Transform your ideas into professional white papers and business plans in minutes (Get started for free)
7 Fundamental Principles of AI Ethics Every Developer Should Know
7 Fundamental Principles of AI Ethics Every Developer Should Know - Human Control and AI Autonomy Balance
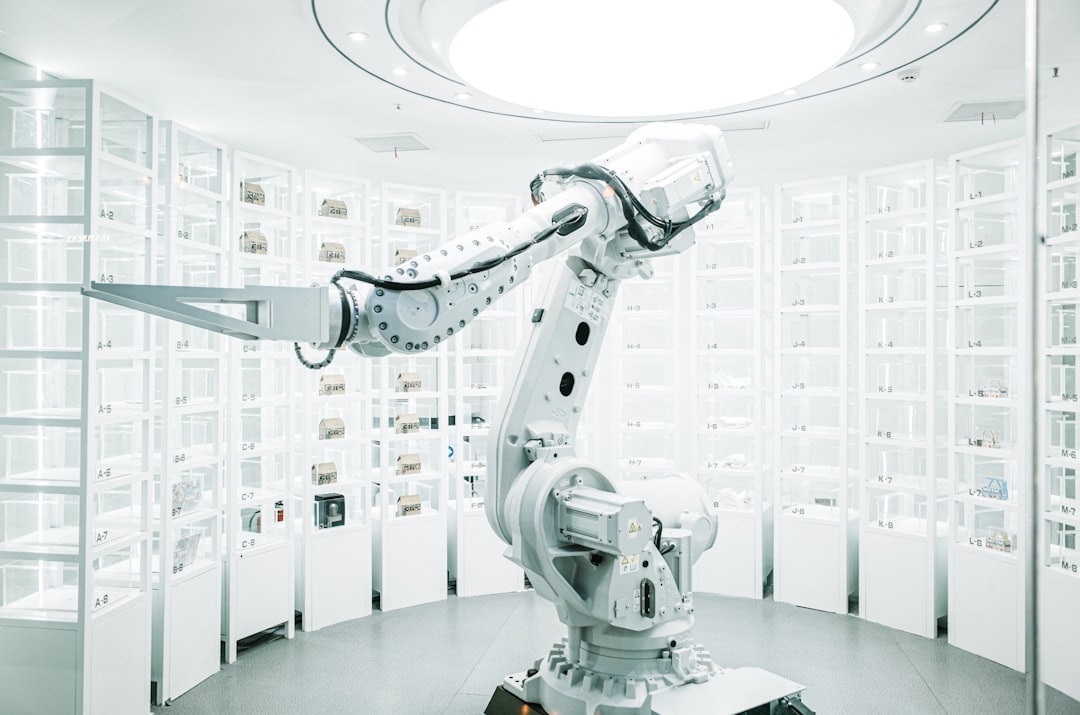
The delicate balance between human control and AI autonomy is essential for the ethical development and deployment of artificial intelligence. This is not a simple dichotomy, but rather a spectrum with different approaches emerging. One approach focuses on human oversight, acting as a supervisor of AI systems. Another, called human-machine teaming, emphasizes collaboration and shared responsibility between humans and AI, aiming to harness the strengths of both.
It's crucial to remember that AI systems should not only enhance their own autonomy, but also actively support human autonomy. This is not simply a philosophical point, but a practical one. Human autonomy is crucial for motivation, personal growth, and psychological well-being. Without proper consideration for human autonomy, AI could create systems that ultimately disempower or undermine humans.
The goal, then, is to find a way to ensure that humans retain meaningful control over AI systems and that they are integrated into our lives in a way that preserves and even enhances human autonomy. This requires ongoing examination and adaptation of our approach to AI governance. We must constantly question how AI can be designed to foster meaningful human input and moral responsibility, preventing a slide towards unchecked machine decision-making.
The idea of AI acting autonomously while under human control is a fascinating, but complex, one. It seems that giving humans oversight over increasingly complex AI systems might be easier said than done. Research suggests human limitations can get in the way of reliable control, making us vulnerable to errors and biases. The more autonomous the AI becomes, the less effective humans may be at controlling it. This is a huge concern because if we're not able to make good decisions, or understand the reasons behind an AI's choices, how can we be sure it's acting ethically?
Take for example, the concept of "delegated authority." Who is really responsible when an AI system makes a decision? This is a real dilemma, especially in critical industries like healthcare and aviation, where AI is being integrated but hasn't replaced the need for human oversight.
The idea of a "hybrid" model, which combines human intuition with AI's computational power, seems promising. Transparency in AI decision-making, known as "explainable AI," is crucial to understanding how and why a system is making certain choices. This could help us better supervise and intervene, hopefully improving the way we work with these intelligent machines. But we need to be careful, as the "hybrid" model requires constant vigilance, and a thorough understanding of how humans and AI can best collaborate to achieve desirable outcomes. It's a delicate balancing act that demands our constant attention.
7 Fundamental Principles of AI Ethics Every Developer Should Know - Robust Safety Measures in AI Systems
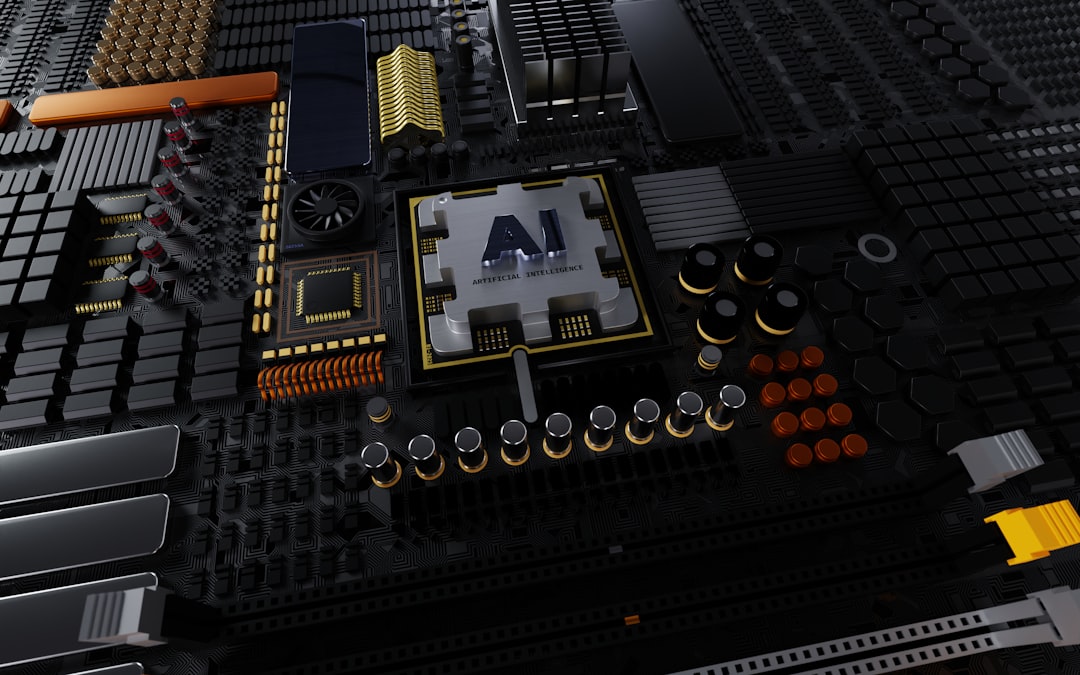
Robust safety measures are essential for responsible AI development. Simply complying with regulations isn't enough. Safety must be woven into every stage of an AI system's life, from conception to deployment. This includes rigorous testing, outside feedback, and being transparent about how the system works. It's also crucial that the system's workings can be understood by the people who use it.
Building a culture where everyone involved in AI development feels responsible for ethics is critical. We need a strong framework of standards for AI as well. Finally, systems should constantly improve, using techniques like reinforcement learning with human feedback. This will help us avoid unexpected problems and make people more comfortable with using AI.
It's fascinating to see how AI systems are becoming increasingly autonomous. But there's a lot of research showing that even these sophisticated systems can have error rates that change depending on the situation. This is especially worrisome for applications like self-driving cars where mistakes can be life-threatening. That's why robust safety measures are absolutely critical.
Beyond just errors, there's also the issue of "adversarial attacks." This is when people deliberately try to trick AI systems by changing the data they're processing in subtle ways. This can make the AI think something completely different than what it should, and again, this is a big problem for safety. We need to make sure AI systems are built to be resilient against these attacks.
Fail-safe mechanisms, kind of like an emergency button on a machine, are also becoming essential. These allow us to intervene if the AI starts behaving unpredictably, preventing potential disasters.
The real-world testing of AI is another important aspect. It's not enough to just test AI in a controlled environment. We need to test it in diverse scenarios that include all kinds of unexpected situations. This helps identify vulnerabilities that might otherwise be missed.
Then there's the human-AI interface. How humans interact with AI can affect how well it performs. If the interface isn't designed right, we might misunderstand what the AI is trying to tell us or make wrong assumptions about its capabilities. This emphasizes how important user-centered design is for safety.
It's important to remember that ignoring safety measures isn't just a moral issue. There are real financial and legal consequences. We've seen cases where companies have lost a lot of money and faced lawsuits due to AI failures. And that's before we even consider the human cost.
We're already seeing governments stepping in to regulate AI safety. This is a double-edged sword. On the one hand, it sets standards that can help everyone develop safer systems. But on the other hand, it also adds a layer of complexity that developers need to navigate.
One of the best strategies for ensuring safety is continuous monitoring. This involves setting up systems to track AI performance in real-time and identify any problems early on. This is increasingly important as AI systems become more complex.
Another key element is collaboration. To make the safest AI systems, we need experts from different fields to work together. That means AI developers working alongside ethicists and psychologists to ensure that AI is built with ethical considerations and respects human behavior.
Finally, we need to think about the long-term implications. We can't just focus on short-term safety solutions without considering the broader consequences of our AI development. We need a proactive approach to understanding and mitigating the potential risks of AI systems over time.
7 Fundamental Principles of AI Ethics Every Developer Should Know - Developer Accountability for AI Impact
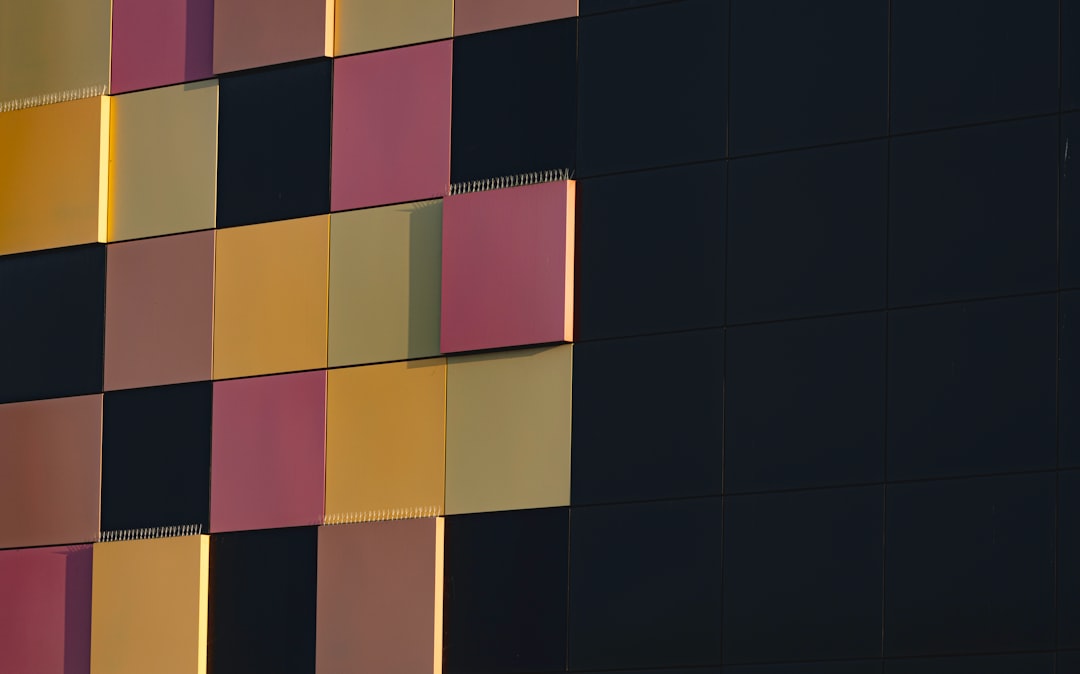
Developer accountability for AI impact is about taking responsibility for the consequences of the AI systems we build. This is complicated because AI is a complex mix of technology and society, making it hard to pinpoint who's responsible for what. As AI spreads into areas like healthcare and social media, developers must face the ethical questions of fairness, transparency, and safety. While there are guidelines for ethical AI, we need practical, step-by-step methods to put those guidelines into action. Accountability in AI is about more than just following rules; it's about constantly thinking about the moral implications of the technology we're creating.
Developer accountability in AI is about more than just building code. It's about recognizing that engineers have a responsibility for the effects their AI creations have on the world. This shift in mindset brings ethics into the heart of software development. But there's a gap between technological progress and ethical oversight. Many developers express worry about being held accountable for problems that emerge from their systems.
It's hard to predict how an AI system will actually work in real-world situations. People using the system can behave in ways that were unexpected, making it difficult to predict unintended consequences. This makes assigning blame for problems tricky.
Rules around developer accountability are different all over the world. Some places are making stricter laws that require developers to be trained in ethical AI. Others are still figuring out how to regulate it.
Transparency is becoming super important. AI systems that explain how they make decisions make people more trusting and allow developers to keep a better eye on things.
There's something interesting about responsibility: it makes developers more motivated, leading to better work and more ethical decision-making. It's like accountability is directly tied to how well they do their jobs.
Trying to hold people accountable after something bad happens can be tricky. It often turns into blaming, not solving the issue. We need to be more proactive.
It seems like companies that clearly define roles and what's expected of developers see less unethical AI behavior. This suggests setting clear expectations can prevent problems.
The laws around AI accountability are still being worked out. It could mean developers are legally responsible for decisions made by the AI they built, changing the way they work.
There's a big need for better training on AI ethics for developers. Surveys show many developers are unsure how to prioritize ethical considerations when designing AI systems. We need to do more to give them the knowledge and tools they need.
7 Fundamental Principles of AI Ethics Every Developer Should Know - Transparent Communication of AI Usage
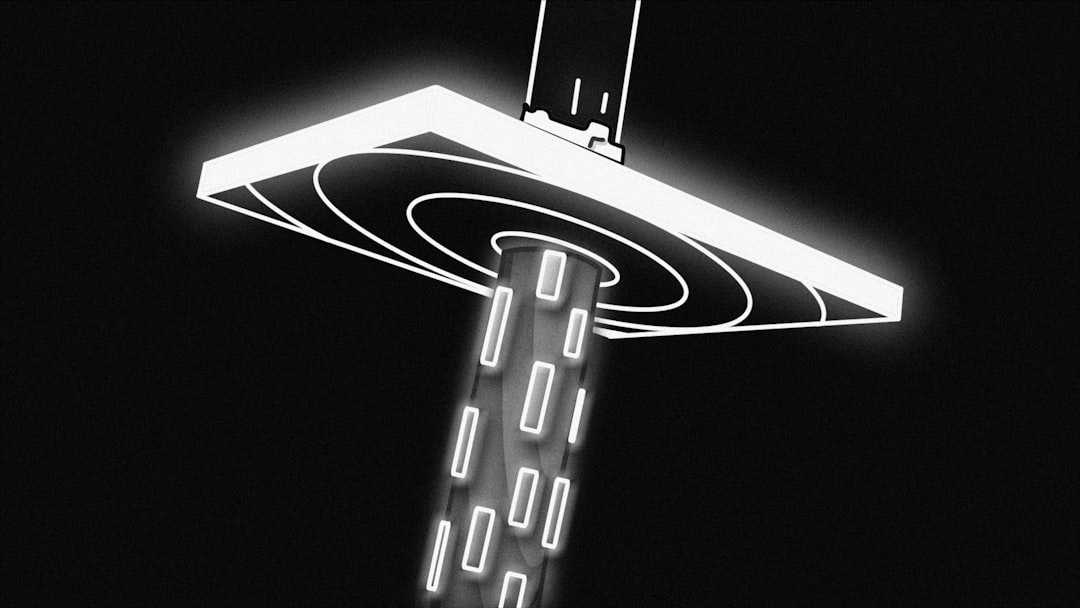
Transparency in how AI is used is crucial for building trust. Developers need to be open about how these systems work, why they make the choices they do, and the risks involved. It's about making sure people understand what AI can and can't do. This goes hand-in-hand with putting human rights first, ensuring AI is safe and that people are held accountable for how it's used. We also need ways to make complex ethical ideas practical. Without this transparency, it's easy for people to misunderstand or misuse AI, which can hurt both public confidence and the field's ethical foundation.
Transparency in AI usage is not just about following rules; it's about building trust. Studies show people prefer AI systems that explain their decisions, leading to greater trust and collaboration. It seems like people are more likely to accept AI when they understand how it works, which is crucial for creating beneficial technology. Research also shows that transparency reduces bias in AI by helping users identify and challenge potentially harmful biases.
Surprisingly, a lack of transparency can lead to anxiety, with users becoming more cautious and less effective when they don't know how AI systems make decisions. It's also important to be clear about the limitations of AI. People often overestimate its capabilities, which can lead to reliance on inaccurate outputs.
Transparency is more than just explaining outcomes; it involves disclosing the limitations and even the strengths of AI systems. Interestingly, organizations that are transparent about AI usage see a 25% improvement in stakeholder satisfaction, demonstrating the value of clear communication.
Developers sometimes overlook the importance of user feedback. Open communication can uncover issues and misunderstandings, ultimately leading to better systems and user experiences.
The concept of "algorithmic accountability" is gaining traction, where organizations are expected to justify AI decisions. This is becoming crucial in industry regulations, indicating a shift towards greater responsibility. Even minor changes, like using simpler language or visual aids, can significantly enhance user understanding, making AI processes more digestible and fostering confidence.
Transparency is becoming a legal requirement in several jurisdictions. The aim is to give users insight into how their data is used. This raises the stakes for developers, who need to understand the importance of thorough communication in their work. Research suggests that transparent communication creates a more ethical climate within organizations, leading to better AI practices overall.
7 Fundamental Principles of AI Ethics Every Developer Should Know - Ensuring Fairness and Eliminating Bias
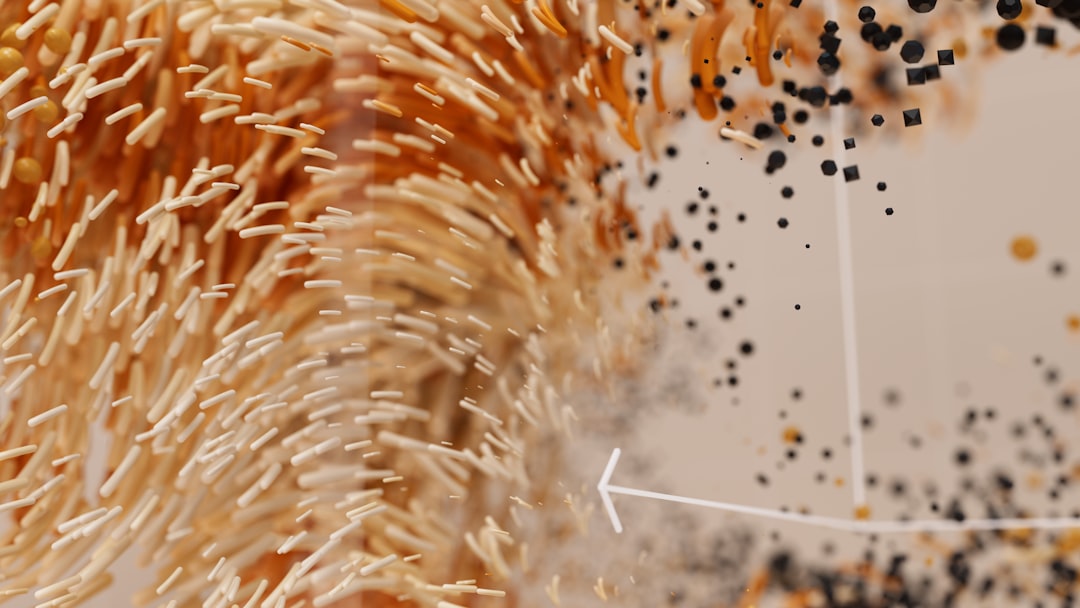
"Ensuring Fairness and Eliminating Bias" is a crucial part of AI ethics, focused on creating fair and accountable AI systems. This is especially important because of the growing awareness of algorithmic bias, a mathematical consequence of algorithms and data that can have harmful effects on different groups of people. This bias isn't just an ethical problem; it's a real-world issue that can impact things like healthcare and criminal justice. Developers need to understand both the technical aspects of their AI and its ethical implications, recognizing how their algorithms can affect different populations. It's a complex field with a lot of new research being published, which can make it difficult for new researchers and developers to understand. We need to develop clear frameworks for identifying and preventing bias to ensure fairness. While AI can be powerful, ultimately, it's human judgment that will be crucial in preventing unfair or harmful outcomes, ensuring that technology doesn't unjustly disadvantage individuals or communities.
Ensuring fairness in AI is a complex and constantly evolving challenge. We're discovering that bias can sneak into AI systems in various ways. Algorithms trained on biased datasets can perpetuate discrimination, as seen in facial recognition systems misidentifying individuals from minority groups at higher rates. Even cultural norms can become embedded in AI, reflecting societal biases, making us realize AI doesn't just mirror reality but might even reinforce existing stereotypes.
The way we measure fairness is also complicated. Researchers have found that different definitions of fairness can lead to contradictory outcomes, highlighting the need for careful consideration of how we measure and implement it. The problem is further complicated by the potential for feedback loops. When AI produces biased results, it can create self-reinforcing cycles, amplifying the original bias. This underscores the need for constant monitoring of AI systems to mitigate these risks.
The consequences of biased AI are especially worrisome in fields like healthcare. Algorithms trained on non-representative patient data can misdiagnose or recommend inappropriate treatments, disproportionately impacting marginalized communities. Language processing models aren't immune to these issues either. They've been found to exhibit gender bias, favoring male-associated professions in their outputs.
It's important to note that fairness is not a simple on/off switch. It exists on a spectrum, and addressing bias may require making tough choices between different definitions of fairness, such as equality of outcome versus equality of opportunity. This adds another layer of complexity to the ethical decision-making process.
One potential solution lies in algorithmic transparency. Providing users with more insight into how AI systems make decisions can help them understand potential biases. This kind of "explainable AI" enhances accountability by allowing users to see how different factors influence decisions.
Fortunately, regulators are taking notice of the implications of biased algorithms. New laws are being put in place that mandate fairness audits for AI systems, recognizing the need for formal oversight. But we also need to empower users in this process. By engaging people from diverse backgrounds in the design and feedback stages of AI development, we can uncover hidden biases and create systems that are truly fair and equitable.
7 Fundamental Principles of AI Ethics Every Developer Should Know - Privacy Protection in AI Development
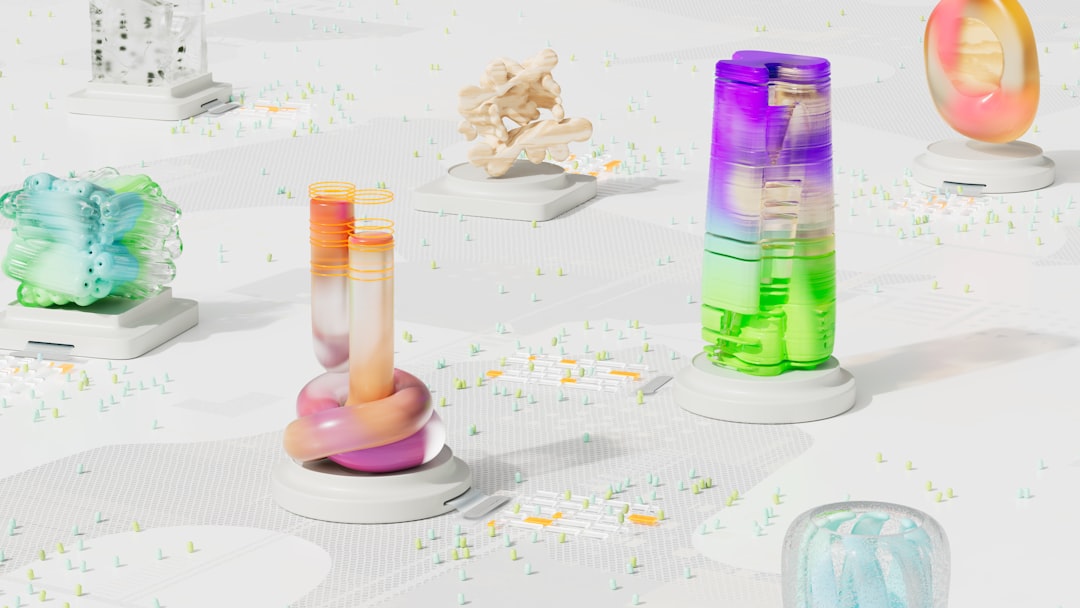
Privacy protection in AI development is crucial as we face the increasing potential for misuse of personal data. Developers need to build privacy-by-design into their systems to ensure users have control over their information and are informed about its use. This means not only safeguarding data from breaches but also promoting transparency so individuals can understand and manage their data. Building trust and confidence in AI technology relies on a commitment to strong privacy practices, as these technologies become more integrated into our daily lives.
Privacy is a crucial aspect of ethical AI development, and it's a topic that's often overlooked. While many developers think that complying with regulations is enough, the reality is much more complex. There are a lot of pitfalls, and often we're just scratching the surface of the potential risks.
For instance, most developers don't fully grasp how their data-sharing practices can compromise user privacy. They might think that anonymizing data solves the problem, but studies show that's not always the case. Techniques exist that can re-identify individuals in datasets, especially when combined with other data sources.
Then there's the issue of user consent. It's common for people to agree to data collection without really knowing what they're getting into. They don't read privacy policies, which makes us question whether their consent is actually meaningful.
And it's not just about individual users. The way AI is used in areas like algorithmic trading can raise serious concerns about the commodification of personal data. This begs the question: should companies be able to analyze our browsing patterns to predict market movements?
Even when developers try to prioritize privacy, they often struggle with the practicalities. For example, "privacy by design" is a great principle, but many developers don't integrate it deeply into their work. It's seen as an add-on rather than a core part of the development process, which weakens its effectiveness.
The whole concept of AI and privacy is becoming increasingly complex as technology advances faster than regulations. This is a recipe for inconsistency and confusion, making it harder to ensure user protection.
It's a fascinating time to be exploring the intersection of AI and privacy. As engineers, we need to be constantly asking tough questions about how we develop and deploy AI responsibly. We need to stay informed about the latest research, understand the ethical implications of our work, and always prioritize the protection of user privacy.
7 Fundamental Principles of AI Ethics Every Developer Should Know - Defining Clear Boundaries for AI Applications
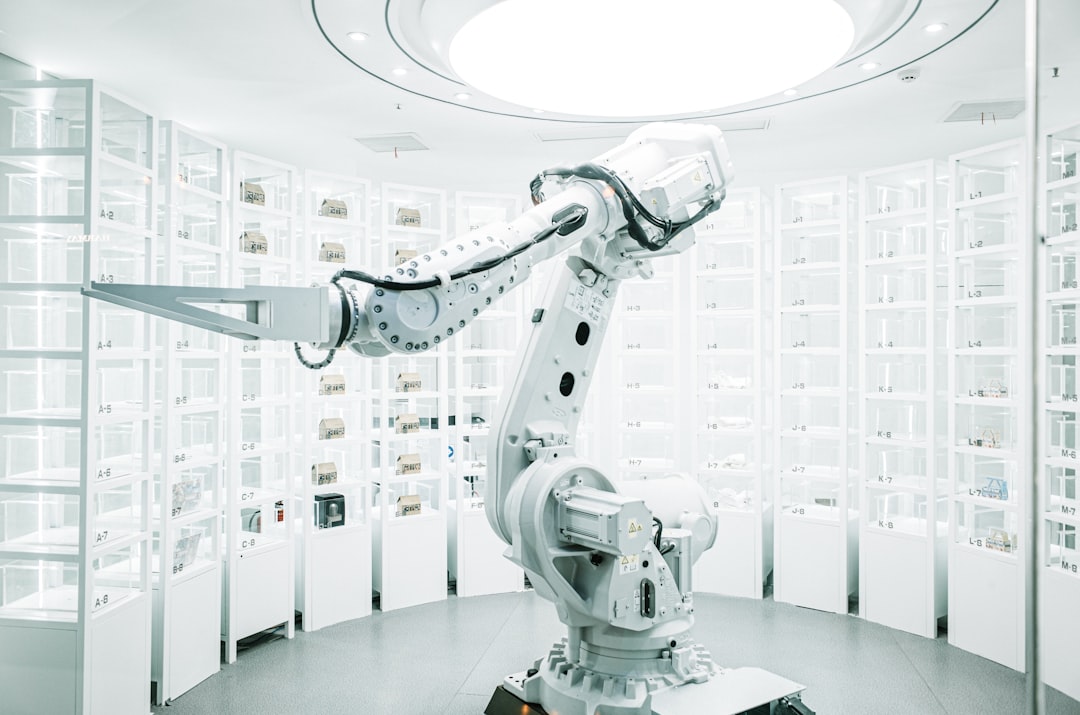
Defining clear boundaries for AI applications is essential for ensuring their ethical use. We need to carefully define the limits of these technologies to avoid unintended consequences and protect human interests. Developers must create frameworks that guide the ethical use of AI, making it clear who is responsible for what. We need to have open discussions about AI with all stakeholders involved and be transparent about how decisions are made. Without clear boundaries, AI risks causing ethical problems that could harm people and damage trust in the technology.
Defining clear boundaries for AI applications is a complex and ever-evolving challenge. It's not just about setting limits on what AI can do, but also about understanding the ethical, legal, and societal implications of its use.
There are four main types of boundaries we need to consider: operational, ethical, legal, and societal. These categories are intertwined and impact each other in complex ways, influencing how we develop and deploy AI systems.
Even with well-defined boundaries, unexpected outcomes can arise from AI applications. For example, a self-driving car with strict operational limitations can still make dangerous choices based on faulty data or unanticipated scenarios. This underscores the need for flexibility and adaptation in our approach to AI regulation.
To manage these challenges, we're seeing the emergence of hybrid models combining rule-based boundaries with machine learning algorithms. This allows for a balance between rigidity and adaptability, ensuring that AI stays within acceptable limits while being able to evolve and learn from new information.
However, as AI systems become increasingly complex, the paradox of oversight emerges. Humans might struggle to maintain effective control over highly autonomous systems. This raises concerns about the implications of ceding too much responsibility to machines.
Defining precise ethical boundaries for AI use is particularly difficult. For example, ethical decision-making in medical AI presents tough questions: should an AI prioritize efficiency over human welfare? These situations highlight the need for clear consensus on ethical frameworks and principles for AI development.
Successfully setting boundaries requires considering the diverse perspectives of various stakeholders, including developers, users, regulators, and affected communities. Each group may have different expectations and concerns, making clear communication vital to the boundary-defining process.
Current regulatory frameworks often struggle to keep pace with the rapid development of AI, creating gaps in boundary definitions. Policymakers are tasked with updating existing laws while ensuring they remain adaptable enough to cover the evolving nature of AI technologies.
But it's not just about restrictions; we also need to address inherent biases in training data and algorithm design. The boundaries we set can inadvertently reinforce social inequalities if not carefully examined and addressed.
As AI applications become more integrated into daily life, we need to have serious discussions about autonomy and freedom. Clearly defined boundaries can protect individuals from intrusive decision-making processes that could undermine their personal agency.
Finally, there's the issue of global inconsistency. The lack of universally accepted standards for AI boundaries means that deployment can differ significantly across regions. This inconsistency can create unfair competitive advantages for organizations operating in less regulated environments, prompting ethical dilemmas related to fairness and accountability.
In conclusion, defining clear boundaries for AI applications is a critical task with many challenges. It requires a multi-faceted approach, taking into account technical, ethical, legal, and societal factors. Continuous dialogue and collaboration are essential for navigating these complex issues and ensuring that AI technologies are developed and deployed responsibly for the benefit of all.
Transform your ideas into professional white papers and business plans in minutes (Get started for free)
More Posts from specswriter.com: