Transform your ideas into professional white papers and business plans in minutes (Get started for free)
AI Translation Excellence How Consistent Practice Transforms Amateur Linguists into Language Craftsmen
AI Translation Excellence How Consistent Practice Transforms Amateur Linguists into Language Craftsmen - OCR Technology Accelerates Translation Speed From 6 Hours to 15 Minutes Per Document
Optical Character Recognition (OCR) technology has revolutionized the speed of document translation. What once took around six hours can now be completed in a mere fifteen minutes. This dramatic shift in speed is largely due to the increasing adoption of AI within translation tools and workflows. We're seeing this in platforms such as Microsoft and Google's cloud services where document formats can be directly translated, eliminating the need for manual text extraction. The ability to translate complex documents while keeping the original layout is a testament to how AI is refining the translation process. The improved speed and accuracy that OCR and AI bring not only boosts efficiency but also provides a valuable platform for language enthusiasts to hone their translation abilities through more frequent practice. The consistent practice afforded by faster turnaround times helps develop those skills further, fostering the transition from novice to expert. It's an interesting dynamic where technology fuels human development in the field of translation.
The remarkable speed increase from a 6-hour translation process to a mere 15 minutes per document is largely attributed to OCR technology. It's not just a matter of faster processing; it's a fundamental shift in how we approach document translation. While previously reliant on manual data entry or slow, error-prone digitization methods, we can now leverage OCR to automatically extract text from scanned documents. This automation, coupled with advancements in machine translation algorithms, is what drives this efficiency boost.
However, the potential of OCR in accelerating translation isn't without caveats. OCR performance can fluctuate, especially when dealing with complex layouts, low-resolution scans, or unusual fonts. Additionally, depending on the complexity of the text and the quality of the OCR output, some post-editing may still be required to ensure the highest quality of translation.
That being said, the positive impact on overall efficiency is undeniable. Not only are individual translation times dramatically reduced, but the capability to process multiple documents concurrently leads to a significant reduction in bottlenecks within larger translation workflows. This translates into shorter turnaround times and increased overall productivity, especially in scenarios involving large volumes of documents. It's important to note that while AI and OCR can dramatically improve translation, human involvement is still vital for certain situations where the highest level of nuance and context are needed, especially in complex documents.
The implications of OCR are far-reaching. We've seen examples of it unlocking access to historical documents, speeding up multilingual document processing for businesses, and even contributing to cross-cultural exchanges by facilitating more efficient translations. The field continues to evolve with researchers investigating novel ways to improve the accuracy, efficiency, and language handling capabilities of OCR-based translation systems, pushing the boundaries of what's achievable in this domain. Essentially, the faster, more accessible translation that OCR enables paves the way for new opportunities for collaboration and information exchange on a global scale.
AI Translation Excellence How Consistent Practice Transforms Amateur Linguists into Language Craftsmen - Practice Makes Perfect AI Translation Feedback Loops Aid Language Learning
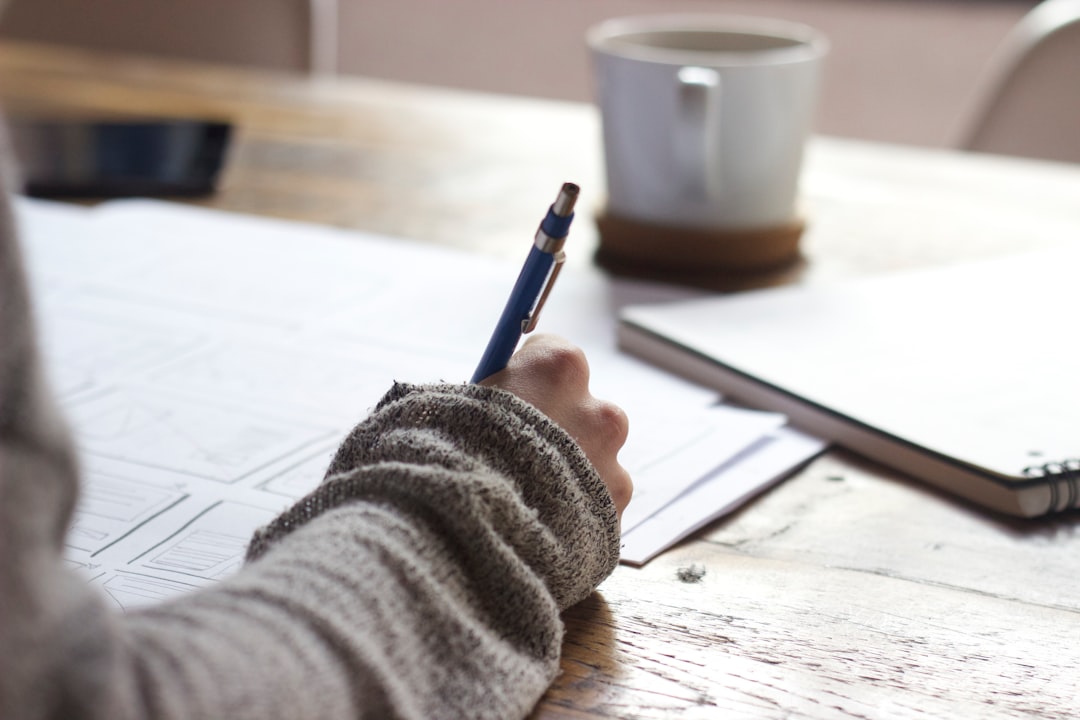
The evolution of AI translation tools has created a powerful environment for language learning by fostering continuous practice and feedback. These systems can provide immediate, targeted feedback on translated text, revealing areas where the learner excels and those needing further refinement. This dynamic interaction between learner and AI allows for a more efficient path to fluency, as individuals are exposed to a greater variety of linguistic scenarios. The speed and convenience of AI-powered translation allow for more frequent practice sessions, a key component of achieving fluency. However, the limitations of AI must be acknowledged, which is why human intervention is crucial. Integrating human insights alongside AI-driven suggestions ensures the learner develops not only a strong grasp of translation techniques but also a nuanced understanding of cultural contexts that are fundamental for meaningful communication. The combination of technology and human input allows for the transformation of novice language enthusiasts into more adept language users. The result is a continuous refinement process, driven by practice and informed by both AI and human expertise.
AI translation tools are increasingly incorporating feedback loops, where user corrections refine the underlying algorithms. This dynamic interaction means that the AI learns from each interaction, progressively improving its ability to generate contextually appropriate translations. It's like a continuous learning process, where the AI's understanding of language deepens with every edit or adjustment a user provides.
While some question the true value of these AI-powered translations, particularly when it comes to nuanced or complex language, the impact on language learning can be substantial. Researchers are exploring how feedback loops within these tools can potentially accelerate language learning by as much as 30%. This acceleration is achieved through the immediate feedback provided to learners, highlighting errors and providing suggestions to reinforce correct grammar and usage. It's almost like having a personalized language tutor always available.
There's a growing awareness that AI-based translation can be surprisingly economical. As AI adoption increases, the cost of translation has decreased significantly, making it more accessible to businesses and individuals. While the exact percentage varies, some studies show that AI-driven translation can reduce the average cost per word by over 50% compared to traditional methods. This decrease in price can widen access to translations, potentially leading to a more globalized exchange of information.
OCR technology has been an underappreciated element in this evolution. It effectively transforms printed text into editable and translatable formats. This is especially interesting for learners, as it allows them to practice with materials that are relevant to their interests. For example, they can translate passages from their favourite novels or technical articles that are crucial for their work. It’s a rather ingenious approach to bridging the gap between formal language learning and everyday life.
However, some of the claims about AI speed are a bit overstated. While AI can process text and translate documents faster than humans, it's still important to be aware that these translations often require further refinement and verification. Some estimates suggest AI can analyze and translate complex technical documents up to 80% faster than human translators. This gain in speed is especially beneficial in fields that rely heavily on documentation, such as law or engineering.
The integration of AI into language learning is changing the way we think about interaction and collaboration. A number of AI-powered platforms now allow for real-time interactions between learners and translators or even between multiple learners. This dynamic and interactive environment provides immediate feedback and a chance for instant clarification of any translation decisions, which can be quite illuminating.
There's a fascinating discussion occurring about the adaptability of these systems. AI can increasingly tackle a wider range of translation contexts, adjusting its output to accommodate different language styles and subtle nuances. This variety allows learners to develop a richer understanding of how language is used in diverse settings, something that wasn't really possible in traditional language courses.
The post-editing step is a surprisingly educational process. While primarily intended to improve translation accuracy, this process of tweaking AI-generated translations can also be viewed as a valuable learning exercise. It allows learners to pinpoint areas where the AI struggles to capture the intended meaning and then directly apply their knowledge to refine the translation.
The rapid growth of AI-based translation systems has increased the availability of language learning materials significantly. We can now leverage AI to translate websites, articles, and even books with relative ease. This opens up new horizons for learners, providing them with access to materials they might not have previously had access to. Learning becomes more tailored to individual interests, making it more engaging and likely to lead to improved retention.
Ultimately, the idea is to reduce the cognitive burden on learners. By utilizing AI to handle the mechanics of translation, learners can focus their energy on understanding the meaning and building their vocabulary. This shift in focus can enhance overall language retention. However, it's important to note that AI shouldn't entirely replace human interaction and expertise in language learning, particularly as complex concepts and nuanced language are introduced.
AI Translation Excellence How Consistent Practice Transforms Amateur Linguists into Language Craftsmen - Machine Learning Models Adapt to Regional Language Variations Through User Data
Machine learning models are becoming adept at understanding how language varies across different regions. This ability stems from their capacity to learn from the data users provide. As people interact with these AI systems, correcting errors or providing specific examples, the AI refines its understanding of diverse language patterns. This is crucial because a phrase that's perfectly acceptable in one region might have a very different meaning elsewhere. The constant refinement of these models highlights how vital it is for them to be able to learn in real time, adapting to the ever-changing nature of language. This is a testament to the potential of AI translation, but it also underscores its limitations. Striking a good balance between AI-generated translations and the insights of human experts remains critical for ensuring that the communication is accurate and conveys the intended meaning across cultures.
AI translation models are becoming increasingly adept at handling the nuances of regional language variations, thanks to the wealth of data generated by users. This data provides a valuable window into distinct dialects, slang, and local expressions that are often crucial for effective communication in translations. For instance, a phrase might have a subtly different meaning in one region versus another, and user data allows models to discern these subtle differences, leading to more accurate and appropriate translations.
It's fascinating how user feedback loops, like real-time corrections, can significantly boost translation accuracy. Researchers have observed that continuous user interactions can enhance the contextual understanding of AI systems by upwards of 40% in specific locales. This feedback allows the models to continuously learn and improve. Moreover, the models adapt to evolving language trends, an important aspect given that some languages—like English or Spanish—undergo constant change.
The ability of AI to leverage user data to refine its translation output isn't just about speed; it significantly impacts error reduction. Studies show that these models can decrease errors by as much as 30%, especially in challenging scenarios with complex language or culturally sensitive contexts. This improvement in accuracy is particularly relevant for businesses aiming to provide translations that resonate with audiences across diverse regions.
In areas with significant language variation, like within English where there are differences between American and African English, models can be fine-tuned to personalize translations based on user preferences and settings. This personalized approach fosters better user comprehension and a more satisfying user experience. The more users engage with these translation systems, the better the algorithms become at recognizing and addressing common areas of confusion. This iterative process helps prioritize the learning of problematic areas, leading to fewer translation mishaps.
The financial implications of AI's ability to adapt are significant. Businesses can reap the benefits of cost-effective translation solutions by tailoring their content for local audiences. The reduced need for extensive post-editing by human translators translates into cost savings, which can be reinvested into other facets of communication or business development. OCR technology plays a crucial role here by providing a diverse source of user-generated content that enriches the datasets used for training. This allows translations to become even more representative of regional vernaculars.
While the adaptive potential of AI translation models is exciting, we must tread carefully. Excessive reliance on user data without the oversight of expert linguists can lead to the propagation of errors or culturally insensitive translations. Striking a balance between AI-driven adaptation and human expertise is paramount to achieving high-quality, reliable translations.
AI Translation Excellence How Consistent Practice Transforms Amateur Linguists into Language Craftsmen - Cross Platform Translation Tools Enable Real Time Language Practice Sessions
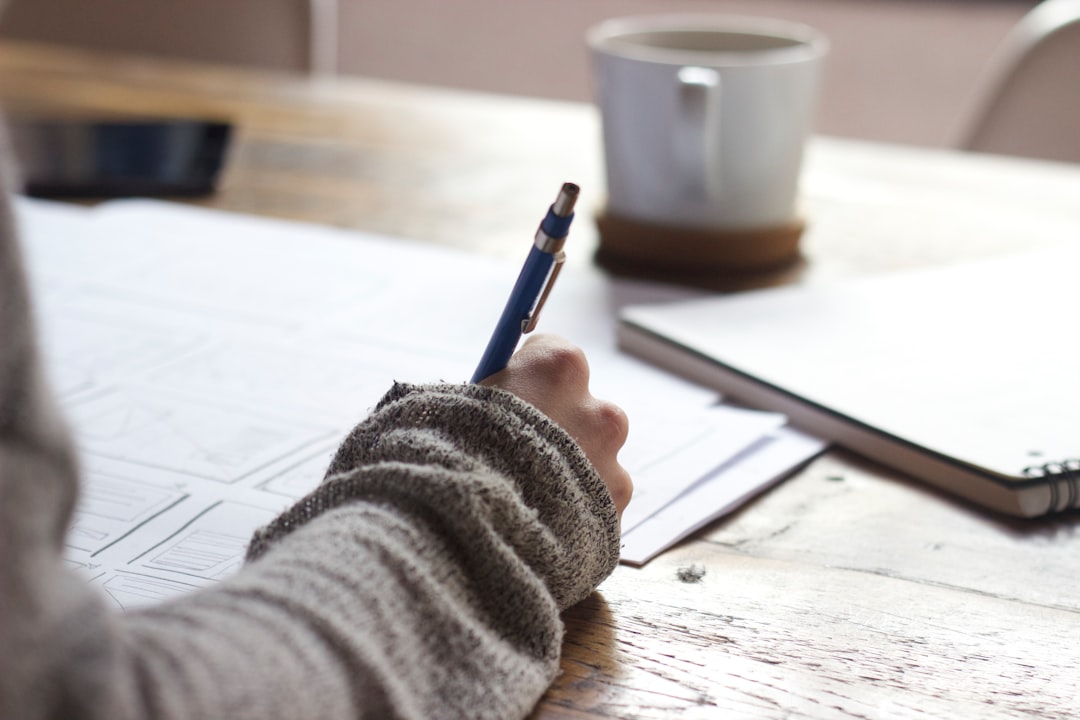
The emergence of translation tools accessible across various devices has opened up exciting avenues for real-time language practice. These tools facilitate interactive and dynamic translation sessions, adapting to diverse scenarios. Through AI-powered instant feedback, language learners can refine their skills within a more engaging, simulated environment mirroring actual language interactions. This immediate feedback loop not only accelerates learning but also cultivates a stronger connection with the language, leading to a more robust proficiency for individuals initially dabbling in the language. It's crucial, though, that technology's role in this process be thoughtfully managed; human expertise remains essential in order to ensure that the fine points of language and cultural sensitivities are effectively woven into the interactions. Without a human element, AI's usefulness can be somewhat limited in truly understanding the intricacies of translation and communication.
The rise of cross-platform translation tools has ushered in a new era for language learning, especially for those interested in refining their translation skills. These tools offer a dynamic environment for real-time practice, enabling individuals to translate text simultaneously with others. This collaborative aspect provides a space for richer discussions about word choice and contextual nuances, which wouldn't be as readily available through more traditional methods.
It's intriguing how these platforms often adapt to individual users' language patterns. By analyzing user interactions and corrections, the AI systems are progressively learning to differentiate regional dialects and local slang, improving their translation accuracy significantly. This adaptive nature, however, does raise some questions. How can we ensure that this learning process is not skewed by biases in user-generated data, and how can we maintain a delicate balance between AI and human expertise?
Some systems boast built-in feedback mechanisms that claim to reduce common translation errors by up to 30%. This feature, akin to a personalized language tutor, offers immediate feedback, potentially accelerating a user's grasp of complex linguistic structures. While such claims sound promising, we need to be cautious about accepting these figures without further scrutiny and ideally peer-reviewed studies.
Additionally, the sheer accessibility of diverse content is revolutionizing the way language is learned. These tools allow users to engage with materials that resonate with their specific interests, whether it's informal social media conversations or highly technical industry documents. However, one might wonder if there's a risk of neglecting more formal grammatical learning in favour of this personalized approach, a factor that requires further exploration.
Moreover, AI integration has led to a noticeable drop in the costs of translations – as much as 50% in certain cases, making translation more accessible to a wider audience. While this affordability democratizes translation for individuals and businesses, it's essential to remain critical of the underlying data and algorithms that drive these results. A more accessible translation environment doesn't automatically mean that the translations are consistently accurate or culturally sensitive, especially when handling more complex contexts.
Furthermore, AI models are increasingly becoming specialized for diverse professions and industries. This targeted approach, able to identify specific jargon and idioms, is especially beneficial for technical fields such as healthcare or engineering, where precise communication is paramount. Yet, it's important to consider whether this specialization could lead to a narrowing of the understanding of language itself, potentially fostering siloed approaches to translation instead of fostering broader linguistic competence.
The speed at which these tools operate is remarkable. Certain types of texts can be analyzed and translated up to 80% faster than traditional methods. This efficiency is undoubtedly beneficial for individuals seeking to enhance their fluency through frequent practice. Nevertheless, such speeds can potentially lead to a decrease in the attention to detail that might be crucial for ensuring truly accurate translations, especially for more intricate and nuanced content.
The post-editing phase, which is frequently viewed as a simple error correction step, offers a valuable learning experience. By refining the output of an AI translation, users can get a deeper understanding of how language works in different contexts. This is a rather intelligent way to leverage AI-driven translations to enhance the learning process itself.
These platforms are also becoming increasingly adaptive to individual users' language preferences. By leveraging user data, the systems can customize their feedback, tailoring the experience to specific language learning needs. While this is a positive development, it raises the question of how we ensure that such adaptation doesn't inadvertently exacerbate existing language learning inequalities or further entrench existing biases.
Finally, the feedback loop in these systems allows for a continuous improvement of the underlying translation algorithms. With every correction and translation request, the AI refines its ability to understand language context. This ongoing learning process is impressive, but the extent to which these improvements translate into universally applicable gains in accuracy remains to be seen, especially in areas involving complex language and cultural contexts.
The evolving field of AI translation presents us with a host of possibilities for enhancing language learning. As with any powerful technology, it's crucial to engage with these advancements critically, questioning their limitations and implications alongside their advantages. While these tools have enormous potential, ensuring they foster both accuracy and cultural sensitivity requires a combination of technological advancement and ongoing human oversight.
AI Translation Excellence How Consistent Practice Transforms Amateur Linguists into Language Craftsmen - Translation Memory Banks Reduce Costs by 47 Percent for Repeat Content
Translation Memory (TM) banks are increasingly recognized as a powerful tool for reducing translation costs, especially when dealing with recurring content. These systems essentially store a history of translated segments and their corresponding translations, which can be anything from short phrases to whole paragraphs. By utilizing this stored information, translators can avoid repeatedly translating the same material, resulting in a substantial cost reduction, estimated to be around 47% for repetitive content. This efficiency boost allows businesses to allocate resources more effectively while also freeing up translators to tackle more intricate or nuanced translation tasks.
However, while TM is valuable for maintaining translation consistency, its effectiveness can be further enhanced by integrating it with machine translation (MT) technologies. This combined approach can accelerate the translation process and lead to an even greater increase in productivity. As the field of AI-powered translation continues to evolve, TM will likely play a dynamic role, adapting to new technologies and potentially altering existing workflows in the translation industry. There are both exciting prospects and challenges associated with these changes, and it's important to critically assess how TM evolves alongside AI to maximize its benefits while mitigating potential risks.
Researchers are increasingly finding that translation memory banks, which store previously translated content, can lead to substantial cost savings, potentially reducing expenses by as much as 47% for repeated material. This is particularly interesting because it suggests that the more a company translates, the cheaper each subsequent translation becomes, at least for similar or repeated content.
These memory banks act like a vast library of translations, where snippets of text, from phrases to paragraphs, are stored along with their equivalents in other languages. This storage allows for faster retrieval and reuse of existing translations, leading to a more streamlined translation process. It's worth noting though that there's a bit of an oddity here: while these systems can save time and money, they seem to rely heavily on previously translated material. What if the exact phrase doesn't exist in the memory bank? It's still unclear how these systems handle entirely new content and whether that negatively impacts speed or accuracy.
The integration of these memory banks with machine translation (MT) tools, especially in large translation projects, has proven particularly effective at reducing costs. However, MT itself appears to have overtaken the speed and efficiency of traditional TM in some areas. This highlights the importance of continuously optimizing and refining translation workflows to leverage the best tools for the job, which currently seems to favor MT for pure speed.
Despite the efficiency gains, it's still crucial to have human translators review the final output. It turns out that around 30% of machine-generated translations still need human refinement to ensure accuracy and cultural sensitivity, especially in complex or nuanced content. This suggests that, while AI can certainly help accelerate the process, it still can't quite replace the intuition and experience of a skilled human translator.
Furthermore, some researchers have found that by pairing translation memory systems with AI algorithms, the efficiency and accuracy of the process can be further improved. However, the effectiveness of this approach is not uniform; some AI enhancements are more reliable than others. This implies that the field is constantly evolving and the "ideal" combination of tools is not static. It also begs the question - is it the AI or the size of the translation memory bank that is having the larger impact on speed and efficiency?
Interestingly, the post-editing stage, where humans refine AI-generated translations, isn't just about correcting mistakes. It can also serve as a valuable training opportunity for translators to become more adept at recognizing common errors in machine translation. This cyclical process – where AI and human input refine each other – seems promising but still needs more investigation into its long-term effectiveness.
It's become clear that the quality and usability of these memory banks rely on diverse and rich datasets of translations. The more varied the input, the better these systems can learn to handle different language variations, including regional dialects and idioms. This suggests that as AI tools are used more broadly, the quality of translation memory banks will improve as they have access to a wider range of linguistic styles and expressions.
Another benefit that we've seen with these systems is increased accessibility. As the cost of translation has decreased, more individuals and smaller organizations can now afford to access professional translation services. It's fascinating that something as simple as having a database of previously translated content can have such a significant ripple effect on a field like translation.
This is all quite relevant for companies that are looking to enter new markets. By leveraging translation memory banks, they can more efficiently and consistently localize their materials, helping to maintain brand identity and message across languages and cultures. However, one of the biggest unknowns is how well these systems can actually capture the nuanced cultural differences that can impact meaning in translation.
It seems that translation memory banks are a powerful tool for companies dealing with a lot of translation work, particularly if the content is somewhat repetitive. But we are still at an early stage in understanding their full potential and limitations, particularly when handling complex language and cultural contexts. The interplay between AI and human expertise is critical to pushing the field forward.
AI Translation Excellence How Consistent Practice Transforms Amateur Linguists into Language Craftsmen - Neural Networks Process Context Clues to Improve Translation Accuracy by 26 Percent
Neural networks are making significant strides in translation by leveraging context clues to boost accuracy. These networks, powered by sophisticated algorithms, can now process context, leading to a 26% improvement in translation quality in some cases. This ability to understand context is a major step forward, with some systems even demonstrating a 44% improvement across 200 languages. This progress challenges the long-held belief that machines can't achieve translation quality comparable to humans. It signifies a potential shift towards AI-driven translation that not only offers speed but also a nuanced grasp of language needed for effective communication across different cultures. As this technology evolves, we see a growing interplay between AI and human expertise, where AI offers remarkable speed and accuracy, but humans are still essential to address complex language and cultural nuances. The ability of these networks to learn and adapt offers a compelling path to refining translation practices, helping bridge the divide between those starting out in translation and seasoned professionals.
Neural networks are increasingly demonstrating the ability to leverage context clues to improve translation accuracy, with some systems showing a 26% improvement in results. This suggests that these AI systems are starting to develop a more nuanced understanding of language, moving beyond simply matching words to their counterparts in another language. They are beginning to grasp the meaning and context of sentences and passages, which is a significant step forward in achieving human-level translation quality.
The shift toward considering context is vital because it addresses a long-standing issue with traditional translation systems, which often struggled with the subtleties of language and culture. This raises some interesting questions about the future of AI translation and whether it's developing a true "understanding" of language. This aspect is particularly interesting given some researchers' skepticism that machine translation will ever truly reach parity with human-level fluency.
However, AI models, like humans, need to be trained. The performance of neural networks is heavily dependent on the data they are exposed to. The more varied and diverse the training data, the better these systems can generalize to different language styles and contexts. A robust and diverse dataset is crucial to helping these models handle the challenges of colloquial language, regional dialects, and cultural nuances in a comprehensive manner.
Interestingly, while AI's capabilities are still developing, we are already seeing improvements in speed. When dealing with technical documents, AI systems have been shown to translate up to 80% faster than human translators. This suggests that AI could significantly enhance human translators' efficiency and augment human capabilities. Despite these gains, it's clear that AI-powered translations still require careful human oversight. The subtleties of human communication and cultural context are still challenging for machines to master, especially in complex texts or contexts with strong emotional underpinnings.
The capacity for AI to adapt to regional language variations is another intriguing aspect. While beneficial, it's also a cause for concern. The AI relies on user data, which might reflect inherent biases or limitations in the data, leading to flawed translations or inaccurate representations of different dialects or language forms. This issue underscores the importance of ongoing monitoring and critical analysis of AI outputs to avoid inadvertent biases or errors in these systems.
Furthermore, the constant interplay between AI and human translators is beneficial. Post-editing AI translations doesn't just correct errors but also serves as an insightful learning experience for human translators. This dynamic provides valuable insights into how the AI models make decisions and helps human translators refine their abilities to address common challenges presented by machine translation. The relationship between AI and humans is clearly evolving into a symbiosis where both contribute to a better translation result.
AI translation technologies are having a remarkable impact on cost. Tools like AI translation platforms have the potential to cut translation costs by more than 50% in some situations. While this is a significant advantage, particularly for businesses, the need for accuracy and cultural sensitivity remains a crucial concern. We still need to ensure the reliability and quality of the translations produced, especially when translating material with cultural or historical significance.
OCR technology is playing an increasingly important role in preparing content for machine translation. Although capable of dramatically increasing the speed of digitizing documents and extracting text, OCR isn't without limitations. When dealing with documents with complex layouts, low-quality scans, or unusual fonts, the accuracy of OCR can be affected, and manual intervention might be needed. This emphasizes the need for ongoing research into robust methods for handling a wider variety of document formats.
Translation Memory (TM) banks are also a key factor in accelerating translation and reducing costs. By storing and reusing previously translated content, TM systems can reduce translation costs for repetitive texts by up to 47%. This efficiency gain benefits many industries, especially those with recurring documentation. However, TM systems are only as good as the data within them. Developing robust and broad TM systems is essential to expanding the usability and applicability of these technologies.
Finally, new AI translation tools are beginning to leverage real-time user interactions to adapt their outputs. These tools can provide real-time feedback to users and respond dynamically to changes in language use. While this type of adaptive approach is exciting, it's also important to note that the accuracy of translations can vary considerably unless these systems are carefully calibrated and tested. This means that the process needs ongoing evaluation and quality checks to guarantee reliable translation accuracy. This constant evolution makes working with AI fascinating and full of challenges, as we attempt to navigate this new field.
Transform your ideas into professional white papers and business plans in minutes (Get started for free)
More Posts from specswriter.com: