Unveiling the Importance of MTTF A Critical Metric for Non-Repairable Asset Reliability
Unveiling the Importance of MTTF A Critical Metric for Non-Repairable Asset Reliability - Understanding MTTF Definition and Scope
Mean Time to Failure (MTTF) is a vital indicator of reliability for non-repairable assets. It represents the average time a system or component will function before failing under typical operating conditions. MTTF is typically measured in units of time, like hours, days, or years, and a higher MTTF implies greater reliability and longer periods between failures.
Understanding MTTF provides valuable insights into the expected lifespan of critical equipment, enabling organizations to plan maintenance and inventory management more effectively. Moreover, analyzing MTTF can identify potential weaknesses in product or system designs, prompting necessary improvements. MTTF is frequently used alongside other reliability metrics to obtain a comprehensive understanding of asset performance and reliability. In industries heavily reliant on non-repairable equipment, grasping the implications of MTTF is essential for informed decision-making and optimized operational efficiency.
Mean Time to Failure (MTTF) is a crucial metric for understanding the lifespan of non-repairable assets, but it's easy to confuse it with Mean Time to Detection (MTTD). It's essential to remember that MTTF incorporates both usage factors and environmental conditions, showing that reliability isn't just about design. While MTTF primarily focuses on non-repairable components, it also finds applications in manufacturing and IT where asset replacement is frequent, showcasing its broader utility.
The average MTTF for electronic components can vary drastically, highlighting the importance of careful product selection for achieving long-term performance. Statistical methods, heavily reliant on historical failure data, are used to calculate MTTF. This reliance on data means that MTTF can evolve as more information becomes available, allowing for proactive reliability assessments. Products with higher MTTF are often perceived as being of higher quality, leading to reduced warranty costs and improved customer satisfaction. This perception can directly impact a company's competitive edge in the market.
The application of MTTF becomes more complex in systems with redundancy built in, where the calculation must account for concurrent failures instead of individual component failures. In some cases, achieving a specific MTTF might necessitate trade-offs between cost and reliability. This demands careful optimization to balance budget constraints with performance expectations. MTTF plays a crucial role in risk management by allowing organizations to predict potential asset failures and allocate resources for replacements in a timely manner. Understanding MTTF is also essential for crafting effective maintenance strategies. By aligning replacement cycles closely with MTTF forecasts, organizations can minimize operational disruptions and enhance efficiency.
Unveiling the Importance of MTTF A Critical Metric for Non-Repairable Asset Reliability - Calculating MTTF The Statistical Approach
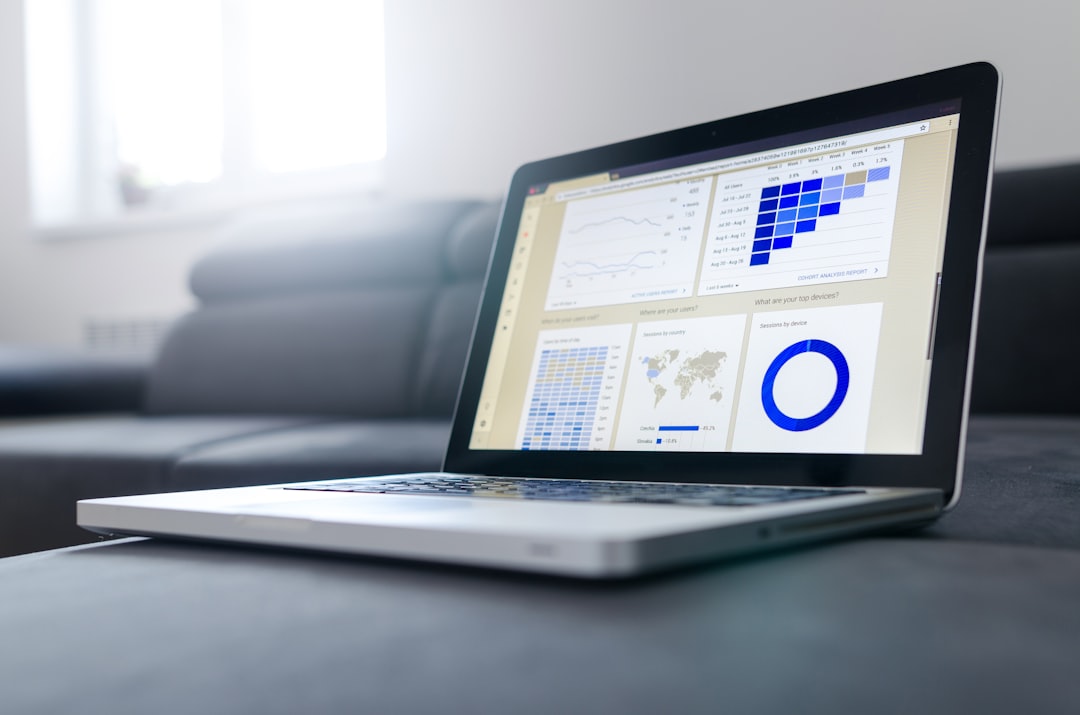
Calculating MTTF through a statistical approach relies on analyzing historical failure data to determine the average lifespan of a non-repairable system. The fundamental formula for calculating MTTF is the total operating time divided by the number of failures. This straightforward equation gives us a sense of the system's reliability. However, it's important to note that the accuracy of MTTF hinges on the quality and completeness of the data. Limited historical information can lead to inaccurate results. Imagine trying to predict the lifespan of light bulbs. While MTTF offers a good estimate, it can't predict the exact moment any individual bulb will fail. Ultimately, a solid understanding of MTTF is vital for organizations seeking to manage their assets efficiently and maintain high operational performance.
The statistical approach to MTTF dives deeper into the probability of a system surviving over time, using tools like survival analysis. This mathematical framework provides a more precise prediction of asset lifespan, though it relies heavily on data collected from controlled reliability testing. However, these tests may not perfectly capture the complexities of real-world operating conditions, potentially leading to discrepancies between calculated MTTF and actual performance.
While MTTF is useful, it's not the complete picture of reliability. Factors like wear, usage patterns, and environment play a significant role and can influence actual lifespan. Industries with high stakes, like aerospace and medical devices, utilize MTTF alongside other metrics, such as Hazard Rate and Reliability Function, to achieve a more holistic understanding of failure risks.
Interestingly, MTTF can expose design flaws. By analyzing MTTF across multiple prototypes, engineers may uncover consistent failure modes and address them before mass production, preventing costly rework later on. The statistical approach to MTTF is not limited to individual components; it can also be applied to entire systems, revealing how different components interact and affect overall reliability.
There is a clear connection between MTTF and the cost of ownership. Components with longer MTTF typically result in lower maintenance costs, allowing for more strategic asset acquisition and lifecycle management. This can also affect warranty strategies, as a product with a high MTTF is less likely to have warranty claims. This allows companies to reduce financial reserves allocated for warranty expenses and invest those funds elsewhere, like innovation.
In the semiconductor field, MTTF often follows technology trends. As transistors shrink, new and unexpected failure mechanisms can emerge, requiring constant adjustments to MTTF expectations in this rapidly evolving field.
It's important to remember that simply increasing MTTF doesn't necessarily guarantee improved reliability. Striking a balance between MTTF, cost, and performance requires careful analysis, as excessive focus on one aspect may unintentionally compromise others.
Unveiling the Importance of MTTF A Critical Metric for Non-Repairable Asset Reliability - MTTF vs MTBF Key Differences Explained
The distinction between Mean Time to Failure (MTTF) and Mean Time Between Failures (MTBF) is critical for effective asset management across various industries. MTTF focuses on non-repairable components, measuring the average time a component functions before failing from the moment it's installed. MTBF, on the other hand, applies to repairable systems, indicating the average time a system operates between failures before requiring maintenance. These metrics provide valuable insight into the reliability and performance of assets, guiding maintenance strategies and enabling organizations to optimize resource allocation and minimize downtime. A thorough understanding of both MTTF and MTBF is crucial for making informed decisions, especially in industries heavily reliant on assets.
While MTTF (Mean Time to Failure) is a crucial metric for non-repairable assets, it's often confused with MTBF (Mean Time Between Failures), a metric used for systems that can be repaired. This distinction is fundamental because it impacts how we understand reliability and plan maintenance. When calculating MTTF, relying solely on limited historical failure data can lead to inaccuracies. Environmental factors, like temperature and humidity, can significantly influence MTTF, meaning a single figure might not be representative across all situations.
While we usually think of MTTF in terms of individual components, it's equally applicable to entire systems. However, in complex systems with multiple components, it's difficult to determine the exact effect of each component on the overall system's reliability. Analyzing MTTF across different prototypes can unveil recurring failure modes, enabling engineers to refine designs for better reliability before large-scale production.
Higher MTTF often translates to lower costs throughout the asset's life cycle due to less frequent maintenance and replacements. This economic aspect is critical when making purchase decisions. But as technology advances, new failure mechanisms can emerge, necessitating constant adjustments in our understanding of MTTF.
In systems with redundancy, calculating MTTF and MTBF becomes more complex as concurrent failures can impact the system's overall reliability. Additionally, a product's MTTF can influence warranty strategies, as products with higher MTTF typically have fewer warranty claims, helping companies allocate resources effectively.
Ultimately, MTTF is a valuable tool for managing risk in industries that rely heavily on assets. By understanding and predicting potential failures, companies can proactively replace assets, improve operational resilience, and minimize disruptions.
Unveiling the Importance of MTTF A Critical Metric for Non-Repairable Asset Reliability - Applications of MTTF in Asset Management
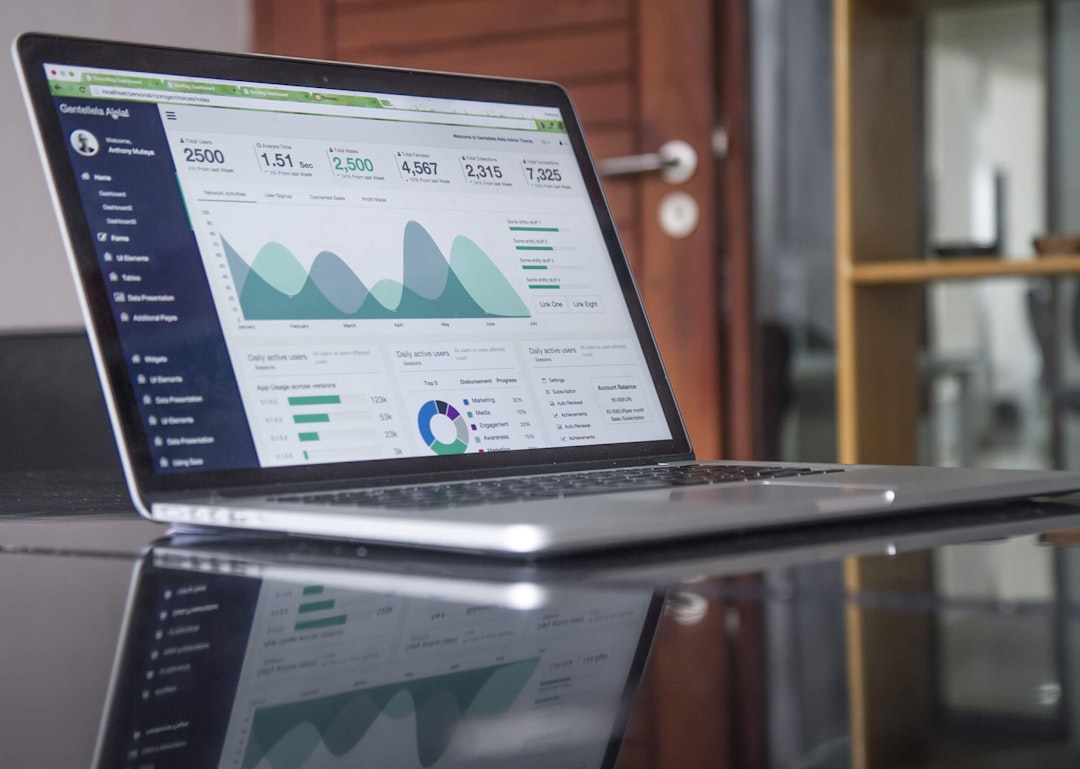
Within asset management, Mean Time to Failure (MTTF) isn't just a number – it's a guiding principle for making smart decisions about non-repairable assets. Knowing the expected lifespan of an asset, thanks to MTTF, helps companies plan when to replace it, ensuring they have the right parts in stock. This foresight also helps them decide when to schedule maintenance, minimizing downtime and maximizing efficiency.
But MTTF isn't a magic bullet. It's important to remember that real-world conditions, like the environment and how the asset is used, can have a big impact on how long it actually lasts. A high MTTF might make a product seem great, but if it's not used correctly or in a suitable environment, it'll still fail sooner than expected. So, while MTTF is a helpful tool, it shouldn't be the sole factor driving decisions.
By understanding the interplay between MTTF and operational factors, businesses can make smarter choices, ensuring their non-repairable assets perform well for as long as possible. This leads to smoother operations, reduced costs, and a competitive edge in the long run.
MTTF, a measure of how long a non-repairable asset lasts, plays a crucial role in asset management, but it’s not without its complexities. It helps determine when to replace equipment, minimizing downtime and aligning replacements with budget constraints. However, MTTF values vary across industries due to different operating conditions, component quality, and usage patterns. To get an accurate picture, you need to tailor your reliability assessment for each specific situation.
By comparing MTTF across multiple versions of a product, companies can identify common failure modes and make improvements before large-scale production, ensuring better quality. But remember, MTTF relies heavily on data. Poor data quality can lead to misleading estimates, so make sure you have complete and accurate information.
Interestingly, a high MTTF is often associated with a reliable product, leading to more favorable warranty terms and reduced warranty claims for companies. But to be statistically sound, your MTTF calculations often require large data samples. Smaller sets can skew results, so you’ll need to collect enough data for accurate reliability models.
Higher MTTF also has a direct impact on the bottom line. Assets that last longer require less frequent replacements and maintenance, resulting in lower operating costs. The concept of redundancy, however, complicates things. In systems with redundant parts, it becomes tricky to assess MTTF, and you might need complex statistical models to get an accurate measure.
The tech world is constantly changing, especially with semiconductors, and new failure mechanisms emerge. This calls for a continuous reassessment of legacy MTTF data and methodologies to stay ahead of the curve. Beyond traditional uses in manufacturing and IT, MTTF is becoming increasingly relevant in fields like aerospace and healthcare, where component reliability is critical to safety and mission success.
Unveiling the Importance of MTTF A Critical Metric for Non-Repairable Asset Reliability - Impact of MTTF on Maintenance Planning
Mean Time to Failure (MTTF) plays a crucial role in maintenance planning because it provides a way to predict how long assets will last before they need to be replaced. When you know the MTTF for a specific type of asset, it becomes easier to manage inventory by having the right parts on hand at the right time. This also helps to proactively schedule maintenance, minimizing downtime and ensuring smooth operations.
While MTTF is a powerful tool, it's not a guaranteed crystal ball. Factors like how the asset is used and the environment it operates in can affect its lifespan. Over-relying solely on a single MTTF value can lead to inaccurate assessments. Balancing the information from MTTF with real-world factors lets organizations fine-tune their maintenance strategies, optimizing their asset management for better efficiency and cost-effectiveness.
The impact of Mean Time To Failure (MTTF) on maintenance planning can be substantial, but it's not a simple equation. It's essential to be cautious about relying solely on historical data, as inaccuracies can lead to misleading MTTF calculations and misguided maintenance decisions. For example, if historical data is incomplete or inconsistent, it might lead to an overestimation of an asset's reliability.
Furthermore, systems with redundancy, a common feature in complex systems, present unique challenges for MTTF analysis. Traditional MTTF methods might not adequately capture the complexities of redundant systems, making accurate maintenance planning even more difficult. It's important to remember that environmental conditions and usage patterns play a significant role in influencing MTTF. While the initial design of a product might contribute to its expected lifespan, actual operational conditions and user behavior can significantly affect its MTTF.
The influence of user behavior on MTTF highlights the importance of comprehensive maintenance planning. By understanding how users interact with equipment and the potential for misuse, maintenance planners can address these factors in their planning to prevent premature failures. MTTF data across various prototypes can reveal critical insights about systemic design flaws. This information can help engineers refine designs before production, minimizing costly rework and maximizing reliability.
A longer MTTF can have a positive economic impact on companies. Less frequent replacements mean lower lifecycle costs, freeing up resources for innovation and other strategic initiatives. However, it's important to note that the accuracy of MTTF calculations hinges on the size and quality of the data set. Smaller, less comprehensive datasets can significantly skew MTTF estimations, requiring careful analysis and potentially larger datasets for more reliable results.
MTTF figures can also vary significantly between different industries, depending on operating conditions and specific usage patterns. While a manufacturing company might be satisfied with a certain MTTF, an aerospace company might demand significantly higher reliability, making a tailored approach to MTTF estimation crucial in different sectors.
Furthermore, the dynamic nature of technologies, especially in fields like semiconductors, necessitates continual reassessment of MTTF data and calculations. As new failure mechanisms emerge with advancements, maintaining the accuracy and relevance of MTTF estimations is crucial. This can involve adjusting methodologies, collecting new data, and incorporating the latest insights into maintenance plans. Overall, while MTTF offers valuable insights into the expected lifespan of non-repairable assets, understanding its limitations, complexities, and influence on maintenance planning is essential for effective asset management and operational efficiency.
Unveiling the Importance of MTTF A Critical Metric for Non-Repairable Asset Reliability - Leveraging MTTF Data for Operational Efficiency
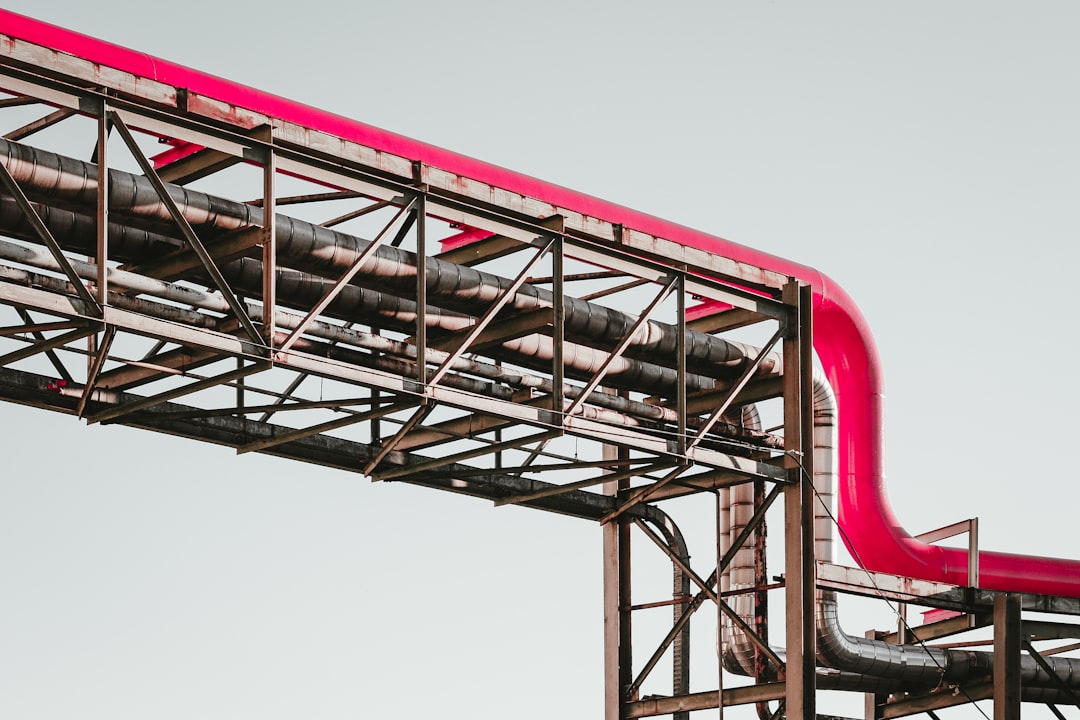
Leveraging MTTF data is crucial for optimizing operational efficiency when dealing with non-repairable assets. By understanding the average time a component is expected to function before failing, organizations can anticipate when replacements will be needed, streamlining inventory management and minimizing downtime. However, relying solely on MTTF can be misleading, as its accuracy is heavily influenced by real-world usage and environmental factors. For instance, a component with a high MTTF might fail prematurely due to harsh operating conditions or improper handling. Therefore, organizations must be cautious in their application of MTTF data, carefully considering these external influences to avoid inaccurate assessments that could lead to operational disruptions. Ultimately, successful implementation of MTTF requires a thoughtful understanding of the specific circumstances surrounding each asset, enabling organizations to achieve improved reliability and efficiency.
MTTF, or Mean Time To Failure, is a crucial metric for assessing the lifespan of non-repairable assets. However, there are several nuances and complexities that influence its effectiveness. It's not a one-size-fits-all solution and requires careful consideration of specific industry contexts and factors.
For example, MTTF values can differ dramatically depending on the industry. Aerospace components, for instance, often have significantly higher MTTF than consumer electronics, reflecting the critical importance of reliability in such sectors. Additionally, the quality of historical data is paramount in accurately calculating MTTF. Inaccurate or incomplete data can lead to misleading estimates and potentially flawed decision-making.
Moreover, MTTF calculations typically don't explicitly account for the gradual effects of wear and tear. As assets age, they may not necessarily meet initial MTTF estimations, prompting the need for a more nuanced approach to maintenance planning. For a robust MTTF estimation, large datasets are critical, smaller datasets can result in unreliable calculations, potentially leading to inaccurate conclusions about asset reliability.
One significant benefit of utilizing MTTF data effectively is its ability to minimize unplanned downtimes. By leveraging MTTF predictions, companies can ensure that replacement parts are readily available before end-of-life scenarios, potentially reducing unplanned downtime by as much as 25%. This leads to enhanced operational efficiency.
However, systems with redundant components pose a unique challenge for MTTF calculations. Since there are multiple failure paths within such systems, advanced statistical models are needed to account for the complexities and provide reliable reliability predictions. Additionally, the way users interact with assets can significantly influence their lifespan. Improper usage or harsh operating environments can shorten an asset's lifespan, resulting in deviations from expected MTTF values.
Analyzing MTTF across different prototypes can be valuable in identifying recurring design flaws early on. This enables proactive modifications before full-scale production, enhancing reliability and minimizing the need for costly rework later. Furthermore, rapid technological advancements, especially in fields like semiconductors, can bring about new failure mechanisms that weren't previously anticipated. As technologies evolve, MTTF estimates need regular updates to reflect these shifts and maintain accuracy.
Products engineered with a high MTTF typically enjoy a better market reputation and result in fewer warranty claims. This translates to significant savings for companies, allowing them to allocate more resources toward innovation and research rather than repair costs.
While MTTF offers valuable insights into non-repairable asset lifespan, understanding its limitations and complexities is essential. A nuanced and data-driven approach is critical for achieving accurate MTTF estimates and harnessing its benefits for effective asset management and operational efficiency.
More Posts from specswriter.com: