of video compression technology.
How H265 Codec Reduces Video File Size While Maintaining Quality in 2024 - Advanced Frame Compression Using Spatial and Temporal Correlations
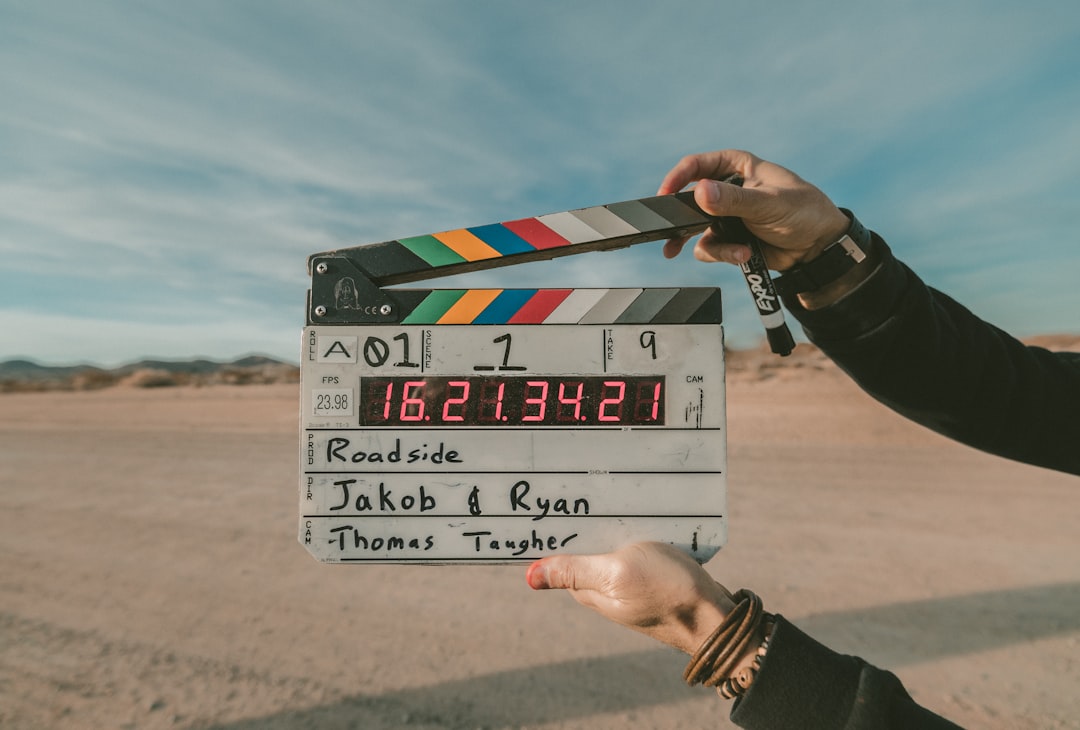
H.265's effectiveness in compressing video files while maintaining quality is, in 2024, increasingly linked to its ability to exploit relationships within and between video frames. This means leveraging what's similar within a single frame (spatial correlation) and the similarities between consecutive frames (temporal correlation). By recognizing these patterns and redundancies, H.265 can efficiently encode video information.
The development of techniques like using prior knowledge about temporal patterns and unified compression approaches that consider both spatial and temporal details has greatly streamlined the video encoding process. These methods are especially useful for real-time applications, ensuring video quality isn't sacrificed for speed.
More recently, machine learning-based methods and adaptable resolution encoding have become key areas of focus. These innovations are critical for handling the expanding volume of video data we encounter. The potential for even more efficient video compression and quality retention remains a central point of development, driving efforts to refine and further optimize these processes. We are in the midst of ongoing progress in this area, with implications for how we manage and consume video content.
H.265, also known as HEVC, achieves impressive compression by cleverly exploiting relationships between pixels, both within a single frame and across multiple frames. This is where the power of spatial and temporal correlations comes into play. For example, examining how similar nearby pixels are within a frame (spatial correlation) allows the codec to more efficiently encode regions that don't change much, like a still background.
The advancements in video compression extend beyond just traditional methods. Modern image codecs are pushing the boundaries of compression ratios previously considered achievable, suggesting there's still significant room for progress. Notably, there's been a surge in the use of machine learning approaches that are particularly adept at integrating both spatial and temporal information. This is a fascinating development, as it represents a shift from the more rigid, rule-based methods used in earlier codecs.
One of the key innovations in H.265 is the clever use of "temporal priors" within the entropy model. This essentially allows the codec to anticipate what information is likely to appear in future frames based on the information it has already encoded. This is particularly useful for video sequences where content changes gradually, as it reduces the redundancy of encoding similar information repeatedly.
The I2VC framework represents an interesting approach to unified spatiotemporal compression. The clever design allows it to adapt feature compression rates based on the perceived importance of different parts of a video scene. This concept of dynamically adjusting the encoding effort based on content importance is a notable aspect of the evolution of video compression.
Furthermore, techniques like ViSTRA showcase the dynamic adaptability of modern codecs. By adjusting both the spatial resolution (the pixel count) and the temporal resolution (the frame rate) during the encoding process, the codec can dynamically optimize its operations, potentially further refining the balance between compression and quality.
A similar goal is pursued by the Spatiotemporal Information Balance (STIB) module. It aims to strike an optimal balance between efficiently encoding both the spatial details within each frame and the temporal changes across frames. This is essential, as a codec needs to represent both aspects well to preserve the overall quality of the video.
Interestingly, learned video compression approaches are adapting traditional coding techniques, such as motion residual coding, through neural networks. This can potentially enhance their effectiveness and resilience to various types of video content. The use of convolutional LSTM architectures in particular highlights how machine learning is impacting video compression by providing a more adaptive means of handling the complex interrelationship between space and time in video data. This approach has shown promise in low-delay scenarios, suggesting that it might find application in real-time video processing.
The application of machine learning in the field of video compression is ushering in a new era of possibilities. As these techniques continue to evolve, we can expect innovations in areas like real-time super-resolution and frame interpolation, which will refine video experiences for viewers. While there are many challenges remaining, the ongoing research promises to reshape how video is processed and enjoyed in the years to come.
How H265 Codec Reduces Video File Size While Maintaining Quality in 2024 - Bandwidth Optimization With 4K UHD Content at Half The Size
The growing demand for 4K UHD content has spurred a need for efficient bandwidth management. H.265, a newer video codec, has emerged as a key solution, achieving impressive results by halving the file size of 4K UHD videos compared to older formats like H.264, while retaining a high degree of visual quality. This reduction is attributed to enhancements in the way H.265 handles video data, including improved methods for breaking down video frames into smaller segments and predicting how parts of a scene move from one frame to the next. This efficiency is particularly notable in streaming scenarios, where recommended bandwidth for 4K HDR content using H.265 drops to roughly 15 Mbps, compared to 32 Mbps with H.264. This makes high-quality video experiences more accessible, even when internet speeds are limited. However, with the ever-increasing resolution and complexity of video content, the pursuit of balancing file size optimization and visual fidelity remains a challenge. Nevertheless, H.265 signifies a significant step in making bandwidth-friendly, high-quality video a reality.
H.265's efficiency in compressing video data is particularly evident with 4K UHD content. It can achieve roughly half the file size compared to H.264, while maintaining a similar level of image quality. This breakthrough in compression ratios significantly reduces the strain on storage and bandwidth, making 4K content more accessible.
The way H.265 handles the partitioning of frames is key to its efficiency. It doesn't just divide frames into fixed-size blocks but uses a dynamic approach where block sizes can vary within a single frame. This adaptive strategy means that simpler parts of a scene, like a mostly static background, can be compressed more aggressively without sacrificing detail in complex areas.
Moreover, H.265's motion vector prediction has seen significant improvements. Adaptive techniques allow for more accurate motion tracking, leading to better visual quality and reduced processing for scenes with many moving elements. This is especially useful for action-heavy content where traditional methods can struggle to keep up.
H.265 introduces a range of prediction modes that it can utilize during the compression process. These modes cleverly exploit redundancies both within a single frame (spatial) and between consecutive frames (temporal). This ability to choose the most appropriate mode based on the content leads to more effective compression and better file size reduction.
The structure of H.265, employing Coding Tree Units (CTUs), offers a more efficient way to process the information in a frame. These CTUs are larger cubic regions, as opposed to just rectangular blocks, allowing the codec to work in a more intuitive and streamlined manner, especially with high-resolution videos like 4K.
It's interesting to note that, because of these advancements, high-quality video can be streamed in real-time with reduced latency. This is significant for interactive applications like video conferencing and online gaming, where quick feedback is paramount.
Furthermore, the codec adapts to the complexity of the content it's processing. For sequences with low action, compression is maximized to reduce file size without any compromise in quality. But during intense action scenes, the codec judiciously allocates its processing resources to maintain smooth visuals, achieving a good balance between compression and visual fidelity.
This efficiency benefits multi-platform video distribution. It makes it easier to deliver high-quality content across a range of network conditions, meeting the demands of users with diverse internet speeds.
It's also noteworthy that H.265 can handle 4K UHD video while supporting High Dynamic Range (HDR). This is significant, as it lets platforms deliver richer color and higher contrast without the typical increase in file size that comes with superior visual experiences.
As video resolution pushes towards 8K and beyond, the adaptive and scalable design of H.265 positions it well for future video content. The codec's design clearly prioritizes efficiency and flexibility, which will continue to be crucial as video technology evolves.
How H265 Codec Reduces Video File Size While Maintaining Quality in 2024 - Adaptive Quantization Methods for Detail Preservation in Dark and Bright Areas

Adaptive Quantization (AQ) plays a critical role in preserving detail in videos, especially in scenes with a mix of bright and dark areas. This method intelligently adjusts how video data is encoded, focusing on keeping important details in both highlights and shadows. The codec adapts by allocating more "bits" (the building blocks of the encoded video) to crucial parts of the image and fewer to less important areas. This dynamic approach ensures that the video quality doesn't suffer from overly aggressive compression.
Two common AQ methods are used: Spatial AQ focuses on the differences within a single frame, and Temporal AQ considers how the scene changes over multiple frames. Both techniques help the codec distribute its encoding effort in a way that's tailored to the specific video. As our expectations for video quality grow with better displays and the need to handle higher-resolution video, these adaptive methods become even more important. This is especially true with the H.265 codec, which relies on these techniques to efficiently compress videos without significantly sacrificing image quality.
The continuous development of new and refined AQ strategies reflects the larger trend in video compression technology: finding better ways to squeeze high-quality video into smaller files, so it can be shared and streamed more efficiently. This is a constant challenge, as both video resolution and viewers' expectations continue to climb, making efficient encoding more important than ever.
Adaptive quantization methods are essential for preserving detail in both dark and bright parts of a video. Standard quantization techniques can sometimes flatten the image, losing crucial details in these areas. This is particularly noticeable in scenes with a wide range of brightness, a problem that these newer methods aim to solve.
The development of adaptive methods often draws from how humans perceive visual information. By focusing on areas that our eyes are more sensitive to, like high contrast regions, encoders can allocate more bits to preserve these details. This leads to a more visually pleasing experience by keeping important features intact.
Adaptive quantization cleverly allocates different quantization levels based on the local content of the frame. For instance, if a section has high luminance or intricate texture, it's given a higher fidelity encoding compared to uniform or less important areas. This tailored approach allows for a more efficient use of bits.
These techniques, in practice, can dramatically enhance encoding efficiency. They can reduce the data needed for less visually critical parts, like a plain background. Meanwhile, detailed regions in the foreground are preserved, leading to an optimized bit allocation scheme.
The idea of customizing the quantization matrix is particularly interesting. By adjusting this matrix based on scene analysis, encoders can finely control the emphasis on detail preservation. This ability to tailor the compression approach can help reduce encoding artifacts.
Further, adaptive quantization allows for improved contrast in high-dynamic-range (HDR) content. It's able to maintain the rich detail in dark areas (shadows) while preventing highlights from getting clipped, which can negatively impact visual quality in standard encoding.
The growing adoption of adaptive quantization methods as part of the core encoding standards in new codecs is a notable development. It reflects a broader recognition within the field that traditional quantization is no longer sufficient for the demands of current video content.
However, the enhanced detail preservation offered by adaptive techniques comes at a cost. These methods can be computationally intensive, leading to a slower encoding process. This necessitates a careful trade-off between quality and processing time, especially in scenarios like real-time video encoding.
Beyond standard video compression, the principles of adaptive quantization are being applied in related areas. For example, it's been useful in high dynamic range (HDR) image processing and certain computational photography techniques, suggesting its broader potential impact on visual technologies.
Researchers are actively investigating ways to improve adaptive methods even further. Current efforts often involve machine learning techniques. By using these algorithms, future codecs might be able to predict scene characteristics and allocate bits more precisely, leading to even better detail preservation as video technology evolves.
How H265 Codec Reduces Video File Size While Maintaining Quality in 2024 - Smart Color Space Management and HDR Integration
Within the context of H.265's role in reducing video file size while maintaining quality in 2024, "Smart Color Space Management and HDR Integration" has become increasingly significant, especially for high-resolution content. Handling color spaces correctly is crucial for ensuring HDR videos look as intended. If not managed well, color inaccuracies can diminish the viewing experience.
As video quality standards rise, combining HDR with advanced color space handling becomes important for delivering richer colors and details without sacrificing compression efficiency. This synergy between color space management and HDR underscores the importance of advanced encoding in achieving a balance between visual fidelity and file size optimization. Effectively integrating HDR is no longer just a desirable feature, but a foundational aspect of modern video production and distribution. It's a key factor in how viewers perceive and enjoy higher-quality video.
Smart color space management and HDR integration are increasingly intertwined with H.265, offering a glimpse into the future of video. The ability to flexibly use multiple color spaces, like Rec. 709 and Rec. 2020, within a single video stream, is intriguing. This adaptability is particularly important for HDR, which aims to reproduce a much broader range of colors and brightness levels than traditional standard dynamic range (SDR) video. We're talking about peak brightness levels potentially exceeding 1000 nits, which can significantly enhance visual experience.
However, the conversation around HDR often focuses on color gamut, which is a limited aspect of color reproduction. Smart color space management, ideally, should consider "color volume"—encompassing not just the range of colors but also the intensity and brightness. This holistic approach ensures more accurate color portrayal, especially in high-contrast scenes.
Furthermore, techniques like perceptual quantization (PQ) have emerged to optimize the way color data is encoded based on human visual perception. PQ utilizes a logarithmic scale, which means more bits are used to encode the details in darker regions, improving the visibility of subtle textures in those areas. It's a thoughtful way of distributing encoding resources based on what our eyes are most sensitive to.
But HDR integration presents unique hurdles, especially in managing shadow details and preventing the loss of information in dark areas. Clever algorithms are needed to maintain visual integrity in these scenarios. It's not surprising that the integration of HDR with H.265 improves compression efficiency. After all, the coding mechanisms are designed to be smart about HDR content dynamics.
One of the fascinating implications is that H.265 can reduce the storage burden without significant loss of visual fidelity, which is a testament to the synergy between HDR and modern compression techniques. We are seeing a continued evolution of HDR standards, like Dolby Vision, which aim to increase color depth to a remarkable 12-bits. This highlights a trend toward even more complex and sophisticated color space management in future video codecs.
However, the capabilities of smart color space management and HDR come at a cost. Real-time processing for these features can be computationally intense, especially in the case of live streaming. As resolutions and color depth continue to rise, it's clear that we will require increasingly capable hardware to meet these demands. It's a crucial consideration, particularly in a world where media consumption increasingly occurs through streaming platforms. These considerations, along with the challenges of ensuring consistent visual experiences across different platforms, suggest the journey toward optimal HDR integration is still ongoing.
How H265 Codec Reduces Video File Size While Maintaining Quality in 2024 - Parallel Processing Architecture for Faster Video Encoding
H.265's complexity necessitates advanced processing capabilities, particularly for encoding high-resolution video at acceptable speeds. To address this, parallel processing architectures have been developed, leveraging multiple processing units to perform encoding tasks concurrently. These architectures, featuring fully parallel hardware designs, can handle a wide range of coding operations, including the numerous prediction modes and coding tree unit partitions inherent to H.265. This approach leads to substantial speed increases – up to 20 times faster than traditional, serial processing methods. This speed boost is crucial for achieving real-time video encoding, enabling smooth 30 frames per second HD encoding.
While offering a clear advantage for processing power, parallel architectures also help mitigate the power consumption that typically accompanies high-performance video encoding. This is becoming increasingly important as resolutions continue to escalate. However, the inherent complexity of the H.265 encoding process presents a continuous challenge, prompting ongoing development and refinement of parallel processing techniques. Further advancements in this area are needed to fully optimize video encoding for the next generation of video resolution and data requirements.
The H.265 codec, also known as HEVC, was developed to significantly improve video compression compared to its predecessor, H.264. While achieving a remarkable 50% bitrate reduction while maintaining video quality, it presents a challenge: its complexity has increased the computational demands of encoding. This complexity necessitates efficient parallel processing to handle the encoding of high-resolution video in real-time.
Fortunately, H.265's architecture is inherently suited for parallel processing. This means multiple encoding tasks can be executed concurrently, significantly speeding up the process. The use of Coding Tree Units (CTUs) plays a role here. Instead of the older approach of using fixed rectangular blocks, H.265 uses these larger cubic regions for encoding. This new structure makes parallel processing more efficient.
H.265 is designed to distribute the workload dynamically across multiple processors. This lets individual processor cores tackle different parts of a video frame, optimizing how the CPU and GPU are used. This approach also extends to entropy coding—a technique used for compression. The encoding decisions are made more rapidly with parallel probability calculations, enhancing overall compression speed.
Moreover, the H.265 framework permits parallelism on both the frame and slice levels. This means the encoder can optimize its performance based on the specific complexity of the video content at hand. It can choose to process an entire frame or focus on slices of it.
It's also noteworthy that H.265 is inherently multi-threaded, which means multiple threads can handle different aspects of video data or different processing steps. This ability to split the encoding workload optimizes resource utilization. Furthermore, advancements in motion prediction in H.265 can be computed in parallel across different regions of the video frame, leading to both faster processing and higher accuracy.
Interestingly, H.265 is designed to leverage SIMD (Single Instruction, Multiple Data) instructions that allow parallel processing of multiple data points. This feature enhances efficiency, especially for high-resolution videos. The codec's ability to adapt to architectural changes like the evolution of multi-core processors and GPUs also ensures that it can always harness the benefits of parallel processing.
Some H.265 implementations have integrated a real-time feedback loop during encoding. This lets the system dynamically adapt its parameters based on processing load and video content. This adaptability ensures optimal use of available parallel processing resources, which benefits both encoding speed and quality.
While parallel processing holds tremendous potential, some research suggests that this type of processing can potentially result in a small loss in Peak Signal-to-Noise Ratio (PSNR), from 0.14dB to 0.77dB, when maintaining a consistent bitrate. The trade-offs between speed and quality need to be considered as these algorithms and their hardware are further developed.
Despite these minor trade-offs, it's clear that the H.265 codec's parallel processing architecture has become a vital aspect of modern video encoding. Its ability to adapt to complex video content, alongside its speed, ensures it will play a crucial role in supporting our growing reliance on high-quality, efficient video content in 2024 and beyond.
More Posts from specswriter.com: