Transform your ideas into professional white papers and business plans in minutes (Get started for free)
Whisper-Powered Local Captioning A 2024 Breakthrough for macOS Video Editors
Whisper-Powered Local Captioning A 2024 Breakthrough for macOS Video Editors - MacWhisper 8 Introduces Real-Time Transcription for Video Editors
MacWhisper 8 introduces a noteworthy upgrade for video editors with its new video player specifically designed to streamline the transcription process. Utilizing the WhisperKit model, it boasts on-device, real-time speech recognition tailored to Apple silicon, resulting in a reported transcription speed that can be up to 15 times faster than real-time playback. The integration of this feature makes transcribing video audio considerably easier, as users can simply drag and drop files in various common formats. The transcribed text is presented as independent subtitles, aiding in the editing workflow.
Beyond basic transcription, MacWhisper 8 provides a built-in search function, making it simple to locate specific words or phrases within the generated transcripts. While this local transcription approach promises speed and potentially increased privacy by not sending audio to external servers, it might limit its applicability for users dealing with less common audio formats or large video files. Whether or not this localized transcription approach becomes standard practice remains to be seen, but MacWhisper 8's emphasis on ease-of-use and speed makes it an intriguing development for those involved in video production and editing.
MacWhisper 8 introduces a fresh approach to video transcription by incorporating a new player feature that leverages the WhisperKit model. This model, specifically optimized for Apple silicon, utilizes hardware acceleration to perform real-time speech recognition directly on the device. The benefit for editors is clear: audio from video files is transcribed in real-time and presented as separate subtitles, streamlining the editing process. It's quite convenient that it supports a variety of audio file formats, such as MP3, WAV, M4A, MP4, and MOV, making it easy to just drag-and-drop the audio for transcription.
Interestingly, the transcription speed is claimed to be up to 15 times faster than real-time, which is a notable feat. While the software is available in both free and paid versions, the developers offer a one-time purchase option for $25, which might be a good value depending on usage. One of its unique features is the focus on local processing. The transcription happens entirely on macOS without the need for internet access or third-party services, which could be a privacy-focused design choice. Furthermore, the integrated search function allows users to quickly find specific words within the transcripts and highlight them, offering an efficient way to review and edit.
Building upon OpenAI's Whisper technology, MacWhisper aims for high accuracy across various languages. In essence, it attempts to significantly reduce the time investment in transcribing spoken audio during video editing. While the accuracy claims are appealing, real-world testing will be necessary to verify its performance in diverse audio conditions and languages. The overall goal appears to be creating a more efficient workflow, particularly in environments that necessitate quick turnaround times for transcribed video content.
Whisper-Powered Local Captioning A 2024 Breakthrough for macOS Video Editors - Whisper's Encoder-Decoder Model Processes Audio in 30-Second Chunks
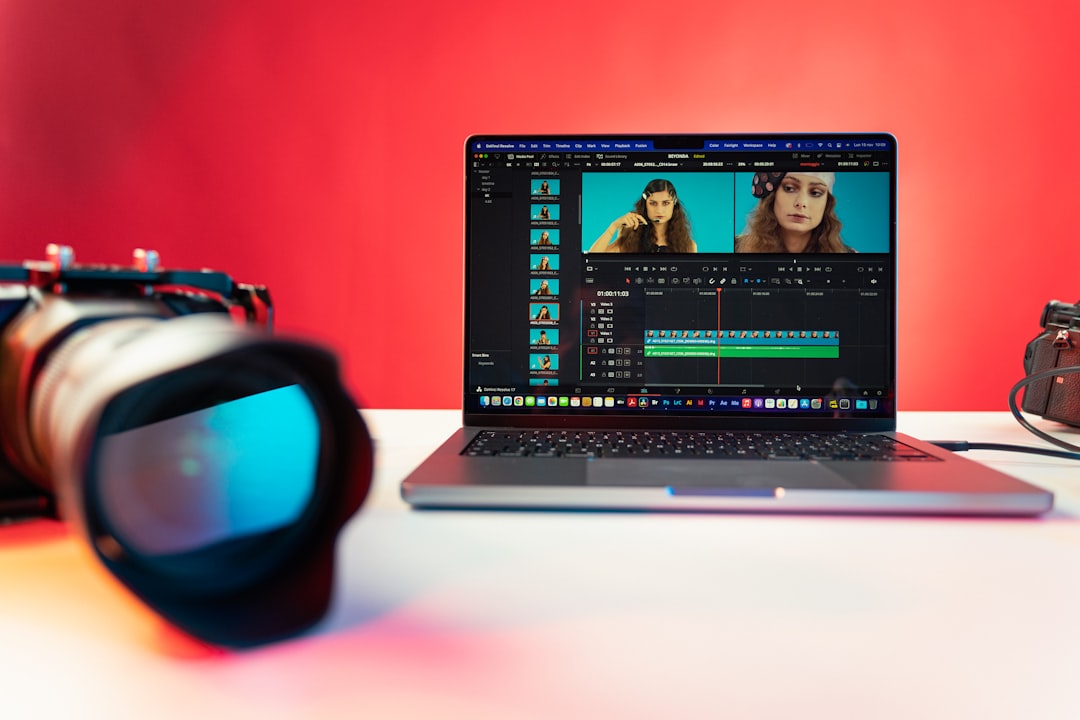
At the core of Whisper's capabilities is an encoder-decoder model that processes audio in 30-second segments. This segmented approach allows the model to handle audio more effectively. Each 30-second chunk is converted into a log-Mel spectrogram, a visual representation of the audio's frequency content over time. The encoder then takes these spectrograms and extracts meaningful patterns and contextual information from the audio signals. This information is then passed to the decoder, which uses it to generate the corresponding text transcription.
Whisper's training process is noteworthy as it relies on a weakly supervised method, meaning it doesn't require extensive manual annotation for each audio sample. It learns from a massive dataset of audio and corresponding transcripts, allowing it to handle a wide range of accents, languages, and audio qualities. Further contributing to its robustness is the autoregressive sequence-to-sequence nature of its predictions within each audio window. This method allows the model to predict words sequentially, considering the context of previously generated words, improving overall accuracy. The model also leverages special tokens that provide supplementary information, such as timestamps for specific phrases within the transcription.
Whisper's architecture includes a sophisticated Transformer design, which effectively handles the complex task of converting audio to text. Though the 30-second chunks might seem like a limitation, this strategy allows Whisper to maintain accuracy and manage the computational demands of real-time transcription. Whether this approach will continue to be prevalent or refined over time remains to be seen, but it clearly contributes to the efficiency of Whisper's current design.
Whisper's core is an encoder-decoder model built on the Transformer framework, a design choice that seems well-suited for handling audio. It processes audio in 30-second segments, a practical approach that helps to manage the computational demands of the task while also potentially improving focus on immediate speech patterns. This segmentation into smaller chunks helps avoid issues with context blurring that can happen with longer audio clips. The encoder part of the model extracts meaningful information from the audio, creating a contextual representation of the sound. Meanwhile, the decoder's job is to translate those representations into a text-based caption.
To help manage these tasks, Whisper utilizes special tokens. These tokens serve a variety of purposes within the system, such as providing the specific timestamp for different phrases during the transcription process. It’s trained on a massive dataset—a staggering 680,000 hours of audio from the internet. The model's training method is interesting in that it doesn't depend on fine-tuning from more specific benchmarks. Instead, it uses a more general approach called weakly supervised training. This seems to be part of what allows Whisper to achieve impressive multilingual and multitask capabilities. It performs well on a variety of benchmark tests and often achieves results comparable to fully supervised models when applied to new languages or tasks without any adjustments.
During the transcription process, Whisper generates predictions in a sequential manner. In essence, it makes its best guess about the next word based on what's come before, which is a typical autoregressive sequence-to-sequence technique. It essentially uses a 30-second sliding window to analyze the audio and generate the transcript. This approach is made possible by the underlying Transformer architecture, which incorporates pre-activation residual blocks and layer normalization. This implementation likely contributes to the efficiency and performance of the model. It's fascinating that this window-based approach seems to be fairly effective, but the 30-second limitation may result in accuracy challenges in more complex audio scenarios. While fast, it may need improvements for situations with substantial background noise or simultaneous speakers.
Whisper-Powered Local Captioning A 2024 Breakthrough for macOS Video Editors - Drag-and-Drop Interface Simplifies Audio and Video Transcription
The ease of use offered by drag-and-drop interfaces has made uploading audio and video files for transcription much simpler. This is now further enhanced by the adoption of OpenAI's Whisper technology, which enables local transcription on macOS, resulting in faster processing times and potentially improved user privacy since data isn't sent to external servers. This new generation of tools can handle various file formats, allowing for flexible use. The added benefits of real-time transcription, simultaneous processing of audio, and searchable transcripts are particularly helpful for video editors. While these developments are encouraging, it's still important to consider if the current versions of these tools are robust enough for scenarios with complex audio. For instance, it remains to be seen how effective they are when multiple speakers are present or there's significant background noise.
The drag-and-drop feature in MacWhisper 8's audio and video transcription tools makes it very easy to get started. It's a welcome change from the usual file import processes, and helps speed up workflows, which can be a huge benefit in environments where time is critical.
By doing the transcription locally on the user's Mac, MacWhisper 8 aims to provide a seamless experience and avoid delays caused by uploading files to external services. This local approach keeps the editor's workflow flowing without interruption.
Supporting a range of common audio formats like MP3, M4A, and MOV means editors don't need extra conversion tools. This can really help to simplify multimedia projects by reducing the technical hurdles.
While the drag-and-drop approach is helpful in the beginning of the transcription process, it does make me wonder about limitations when it comes to more unusual audio formats. It's possible that less common audio files might not transcribe as well, which could limit the tool's usefulness for some people.
The built-in search function is more than just a navigation aid—it also lets users quickly find exact timestamps within the transcription. This is a feature I've found often missing in other tools, and it can make a big difference when editors are trying to quickly make edits during the post-production process.
The real-time transcription speed (claimed to be 15 times faster than playback) could potentially impact accuracy. It's a very interesting topic for future research—how to find the right balance between speed and the accuracy of the transcriptions, especially in dynamic audio environments.
Keeping transcription on the device itself helps mitigate the privacy issues that can arise with cloud-based services. This is especially useful when dealing with sensitive audio that people may not want to share with outside services.
The reliance on Apple silicon for optimal performance raises interesting questions about future applications of these transcription tools on other hardware platforms. This could present a limitation for users who prefer a different operating system.
The simple way users can create subtitles from raw audio files could be a game-changer in the way video editing is done. Traditional workflows often involve multiple software applications, but tools like MacWhisper 8 might simplify the process, perhaps leading to changes in how localized video editing is done.
Despite the improvements, the segmented approach to audio processing could lead to drops in transcription accuracy. This is especially true in audio with a lot of background noise or overlapping dialogue. It's clear that more research is needed into how to improve transcription in these more complex audio landscapes.
Whisper-Powered Local Captioning A 2024 Breakthrough for macOS Video Editors - Local Processing on macOS Eliminates Need for Cloud Dependencies
The ability to process audio and video locally on macOS is changing how video editors work, eliminating the need to send data to cloud services for tasks like transcription and captioning. This shift is driven by the use of OpenAI's Whisper model, which can now operate directly on a Mac, resulting in faster processing times and increased user privacy because audio data stays on the user's computer. By performing transcription locally, the process is significantly faster—potentially 15 times faster than real-time playback—and less dependent on stable internet connections. While this local approach offers advantages, it's unclear how well it handles more intricate audio environments, such as situations with multiple speakers or significant background noise. Whether this localized transcription method becomes a standard in video editing workflows remains to be seen, but it's certainly an intriguing development that offers benefits for those who value both speed and privacy.
macOS's ability to handle audio transcription locally eliminates the need to rely on cloud services, resulting in significantly faster processing times compared to cloud-based alternatives. This speed can be a game changer for video editing workflows where quick turnaround is essential. Keeping the process local also presents privacy advantages, since sensitive audio doesn't leave the user's system. This is especially relevant for anyone working with confidential or sensitive material.
MacWhisper 8, for example, supports a range of common audio formats like MP3 and WAV, which is useful. However, I wonder if there will be issues with niche or unusual formats – something I'll be curious to experiment with. The developers boast transcription speeds up to 15 times faster than real-time. This is intriguing, but it makes me wonder if the system can maintain accuracy at such high speeds, particularly in complex situations with multiple speakers or significant background noise.
The training of the underlying Whisper model is based on a weakly supervised method. This means it learns from large amounts of audio and transcripts without needing extensive manual labeling. This kind of approach is interesting as it sets Whisper apart from models that usually rely heavily on highly curated datasets. Whisper's architecture handles audio in 30-second chunks. This seems like a smart approach for breaking down the task into smaller, more manageable pieces. However, it might lead to issues with longer conversations or scenes with quick shifts in topics or speakers.
MacWhisper 8 also includes a built-in search function that lets users quickly locate words or phrases in the transcripts and also retrieve their timestamps, greatly assisting the editing process. The Whisper model’s encoder-decoder architecture leverages a Transformer design, a popular structure for handling sequences. It processes audio and outputs text, but its autoregressive nature may struggle in situations where there are rapid changes in dialogue. The fact that MacWhisper 8 has been optimized for Apple Silicon means it's exceptionally fast on Apple's latest hardware, which is nice. But, that also means that compatibility with other systems is an open question. It will be interesting to see if this local approach to transcription becomes more common, as it could completely change how we handle video editing. This field is rapidly evolving, and it will be important to keep track of how these localized methods improve and mature over time.
Whisper-Powered Local Captioning A 2024 Breakthrough for macOS Video Editors - GPU and CPU Compatibility Offers Flexible Performance Options
The ability to use both GPUs and CPUs offers a range of performance options for tasks like audio transcription with Whisper. Utilizing a GPU, especially those with ample memory and processing capabilities like the RTX 4070, can speed up transcription significantly compared to relying only on the CPU. This flexibility allows users to choose hardware that best fits their workflow, whether that involves demanding real-time captioning or handling large audio files. While GPUs clearly improve performance in many scenarios, there are still limitations. For example, it's not entirely clear how well they handle intricate audio situations with numerous speakers or significant background noise. As video editing continues to embrace AI-driven tools like Whisper, understanding the interplay between hardware and software becomes crucial for optimizing performance in different real-world applications.
The interplay between CPUs and GPUs offers a fascinating range of performance possibilities, particularly for tasks like transcribing audio in video editing. GPUs, with their parallel processing capabilities, excel at accelerating the intricate calculations involved in AI models like Whisper. This advantage stems from their ability to handle numerous operations simultaneously, a strength that CPUs, despite their higher clock speeds, don't fully replicate.
The speed boost we see when using GPUs often comes down to faster data transfer rates. While CPUs are designed for handling sequential operations, GPUs can juggle many tasks at once, which is particularly useful for processing large chunks of video data. Additionally, GPUs typically boast a greater memory bandwidth, facilitating the swift movement of data within the system – crucial for the demanding memory access patterns often seen in AI models.
This parallel processing strength comes from GPUs' architecture, which features many smaller cores. When Whisper is processing audio for transcription locally, this architecture shines, enabling it to tackle multiple audio segments concurrently and deliver much faster results.
The rise of AI-optimized chips like Apple's neural engine adds another layer to this performance picture. These specialized hardware components can work alongside traditional CPUs and GPUs, tailoring their efforts to specific machine learning tasks, potentially pushing the boundaries of audio processing even further.
There's also the element of thermal management to consider. The way CPUs and GPUs handle heat can significantly impact sustained performance under heavy loads, like those encountered during real-time transcription. GPUs often distribute heat more effectively, helping them maintain a steady performance level, something traditional CPU designs may struggle with.
Furthermore, the ongoing optimization of software like MacWhisper 8 for both CPUs and GPUs on macOS contributes to these speed improvements. Developers continuously refine their models, making them better at utilizing the strengths of each processor type. The nature of the instructions each uses (e.g., SIMD instructions) also plays a role. CPUs can use SIMD to speed up tasks by working on multiple data points at once, but GPUs take it to a whole other level in areas like running neural networks.
The ever-changing nature of video editing workloads presents a chance for CPUs and GPUs to dynamically share duties. This adaptability can lead to more efficient workflows, as each type of processor tackles the aspects it's best suited for. In the context of real-time transcription, this translates to potentially quicker overall processing times.
As both CPUs and GPU technologies continue to evolve, we'll likely see even smoother compatibility and potentially more performance breakthroughs in transcription. This trend has the potential to significantly redefine the video editing process, creating new and streamlined ways to work with audio and video content. The fusion of CPU and GPU power seems set to drive exciting future possibilities for efficient real-time features in video editing.
Whisper-Powered Local Captioning A 2024 Breakthrough for macOS Video Editors - Sliding Window Technique Enhances Transcription Accuracy
The Sliding Window Technique plays a crucial role in improving the accuracy of transcriptions, especially within video editing workflows. By segmenting audio into smaller, manageable chunks, the transcription process can focus on immediate speech patterns and related context. This technique complements the segmented approach already used by models like Whisper, allowing for efficient real-time transcriptions. However, this approach isn't a panacea. It's still a challenge to ensure high accuracy in situations where there are several speakers or a noisy environment. As the technology develops, finding the right balance between the speed of transcription and the accuracy of the results will be important for those involved in video editing. The ability to maintain good accuracy even under difficult conditions will influence how the technique is applied in the future.
Whisper's approach to audio transcription relies on a 30-second sliding window, a strategy that's not typical in many other models. This method allows the model to maintain a focus on immediate speech patterns while avoiding potential noise or context blur from longer audio segments. This windowing also contributes to a more refined understanding of the audio's frequency content over time using log-Mel spectrograms, potentially capturing nuances that might be missed with less granular techniques.
Interestingly, Whisper doesn't need heavily labeled data to train. Instead, it uses a weakly supervised learning method, which is able to take advantage of a massive 680,000 hour dataset of audio and transcripts. This approach, which isn't always employed in AI models, may be contributing to the model's robustness and ability to handle varied audio conditions. The model's architecture is built on a Transformer framework, which uses attention mechanisms that can help the model to selectively focus on crucial elements within the audio stream. This seems to contribute to its accuracy, at least in many situations.
The use of the sliding window method within Whisper's design allows for rapid transcription speeds - up to 15 times faster than real-time on compatible hardware. This speed can be attractive, but might also introduce challenges to accuracy when the audio is fast-paced or complex. Like other models, Whisper uses an autoregressive approach where it guesses the next word based on what it has already processed. While this works well in many scenarios, it could lead to accuracy issues if there's a sudden shift in topic or overlapping voices.
Another interesting design element is how it handles memory and processing. Because the model works in these 30-second segments, it can work on several at once. This helps to optimize how memory is used and can potentially minimize delays that might occur if processing had to wait for longer stretches of audio. The encoder-decoder model structure also incorporates special tokens that provide timestamps for certain phrases during transcription, a handy capability for tasks that involve detailed editing or audio analysis. The sliding window also allows the model to more easily adapt to different speaking styles and tempos, which is a key feature in situations where speakers might have variable speeds or cadence.
The successful integration of the sliding window technique in Whisper suggests there's ongoing research into developing more sophisticated segmenting techniques. The goal of this research is to enhance transcription capabilities for audio that might be problematic for the current generation of models. This includes instances with background noise, multiple overlapping speakers, or other challenging conditions. While current applications of Whisper are showing promising results, addressing these difficult situations is a central focus of future research into this innovative method of audio processing.
Transform your ideas into professional white papers and business plans in minutes (Get started for free)
More Posts from specswriter.com: