Transform your ideas into professional white papers and business plans in minutes (Get started for free)
Patent Analysis AI Video Generation Models Trained on TikTok Content - Technical Implementation and IP Landscape
Patent Analysis AI Video Generation Models Trained on TikTok Content - Technical Implementation and IP Landscape - Training Data Architecture Behind ByteDance MagicVideoV2 Model
ByteDance's MagicVideoV2 model presents a novel approach to AI-driven video generation, integrating a series of modules into a cohesive pipeline. This includes a text-to-image converter, a mechanism to generate video movement, a module for processing reference images, and a module for smoothing out video frames. This integrated structure allows for efficient generation of high-quality video content from text descriptions.
The core of MagicVideoV2 utilizes latent diffusion methods, a technique that efficiently translates text into video. The model's 3D U-Net architecture is notable for its ability to produce video clips at 256x256 resolution, a feat achievable using just a single GPU and reportedly much faster than prior techniques. This design choice is likely driven by a need for scalable video generation, a critical element for the sheer volume of content found within platforms like TikTok.
Instead of training directly on the standard RGB color space, MagicVideoV2 uses a pre-trained Variational Autoencoder (VAE). This allows it to compress video clips into a more compact, latent space, making the process of learning how videos are distributed more efficient. This architectural choice, along with the other optimizations, likely contributed to the substantial speed improvements compared to older models.
The developers of MagicVideoV2 emphasize aesthetic quality and fidelity, highlighting its performance compared to other advanced models. This aligns with the increased demand for AI tools that can produce visually appealing content, something that becomes paramount in the competitive landscape of social media and user-generated content. The model's ability to produce such content within the TikTok context suggests a potentially significant impact on the future of how users create and interact with video content on platforms.
The development of MagicVideoV2 demonstrates ByteDance's continued investment in the advancement of AI in generative modeling, a field likely to see continued evolution in the years to come. This approach suggests that future platforms and tools for online content creation might leverage AI in sophisticated ways, with the ultimate goal of producing compelling and immersive video experiences for users.
ByteDance's MagicVideoV2 model employs a sophisticated pipeline that merges different components – a text-to-image converter, a video motion engine, a reference image embedding module, and a frame interpolation module – to generate videos from scratch. The goal, clearly, is to generate high-quality videos solely based on text prompts, a testament to the strides in AI-powered video creation.
Interestingly, they've adopted latent diffusion methods to achieve this text-to-video transformation. It's an efficient approach, capable of crafting highly realistic outputs. Utilizing a 3D U-Net structure, the model can create videos at a resolution of 256x256, all while running on a single GPU—a significant improvement in efficiency, supposedly 64 times faster than earlier models. ByteDance emphasizes its superior performance over other text-to-video models like Runway's Gen2 or Stable Video Diffusion, notably in terms of visual appeal and detail.
However, unlike many other video generation models, MagicVideoV2 avoids direct training within the typical RGB color space. Instead, it relies on a pre-trained Variational Autoencoder (VAE) to condense the video data into a more manageable latent space. This strategy seems designed to optimize the training process, allowing the model to learn the distribution of video content more effectively.
This architecture allows for the generation of smooth, coherent video clips by capturing video patterns within this compressed latent space. This design represents a considerable improvement over prior video generation techniques, promising to unlock new possibilities for content creators. It's also suggestive of ByteDance's larger ambitions – pushing the boundaries of AI in generative modeling and influencing the future of digital content creation. But one could also view it as a move to assert control over the evolving field of video generation, particularly within TikTok's ecosystem. The overall implications of this technology on user creativity and content control deserve close consideration.
Patent Analysis AI Video Generation Models Trained on TikTok Content - Technical Implementation and IP Landscape - Machine Learning Implementation for TikTok Video Hashtag Processing
TikTok's video recommendation system heavily relies on machine learning to process the hashtags used in videos. This involves using techniques like natural language processing and metadata analysis to understand the meaning and context behind the hashtags. The platform analyzes many factors related to the videos, including popular hashtags and surrounding details, to tailor recommendations to individual users. This ability to analyze hashtag data improves user engagement by surfacing relevant content. It also highlights the constantly evolving nature of social media platforms and how AI impacts the way people create and interact with content. However, this advanced filtering and recommendation system raises important concerns about user privacy and data management, prompting ongoing discussion about how such technology should be implemented responsibly. The ever-changing nature of TikTok's algorithm, driven by this machine learning approach, continues to shape the landscape of how video content is created and consumed.
TikTok's recommendation system relies heavily on machine learning, using computer vision, natural language processing (NLP), and metadata analysis to understand video content and connect users with what they might like. Computer vision, a branch of deep learning, enables TikTok to analyze the visual aspects of videos, essentially "seeing" what's in the content. NLP plays a role by creating text transcripts of videos, allowing the platform to "understand" the spoken or written content. It's through these techniques, combined with analyzing data like music, filters, and of course, hashtags, that the system attempts to gauge the likely appeal of a video to a particular user.
Their algorithm relies on a recommender system that tailors suggestions based on individual user profiles and preferences. This, coupled with the massive data generated by TikTok's user base (over 283,000 unique videos in one training dataset alone), makes it incredibly efficient. The IP landscape around TikTok is naturally evolving as well, with a growing number of patents centered around AI-driven video processing techniques. We're seeing more research focused on TikTok's algorithm itself—how it affects data privacy and user behavior—and on automated video creation using AI, potentially further changing how content is made and shared.
There have been some interesting leaks of internal documents which shed some light on how TikTok's engineering team works. They suggest TikTok's dependence on huge datasets to build user profiles and deeply understand the content on the platform.
One could speculate that the constant development of these AI features is not just about enhancing the user experience but also likely about increasing engagement and user data collection for ByteDance, the company behind the platform. There's a lot more to be understood about the role of these algorithms and what impact they have on the way people use TikTok and generate content. The potential influence on privacy and content control raises legitimate questions that need to be discussed as this field continues to rapidly develop. It's fascinating to see how such a young platform is already utilizing AI in a vast array of ways, with significant potential, and risks, for both creators and viewers.
Patent Analysis AI Video Generation Models Trained on TikTok Content - Technical Implementation and IP Landscape - Technical Framework of Short Form Video Generation Models
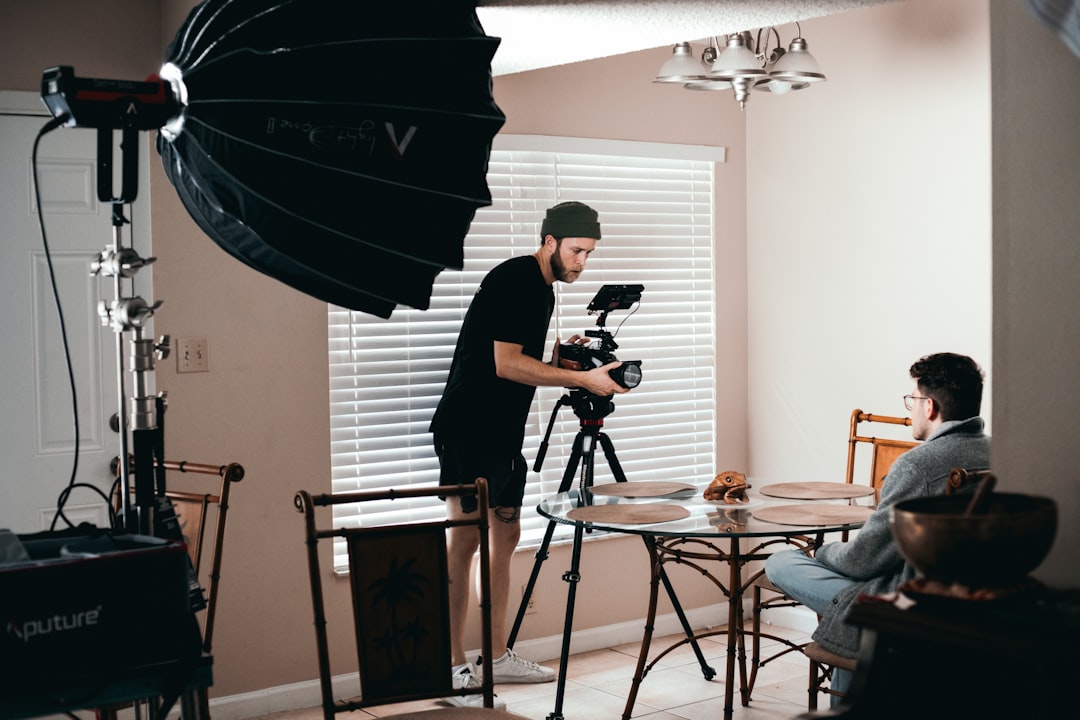
The technical foundation of short-form video generation models encompasses a range of innovative approaches for crafting high-quality videos. These models leverage techniques like text-to-video (T2V), image-to-video (I2V), and video-to-video (V2V) generation, significantly impacting content creation across various platforms. Underlying these models are often intricate architectures that incorporate tools like transformers, designed to streamline the process of creating videos with diverse lengths and resolutions. Models such as OpenAI's SORA demonstrate the growing capacity to automate the generation of videos, producing lifelike and detailed outputs based on simple textual instructions. While these advances hold great potential for content creation and user experience, they also raise critical questions about how much control users have over their own data, especially when dealing with large amounts of user-generated content seen in environments like TikTok. The potential implications of these rapidly developing technologies for data ownership and privacy remain a vital area of discussion.
The field of short-form video generation is rapidly advancing, with models like MagicVideoV2 demonstrating impressive capabilities. These models leverage latent diffusion methods to efficiently transform text prompts into high-quality video content, showcasing the interplay between language understanding and video synthesis. It's a fascinating blend of seemingly disparate fields.
One key innovation is the use of a pre-trained Variational Autoencoder (VAE) in models like MagicVideoV2. By compressing video data into a latent space, the VAE reduces the complexity and volume of training data needed, ultimately speeding up the learning process. This is a clever strategy for handling the massive amounts of data involved in training video generation models.
The architecture of models like MagicVideoV2 often utilizes a 3D U-Net, allowing them to create videos at resolutions like 256x256 while maintaining low computational needs. This is particularly important for real-time applications, crucial for a platform like TikTok where immediate responses are expected. But we still have a lot to learn about how to balance quality and speed for different use cases.
TikTok's patent WO2014068309A1 shows us another trend—the development of robust action recognition systems. These systems rely on vast datasets to learn to differentiate between even subtly different actions within a video. They aren't just recognizing actions, they're beginning to understand the context and meaning behind them, a significant step forward.
Interestingly, models like MagicVideoV2 often veer away from the conventional RGB color space, opting for a more compact latent representation. While this might seem like a minor detail, it can have a significant impact on both quality and efficiency, suggesting a shift in the strategic design choices being made within AI-driven video models.
TikTok's action recognition system also incorporates hierarchical models, allowing it to analyze actions at multiple levels of abstraction. This enables a deeper understanding of user interactions, paving the way for more refined, personalized content recommendations. It’s a clever example of how we can use AI to adapt to individual user preferences in a nuanced way.
TikTok's recommendation algorithms are continually evolving, adapting based on user engagement patterns. They don't just analyze video content and hashtags through machine learning, they’re continuously learning and adjusting to optimize viewer experience. This creates a constant feedback loop, which is beneficial for the user, but also raises questions about how transparent these algorithms are.
The use of optical flow data in action recognition illustrates a sophisticated way to capture motion patterns. This allows these systems to effectively capture the inherent dynamism found in user-generated content, providing a richer source of information for the model to learn from. This seems to be a good approach to leveraging the complexity of user-created content.
For a platform like TikTok, real-time video processing is a must-have feature, demonstrating the convergence of rapid video analysis and machine learning. The platform needs to keep up with the ever-increasing demands of users for content that is both engaging and instantly accessible.
Finally, the enormous datasets that power many of these AI video systems are striking. The scale of the training data—like the 283,000 unique videos in one TikTok dataset—illustrates the massive computational resources required for effective AI video processing. As content volume continues to skyrocket, we have to wonder about the long-term scalability of these models and the potential limitations they might face. It's an important issue to consider as we think about the future of these technologies.
Patent Analysis AI Video Generation Models Trained on TikTok Content - Technical Implementation and IP Landscape - Legal Boundaries and IP Protection Methods for AI Generated Content
The legal landscape surrounding AI-generated content, particularly within the realm of video creation, is still developing and uncertain. Current copyright law often hinges on the idea of human authorship, creating challenges when it comes to determining who owns content generated solely by artificial intelligence. As AI models become more advanced and capable of creating diverse types of content, traditional intellectual property laws struggle to keep pace. This leads to questions about the originality of AI-generated work, and the legality of using copyrighted material to train AI models. Governments and legal bodies are starting to address these concerns and consider changes to existing laws. However, a clear and consistent approach to IP protection for AI-generated content remains elusive. This ongoing challenge emphasizes the need for more defined guidelines and practical tools to assist creators who are exploring the possibilities of generative AI while understanding the potential legal implications of their work. The use of copyrighted content within AI training datasets and the lack of established guidelines continue to be a crucial concern for the field.
The legal landscape surrounding AI-generated content, especially from models like ByteDance's MagicVideoV2, is currently hazy. It's unclear who, if anyone, legally owns content created solely by an AI. This is a significant issue, especially as AI models increasingly become capable of creating original works.
Companies are increasingly patenting the underlying technologies that drive AI models. This trend can limit others from developing similar techniques without risking patent infringement. It's a common pattern we've seen with other technological advancements, with patents establishing control over certain core techniques.
AI models trained on large platforms like TikTok rely on vast amounts of user-generated data. This raises substantial questions about who owns that data and whether users are aware of how their content is being used to train AI models. The ethical implications of this are still unfolding and potentially could impact future user expectations of content creation platforms.
Perhaps, we'll see new types of intellectual property (IP) rights specifically for content made by AI. This could lead to a redefined understanding of how ownership and rights are managed for AI-produced media. It's an intriguing prospect, as the very nature of “authorship” is being redefined within this context.
Governments are still playing catch-up in this area. New regulations might be needed to clarify IP rights related to AI-generated content. These new rules could reshape existing copyright law and ultimately impact the future of creative work. The challenge is to create laws that encourage innovation while also protecting creators' rights.
It's possible that the algorithms powering platforms like TikTok can subtly reflect or amplify societal biases. This raises important questions about content moderation and how we should ensure ethical practices when AI is involved in content filtering and recommendation systems. It's a crucial topic because AI has a lot of power to influence people's views and decisions.
AI content generation arguably challenges traditional copyright. Copyright laws usually focus on the concept of human originality and intent. AI doesn't have the same creative intent as a human artist, so the legal questions of "originality" become harder to define. This is going to be a key area for future legal challenges.
The increasing availability of open-source AI models is a double-edged sword. While it helps democratize access to advanced technologies, it also creates complexities in managing IP and enforcing rights when contributions come from various sources. It’s a tricky balance of making tools accessible and preventing misuse or accidental copyright infringement.
To maintain a competitive edge, AI model developers might leverage trade secrets to protect their innovations. This offers a different approach to IP protection compared to patenting. It’s a strategic decision, and often a delicate balance of protecting confidential algorithms and keeping research open to collaboration.
As AI-driven creative tools become commonplace, we can expect new kinds of legal disputes related to content ownership. These disputes will likely highlight the urgent need for clear and adaptable legal frameworks that can keep up with advancements in AI. This is a vital area of legal research as it becomes clear that existing frameworks don't quite address this new landscape of creative possibilities.
Patent Analysis AI Video Generation Models Trained on TikTok Content - Technical Implementation and IP Landscape - Cross Platform Integration Methods for Video Generation Models
The ability of video generation models to integrate seamlessly across different platforms is becoming increasingly important as content creation expands across diverse online spaces. The goal is to create AI models that can effortlessly handle various types of data and interact smoothly with a variety of user interfaces, making them practical and useful within specific environments, like TikTok. The rise of diffusion-based methods for video creation, distinct from older techniques, has led to more realistic and higher-quality video outputs. This is further amplified by training these models on vast, unified collections of visual data, allowing them to scale to handle increasingly large datasets. But there are some significant drawbacks to consider. There are ongoing concerns about the appropriate and ethical use of content produced by users on these platforms, especially in light of the huge amount of user-generated content. The question of ownership of AI-generated outputs also remains a challenge. Moving forward, the existing legal framework surrounding intellectual property needs to adapt and develop new rules to accommodate these issues, while also encouraging ethical development and encouraging innovation within the field of AI-driven video generation.
AI video generation is a dynamic field, with models like those trained on TikTok content showing a lot of promise. But when we start thinking about using these models across different platforms, a whole new set of challenges emerges. One of the more obvious issues is the sheer variety of how different platforms handle video data. Models built for mobile may not translate easily to web applications, for example, because the hardware and software environments are so different. This highlights a crucial problem: a lot of extra work is needed to make these models work well across platforms.
Another complication is getting data to play nicely across platforms. TikTok might store video metadata differently than YouTube or Instagram. If we want to create a unified model that can generate videos from various sources, we need to develop sophisticated ways to standardize the data and minimize the processing bottlenecks. This is a computationally intensive task and could be a significant obstacle to broader adoption.
Even though many video generation models boast real-time capabilities, that's not always true when we're working across platforms. Getting a model to generate video at a good quality and refresh rate requires a fair amount of computing power. Most common GPUs might not be up to the job, especially if the model needs to pull in information from multiple sources in real time. It's like trying to juggle multiple high-definition video streams at once—it can get complicated quickly.
Furthermore, the ways people interact with video content differ from one platform to another. A model trained to work with TikTok's quick, short-form videos might not handle longer, more narrative-based content elsewhere. Retraining or modifying these models for different interaction types can be time-consuming and resource-intensive.
Even if a platform offers an API, it's unlikely to be the same across the board. This can impact the ability of generative models to tap into a wide range of user-generated data, limiting how much training data they can collect. It's important to think about the ways in which platform-specific limitations influence the capabilities of models designed for wider application.
We also need to be mindful of potential bottlenecks in speed. If a model is designed to take input from multiple sources, latency can build up over time. This is particularly problematic for quick-moving platforms like TikTok, where fast refresh rates are expected. Any sort of slowdown during the integration process can impact the user experience.
In addition, different platforms have different standards for what is considered appropriate content. A model trained on one platform might generate output that violates the rules of another. This forces us to think carefully about how models are constructed to make sure they don't accidentally cause trouble in new environments.
The legal side of things gets complex as well, since each platform has its own IP rules and policies. Developers need to carefully consider the intellectual property ramifications when integrating models across different platforms.
The feature extraction process itself might not be consistent across different platforms. If a model is primarily trained on short videos, it may not generalize well to different types of videos, for instance, longer form or live-streamed content. We might find it difficult to create a single video model that can successfully leverage diverse video formats.
Finally, there are ethical implications when integrating AI models across platforms. The question of data privacy is a significant concern when we're pooling information from various sources. Different platforms have different policies for data access, and it's not always easy to reconcile these with each other. Ensuring that any data collected adheres to the rules set by a platform—while also meeting general data privacy laws—presents a significant challenge as we explore new possibilities for AI-based content generation.
It's clear that cross-platform integration is a vital part of making AI video generation technology more widely applicable. However, achieving this in a way that maintains performance, efficiency, and ethical considerations is a difficult engineering challenge. As the field progresses, we need to address these limitations and push the boundaries of how these models function across a diverse range of applications.
Transform your ideas into professional white papers and business plans in minutes (Get started for free)
More Posts from specswriter.com: