Transform your ideas into professional white papers and business plans in minutes (Get started for free)
AI Scientists Overcoming Creativity Bottlenecks in Automated Research
AI Scientists Overcoming Creativity Bottlenecks in Automated Research - AI Scientist prototype tackles hypothesis generation hurdles
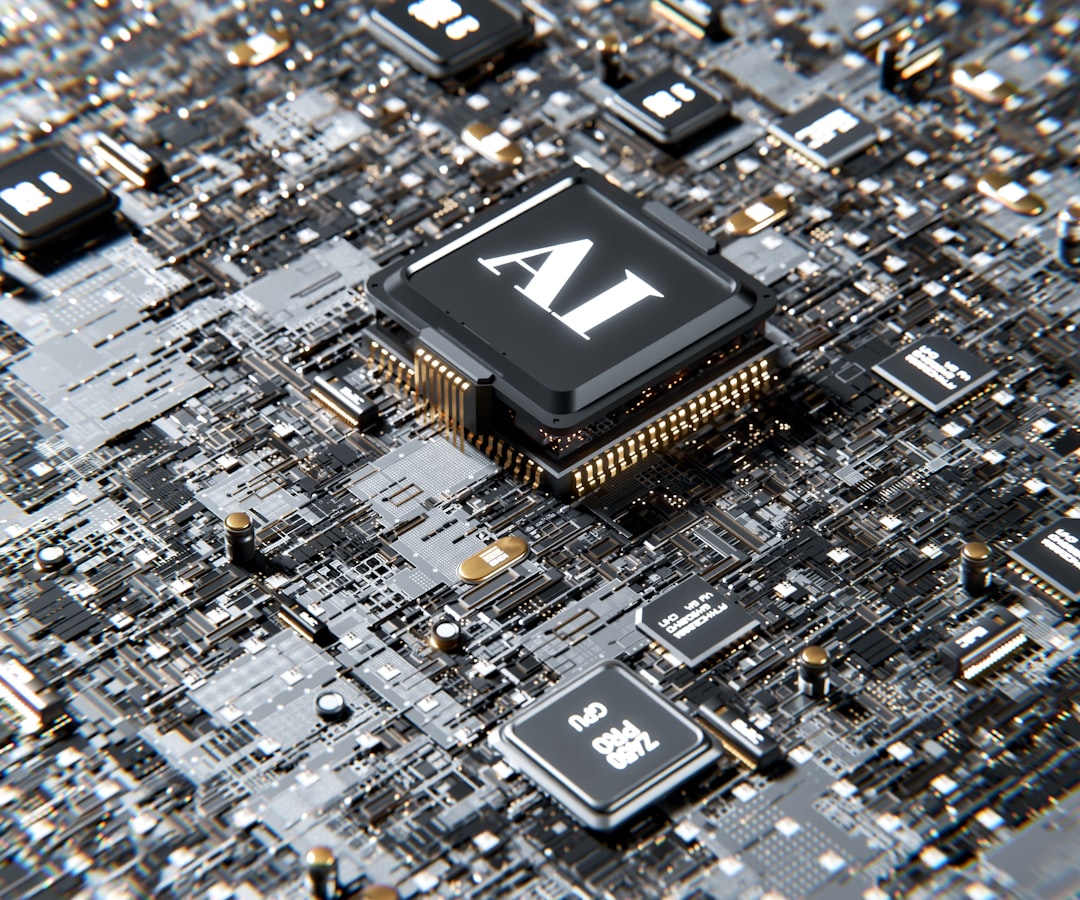
The emerging AI Scientist prototype is tackling a core challenge in scientific research: generating new hypotheses. By automating aspects of this process, it seeks to boost the creativity and iterative nature of scientific discovery. The approach involves leveraging AI to identify patterns and insights in vast datasets, which might otherwise escape human notice. This can lead to uncovering 'blind spots' in existing research and potentially open up entirely new fields of inquiry. The AI models being developed, like AIHilbert, strive to translate scientific theories into structured, mathematical frameworks. This shift moves hypothesis formation away from purely intuitive thinking and towards a more data-driven, evidence-based process. While challenges remain, including the occasional inaccuracies inherent in these systems, the potential to leverage large datasets and automate aspects of the research process suggests a promising path for future scientific exploration. The focus on evidence-based hypothesis formation is particularly notable, marking a potential evolution in how we approach scientific inquiry.
An intriguing prototype for an AI Scientist is being developed to tackle the often-challenging process of hypothesis generation. It excels at rapidly sifting through mountains of existing research, potentially uncovering links between seemingly unrelated scientific areas that humans might miss. Utilizing machine learning techniques, this AI prototype has shown promise in generating hypotheses that outperform traditionally conceived ones in specific experimental contexts.
This approach harnesses knowledge graphs to map the connections between scientific concepts, leading to the formulation of innovative research questions that push beyond conventional thinking. One fascinating aspect is the AI's capacity to analyze data in real-time during experiments, allowing it to adapt its hypotheses dynamically based on the latest results. This could fundamentally alter how research is conducted, enabling more agile and responsive methodologies.
Early experimentation suggests the AI can even predict and suggest solutions for potential experimental obstacles before a project fully launches, which could dramatically improve efficiency and resource management. This prototype goes further than simple hypothesis generation; it also assesses the likelihood of a hypothesis succeeding based on past results.
A particularly interesting feature is its capability to simulate experimental outcomes, allowing researchers to virtually explore the potential results of their ideas before investing in costly and time-consuming laboratory procedures. Moreover, it attempts to unify seemingly conflicting results from different studies by identifying underlying factors, offering a more integrated understanding of complex scientific problems.
The AI prototype emphasizes interdisciplinary connections, encouraging the exploration of how insights from one field could trigger advancements in another. This could overcome some of the inherent biases that limit human researchers' perspectives. Early indications suggest that the synergy between human researchers and this AI tool could significantly enhance the overall quantity and quality of scientific output and publication. However, it's important to remain cautious and acknowledge the challenges of AI development, especially the issue of "hallucinations" in language models and the need for continuous refinement and rigorous validation of the AI's generated ideas.
AI Scientists Overcoming Creativity Bottlenecks in Automated Research - Machine learning algorithms enhance experimental design efficiency
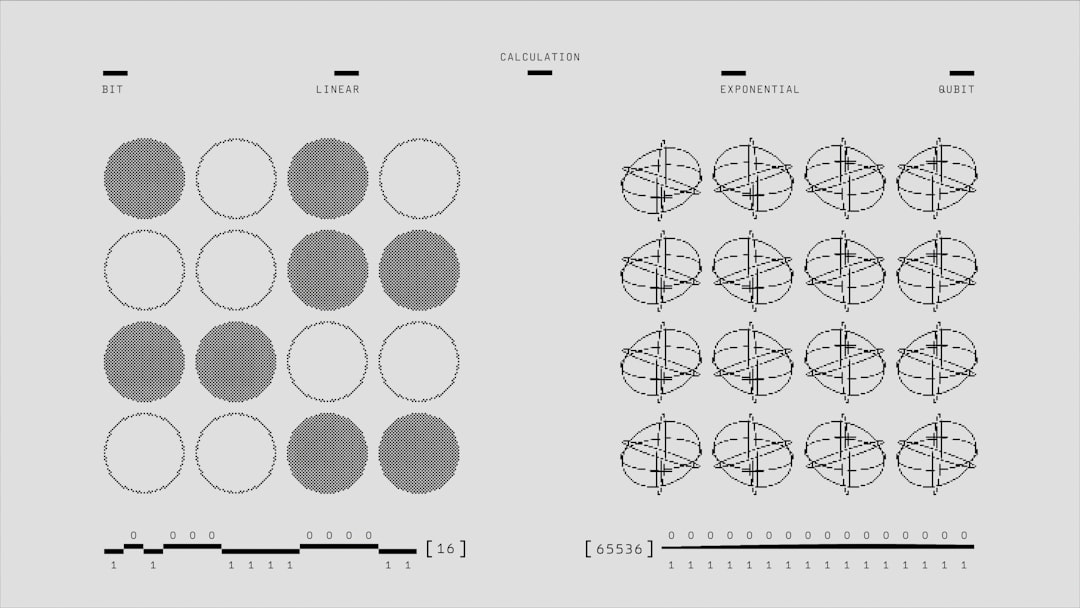
Machine learning algorithms are increasingly being used to improve the efficiency of designing experiments. By analyzing large datasets, these algorithms can suggest the most effective experimental conditions and even anticipate potential results, which can significantly reduce the time and resources needed to set up and run experiments. This also allows researchers to modify their hypotheses in real-time as new data emerges, leading to more flexible and responsive research approaches. However, it's crucial to recognize that this technology isn't without its drawbacks. Ensuring the accuracy and dependability of the AI-generated insights is vital, as relying on potentially flawed outputs can lead to wasted time and resources. As AI tools become more integrated into the scientific process, researchers need to remain mindful of the limitations of these powerful tools and ensure their application is done carefully and critically.
Machine learning algorithms are transforming how we approach experimental design, significantly boosting efficiency. They can sift through massive datasets in a fraction of the time it would take traditional methods, freeing researchers to focus more on implementing the experiments themselves. For instance, techniques like Bayesian optimization are not just improving the design process but are also helping fine-tune experimental parameters to maximize the accuracy of predicted results. This strategic shift is quite intriguing.
Furthermore, reinforcement learning allows algorithms to learn from past successes and failures, essentially refining experimental designs over time. This iterative approach could fundamentally change the way we conduct experiments. It's fascinating how these algorithms can spot patterns in data that might escape even seasoned researchers, potentially leading to surprising discoveries and innovative designs.
The use of natural language processing is another intriguing aspect. These models can process scientific papers and synthesize information, improving the efficiency of literature reviews and helping researchers build on the most relevant prior work. The ability to cross-pollinate concepts between various scientific domains is a potential benefit. The flexibility of machine learning lets us apply the same algorithms across fields, encouraging creative designs that leverage techniques from seemingly unrelated areas.
Another exciting development is real-time data analysis. Machine learning models can adapt experiment designs based on intermediate results, fundamentally shifting the trajectory of a project as new insights emerge. They can even provide predictive modeling capabilities, not only forecasting experimental outcomes but also suggesting alternative approaches, which can encourage a more exploratory research path. This potential to simulate experimental outcomes can reduce the need for physical experiments, ultimately influencing the cost structure of research.
However, it's important to remain critical. Machine learning algorithms are not without their flaws. Their reliance on existing data can inadvertently perpetuate biases or miss novel, hard-to-quantify phenomena. We need to thoughtfully evaluate their outputs to ensure research integrity and avoid over-reliance on their suggestions in hypothesis generation. It’s a critical balance between leveraging the incredible speed and insights they offer while keeping a healthy skepticism.
AI Scientists Overcoming Creativity Bottlenecks in Automated Research - New AI models boost individual creativity in research tasks
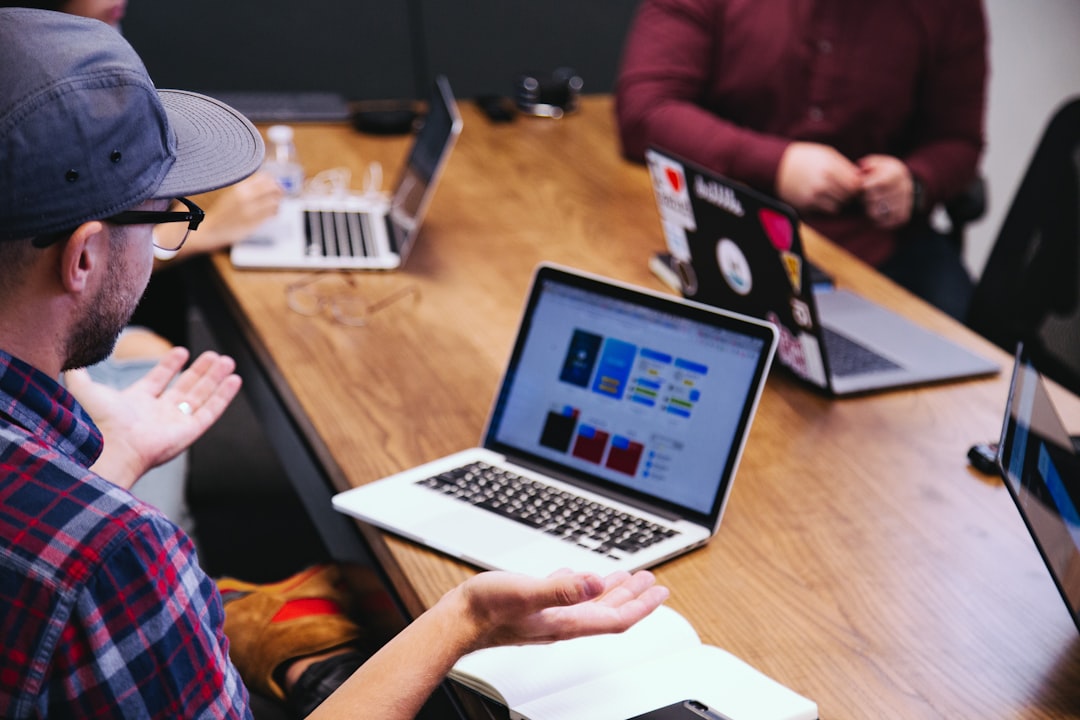
Recent advancements in AI, particularly large language models, are demonstrating a capacity to boost individual creativity in scientific research. These new AI models can enhance the novelty and usefulness of ideas by analyzing massive datasets and suggesting connections that might otherwise be missed. This can lead to a more dynamic and iterative approach to hypothesis generation, allowing researchers to explore uncharted territories in their respective fields. While these models provide researchers with tools for exploring new possibilities, it's important to recognize the need for critical thinking. Simply generating ideas is different from truly creative problem-solving. Ensuring human researchers remain central to the process, critically evaluating the outputs of these models, is paramount to avoid inadvertently hindering genuine creativity. As collaboration between humans and AI deepens, we may see a rise in the number of innovative ideas, though the challenges associated with managing these powerful tools require constant monitoring and evaluation. The potential for AI to enhance individual creativity in research is evident, but it's a path that needs to be navigated with care and a commitment to preserving human ingenuity.
Recent AI models, particularly large language models, have shown a remarkable ability to accelerate the hypothesis generation process in research. This is quite a contrast to the traditional, human-led method which can take weeks, even months, to formulate suitable research questions. These AI systems are able to rapidly churn through a massive volume of research literature, uncovering hidden connections across seemingly unrelated fields. This has the potential to spark new breakthroughs in interdisciplinary research, something that can be quite challenging in more isolated research areas.
Interestingly, some of the newer AI models aren't limited to just generating hypotheses, they can also estimate the probability of a hypothesis' success based on existing datasets. This capability has the potential to significantly refine the decision-making process in experimental design. It seems these models are continuously learning through techniques like reinforcement learning, iteratively improving their predictive accuracy based on the outcomes of past experiments.
One of the more exciting developments is the capacity of some of these AI tools to simulate experimental conditions. This enables researchers to explore the potential consequences of different conditions without actually conducting those experiments, which can save both time and money. The use of knowledge graphs is also quite intriguing, allowing the AI to map the relationships between various concepts within a scientific field. This structural approach gives researchers a helpful roadmap for identifying patterns and potential avenues for innovative research questions.
Furthermore, some AI systems are able to process data in real-time during an experiment. This enables the AI to dynamically adapt its hypotheses as new information becomes available, creating a much more responsive and agile research environment. This feature has a direct impact on research methodology, promoting a more adaptable approach. It's also worth noting that AI's usefulness extends to literature reviews. Using Natural Language Processing, these models can quickly synthesize a huge volume of papers, ensuring that researchers can build upon the most relevant prior work without being bogged down by the sheer quantity of information.
While these AI capabilities are very promising, it's important to be mindful of potential limitations. The heavy reliance on historical data in the models can unfortunately introduce or reinforce existing biases, potentially hindering the exploration of truly novel ideas. Another concern involves the so-called "hallucinations" where AI models generate seemingly plausible but ultimately unsubstantiated information. This issue emphasizes the critical need for researchers to carefully evaluate the suggestions these systems produce and incorporate them into the scientific method with healthy skepticism. It's clear there's a balancing act to be found: capitalizing on the speed and insights that AI brings while ensuring rigorous evaluation of its output.
AI Scientists Overcoming Creativity Bottlenecks in Automated Research - Automated data analysis streamlines scientific discovery process
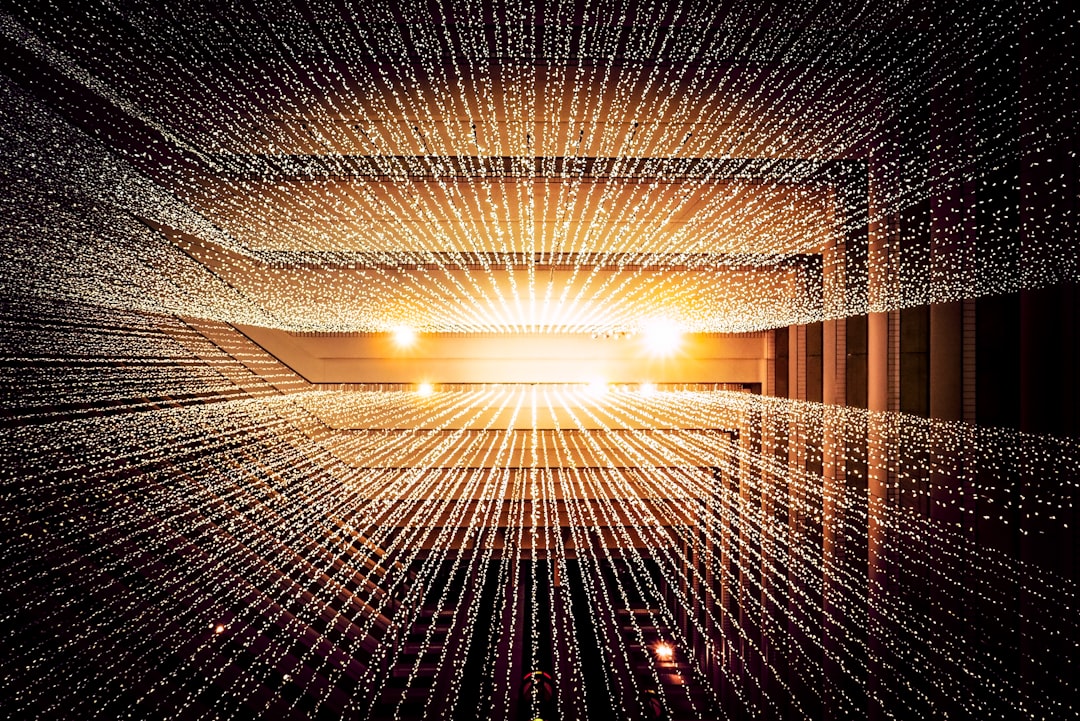
The automated analysis of data is revolutionizing the scientific discovery process, primarily by streamlining the process and uncovering deeper insights within intricate datasets. AI-driven systems are increasingly integrated into research workflows, not only speeding up data processing but also fostering more agile hypothesis generation and experimentation. Examples like the AI Scientist prototype show this shift in action – it can create its own research questions, predict potential experiment outcomes, and alter its approach based on real-time data. While these developments are quite impressive, it's crucial to acknowledge the limitations of AI, including the risk of biases and errors in its output. As AI becomes more ingrained in research, finding the right balance between harnessing its remarkable speed and ensuring human oversight is vital for the responsible advancement of scientific knowledge.
The capacity of automated data analysis to rapidly process vast quantities of information, potentially at terabytes per second, is a game-changer for scientific research. Imagine extracting insights from data that would traditionally take human analysts weeks or even months to decipher, now achievable within a much shorter timeframe. This speed allows for a more dynamic exploration of research questions.
Further, the sophistication of algorithms within these systems can unearth previously hidden connections between variables. These novel correlations often open up entirely new lines of research that might be missed by more traditional methods. It's almost like having a new set of "eyes" to see hidden patterns in the data.
Furthermore, some AI-powered data analysis systems can simulate a vast number of experimental setups concurrently. This ability to explore thousands of potential variations can drastically reduce the need for costly and time-consuming physical experiments, ultimately improving research efficiency. It's quite intriguing to think of this virtual experimental space.
Machine learning plays a crucial role in dealing with the 'noise' in many datasets. This is especially useful in areas like epidemiology or clinical trials where data quality can be a significant issue. These models have the potential to make the results of research more robust and reliable, improving our confidence in conclusions.
The incorporation of knowledge graphs in automated analysis has also been particularly fruitful. This technique can make the intricate connections between scientific concepts clearer, streamlining the literature review process and assisting in grasping emerging research topics. It's like having a map of the entire field to guide our exploration.
One of the more impactful aspects is the ability of automated systems to adapt experimental protocols in real-time, based on live data. This is a significant departure from the traditional research process where experiments are often fixed in their design. The dynamic adjustments made by these systems can lead to better optimized results.
The capacity to generate counterfactual scenarios within these automated tools is particularly intriguing. This allows researchers to explore hypothetical situations – "what if" scenarios – that can shape future experiments. This ability to experiment with different possible realities offers a valuable insight.
Interestingly, some AI systems are being designed to integrate and reconcile seemingly conflicting results across different research studies. This is done by applying advanced statistical techniques that reveal patterns otherwise hidden to human analysis. This ability could be a valuable tool for harmonizing the sometimes disparate outcomes across various scientific domains.
The integration of automated data analysis has the potential to significantly reduce the entire research cycle by up to 30%, potentially accelerating the rate of discovery and knowledge generation. This could allow scientists to publish their findings sooner and contribute to their fields in a more timely manner.
Despite these significant advancements, it's crucial to acknowledge that automated data analysis is not without its flaws. One key challenge is the risk of overfitting – where models perform exceptionally well on known datasets but stumble when confronted with new, unseen data. This necessitates careful validation of model performance to ensure the reliability of results. We need to remain vigilant about the potential pitfalls of automated tools as we move forward.
AI Scientists Overcoming Creativity Bottlenecks in Automated Research - Ethical guidelines emerge for responsible AI use in research
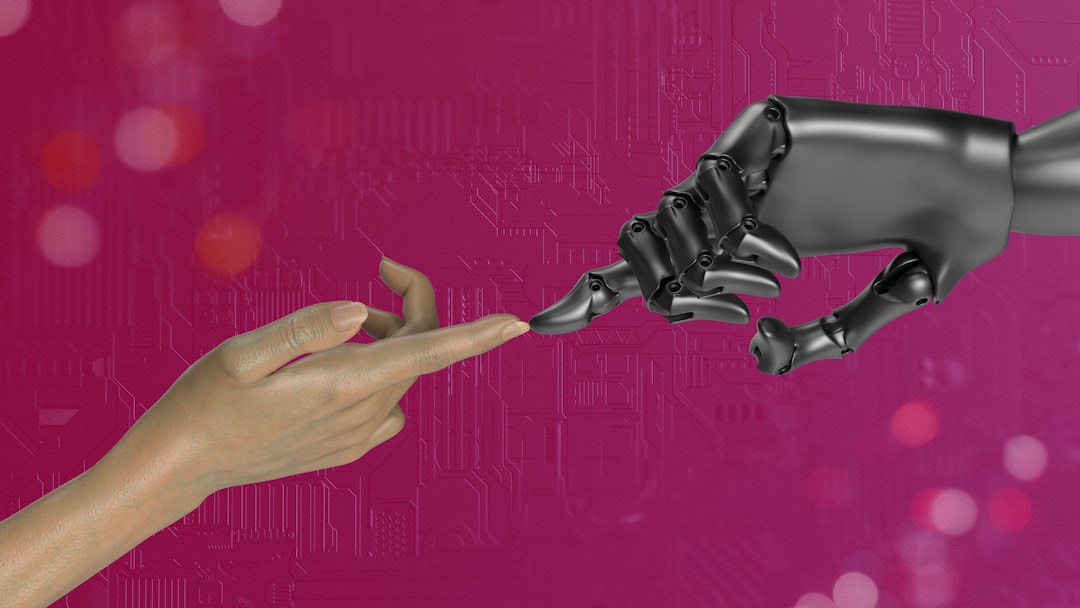
The increasing use of AI in research has led to a growing awareness of the ethical considerations involved. New guidelines have emerged to promote the responsible application of AI, particularly generative AI, in research endeavors. These guidelines acknowledge the rapid pace of AI development and emphasize the need for continuous updates to remain relevant. A key focus is on ensuring ethical practices, including promoting diversity, fairness, and preventing potential harm caused by AI systems. The guidelines also advocate for acknowledging the broader societal and environmental impacts of AI research.
Recognizing the unique challenges presented by AI, the guidelines highlight the need for researchers to understand the limitations of these technologies. To support responsible AI use, a practical toolkit has been developed to help researchers incorporate AI into their research ethically. As the use of AI expands within the scientific community, the demand for comprehensive ethical frameworks is increasing. This includes the implementation of robust ethics review processes, designed to identify and mitigate potential negative consequences of AI-driven research. Additionally, there are calls for the development of specific governance structures for AI research, potentially building on existing models used in other sectors, to guide and oversee AI-driven scientific progress in a responsible manner.
The rise of AI in research, especially with tools like the AI Scientist prototype, has spurred the development of ethical guidelines to navigate the complexities this technology brings. A major concern revolves around data privacy, especially as automated systems delve deeper into sensitive information. Researchers are increasingly urged to prioritize informed consent when working with human participant data, which is crucial for maintaining ethical integrity.
Transparency is another crucial element in these new ethical frameworks. Researchers are expected to not only meticulously document their AI methods but also make them easily understandable to a broader audience, including non-experts and the public. This push for clearer communication is meant to foster trust and open dialogue around AI-driven research.
A persistent worry is the potential for algorithmic bias, where AI systems inadvertently reflect existing societal prejudices. The guidelines advocate for regular audits of AI systems to uncover and mitigate biases that might distort results or create unfair outcomes for specific demographics.
The dynamic learning abilities of AI models also raise questions about accountability. If an AI system generates an incorrect hypothesis or flawed result, determining who or what is responsible becomes challenging. These emerging ethical frameworks encourage developing clearer structures for accountability to address such ambiguities.
Moreover, the automation of hypothesis generation raises concerns about the potential diminishment of human intuition in science. This creates a discussion on the balance between human creativity and data-driven insights in scientific discovery. Is the human element being overlooked as algorithms increasingly drive research questions?
These guidelines also promote collaborative research by encouraging interdisciplinary teams to bring diverse perspectives to bear on AI development and application. This is an attempt to make AI research more flexible and relevant across different scientific domains.
AI's capability to simulate and predict experiment outcomes presents a double-edged sword. While significantly accelerating research, the ethical use of these 'virtual experiments' needs careful consideration. Researchers are advised to be cautious about how the results of simulations influence the design and interpretation of real-world research.
The shift towards automated hypothesis generation also raises questions about who deserves credit for the research results. New ethical considerations are evolving around authorship and acknowledgment in projects involving AI to ensure fair recognition of human contributions.
The ethical guidelines also emphasize rigorous validation processes for AI-generated findings. Before results are published or used in practice, a strong emphasis is placed on verification and confirmation, which is vital for maintaining the integrity and reliability of the science.
As AI continues to evolve, the importance of continuous learning and training in ethics for researchers is highlighted in these frameworks. This ongoing development of ethical awareness aims to provide researchers with the necessary tools and perspective to navigate the changing landscape of AI in research responsibly.
AI Scientists Overcoming Creativity Bottlenecks in Automated Research - Human-AI collaboration frameworks address collective creativity challenges
The integration of AI into research and creative endeavors is increasingly emphasizing the importance of human-AI collaboration frameworks. These frameworks are moving beyond simply using AI as a tool towards a more nuanced approach where humans and AI co-create. This shift is evident in areas like education and research, where the potential benefits of collaboration are becoming more apparent. However, the rise of generative AI also brings forth questions about the nature of creativity itself. While generative AI can enhance individual creative output, some worry that it might inadvertently stifle collective creativity, the kind that emerges from diverse perspectives working together.
To address this challenge, new theories like Human-AI CoCreativity (HACC) are gaining prominence. HACC views creativity as a shared pursuit, a collaborative endeavor between humans and AI. This perspective highlights the need to carefully consider the roles and responsibilities of both agents in the creative process. Furthermore, ethical concerns related to human-AI collaborations in creative spaces require careful attention, particularly regarding the definition of roles and the potential for misuse or bias within these systems. By understanding and navigating these complexities, we can leverage human-AI partnerships to foster more innovative and collaborative problem-solving. This, in turn, can contribute to a more holistic and impactful approach to creativity within the scientific and research community, maximizing the benefits of both human ingenuity and AI's computational power.
Human-AI collaboration frameworks are shifting from simple tool usage to a model of genuine co-creation. We're seeing this impact in various areas, including higher education, where it's increasingly important for students to develop AI literacy. There's even work on designing AI assistants specifically to monitor and optimize teamwork between humans and AI, which has potential in demanding settings like search-and-rescue or complex medical situations.
However, these new generative AI tools are also challenging our long-held ideas about what creativity truly means, particularly when it comes to collaborative efforts. While these AI systems undoubtedly enhance individual creativity, there are emerging concerns that they might inadvertently reduce collective creativity. The emergence of Creative Artificial Intelligence Systems (CAIS) has reshaped the way we think about creative practices. They've emphasized the crucial role of the human collaborator in the loop, the significance of a good user experience, and the potential for entirely new modes of creative expression.
The concept of co-creation, alongside the individual's sense of confidence (self-efficacy) when working with generative AI, is becoming increasingly critical. More and more individuals are working with AI to generate creative outputs, highlighting the growing importance of these collaborative approaches. This has led to the development of Human-AI CoCreativity (HACC), a theory that frames creativity as a joint problem-solving process, emphasizing how humans and AI work together.
The explosion of AI-generated content has forced a reevaluation of traditional notions about human creativity. We're questioning long-held assumptions about the inherent uniqueness and superior quality of human-made art and creative outputs. Collaborative frameworks within AI research are revealing potential creativity bottlenecks, and researchers are working to develop strategies to overcome these, thereby enhancing collective innovation.
Naturally, ethical considerations are central to the development of human-AI collaborations, especially in creative endeavors. It's essential to establish clear boundaries about the roles of humans and AI in creative processes. The interaction between generative AI and contemporary art is an active area of study, where we're examining how AI influences both artistic perspectives and the creative process itself. It's an exciting and somewhat concerning trend we are trying to understand better.
Transform your ideas into professional white papers and business plans in minutes (Get started for free)
More Posts from specswriter.com: