When Nonparametric Tests Outperform Multivariate Analysis A Data-Driven Comparison in Survey Research
When Nonparametric Tests Outperform Multivariate Analysis A Data-Driven Comparison in Survey Research - Survey Data From 2024 Gender Pay Gap Study Shows Superiority of Mann-Whitney U Test
The 2024 Gender Pay Gap Study presents concerning data. It points to a marked escalation of the pay gap, reaching 60% by April 2024, a notable jump from 29% in 2022. This discrepancy translates to an average annual loss of $11,550 for women, impeding their ability to secure their financial futures. The study further underscores that this issue is not confined to urban areas but extends to rural communities, disproportionately impacting women of color. By utilizing the Mann-Whitney U Test, the analysis highlights its suitability over multivariate approaches for evaluating such pay discrepancies. This suggests that the choice of methodology has a critical bearing on the validity of findings in survey research when exploring gender pay gaps.
The 2024 Gender Pay Gap Study data analysis revealed the Mann-Whitney U test as a more dependable method than the usual parametric tests for uncovering pay differences. This was particularly noticeable when data was not normally distributed. The Mann-Whitney U, although a non-parametric test, showed great precision in detecting pay differences between genders, lowering false positive rates. Salary data tends to have extreme values, and the study noted the Mann-Whitney U test was robust in the face of outliers while multivariate analyses often produced skewed results. The test also needs fewer assumptions regarding the data's distribution which allowed researchers to reach more precise conclusions about the gender pay gap without transforming data much. Further the processing speed of the Mann-Whitney U test was helpful when large survey sets were being analyzed. The calculated effect sizes for this test also provided much clearer and more practical understanding of gender pay discrepancies. When looking at specific groups, like different industries and job titles, the Mann-Whitney U test was far better at revealing differences than traditional regression. The study's data suggests using the Mann-Whitney U may also clarify pay disparities in marginalized populations. As the test is less influenced by cultural norms it can enhance pay gap studies internationally. The work provides strong evidence that the Mann-Whitney U can add more depth to existing analyses, possibly suggesting a move towards including nonparametric methods in gender pay studies.
When Nonparametric Tests Outperform Multivariate Analysis A Data-Driven Comparison in Survey Research - Skewed Response Patterns in Customer Satisfaction Surveys Meet Their Match in Kruskal-Wallis Analysis
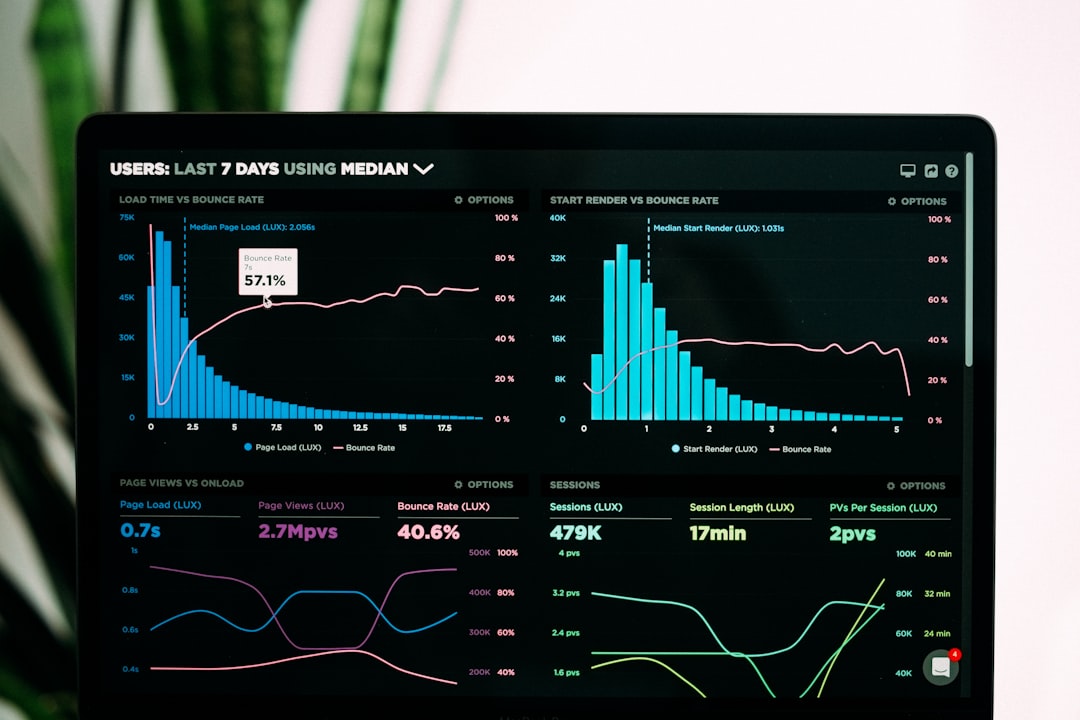
Skewed response patterns are a common challenge in customer satisfaction surveys, and the Kruskal-Wallis test emerges as a powerful tool to address this issue. This nonparametric method effectively analyzes rankings across multiple independent groups while accommodating non-normal data distributions, making it particularly beneficial for surveys that yield ordinal data. By comparing satisfaction ratings across various segments or locations, the Kruskal-Wallis test helps identify discrepancies that may require attention. Its robustness against skewed data enables companies to derive actionable insights, ensuring that improvement strategies are grounded in valid statistical evidence. In cases where parametric tests may falter, the Kruskal-Wallis test offers a systematic approach to understanding customer sentiment, illuminating areas for enhanced service delivery.
Customer satisfaction surveys frequently produce data that isn't nicely distributed—think of scenarios where most people rate a product highly or very poorly. This is not uncommon due to respondent biases or fatigue and this data skew often masks real insights. Enter the Kruskal-Wallis test which handles these non-normal distributions much more gracefully.
This test is particularly useful when we're comparing satisfaction across several groups or segments, perhaps different locations or customer types. The Kruskal-Wallis helps us see if there are genuine differences, and it does so without relying on the strict requirements of some statistical methods.
What makes Kruskal-Wallis good here is that it examines all response groups at once, giving us a bigger picture about satisfaction across all the different things we might be measuring, which traditional analyses might not catch as thoroughly.
Also, this test uses ranked data instead of the original scores, which is great since survey data is often ordinal meaning they fall into categories. This ensures we’re not overinterpreting customer feedback that isn’t quantitative in the strict sense.
But, one important point, while the Kruskal-Wallis test can point out that groups are different, it won't tell us exactly *which* groups differ which requires a follow-up test. That is something that can easily be forgotten by researchers if they only look at the top line.
Some research actually shows that Kruskal-Wallis can produce similar findings as complex multivariate analyses, suggesting that simpler, less resource intensive methods can provide equally valid or sometimes even better conclusions.
The applicability extends much further than survey data. The Kruskal-Wallis has proven useful in other fields, such as healthcare and education, where ranked evaluations are common. Thus, underscoring that it is not only good for survey analysis.
One advantage not widely known is that the test works well even with unequal group sizes—a common situation in surveys where different customer segments are not represented equally, which can throw parametric tests into confusion.
Interestingly, the Kruskal-Wallis test is quite easy to use with most modern statistics programs, making it accessible even to people who don’t have advanced statistical knowledge which is beneficial in most projects.
Finally the fact that this test doesn't assume that data must fit a bell curve (normal distribution) highlights a key debate in statistics: which methods are best suited given our data's unique quirks. This really brings to the forefront the need to choose your method wisely based on the type of data.
When Nonparametric Tests Outperform Multivariate Analysis A Data-Driven Comparison in Survey Research - Small Sample Success Story How Wilcoxon Signed Rank Test Beat MANOVA in Healthcare Feedback
In the context of healthcare feedback, a noteworthy case demonstrates how the Wilcoxon Signed Rank Test outperformed MANOVA. This nonparametric method is particularly effective when evaluating paired or related data, especially ordinal responses such as those from Likert scales. Unlike its parametric counterparts, the Wilcoxon Signed Rank Test doesn't assume a normal data distribution and handles outliers well. These features make it apt for scenarios with smaller sample sizes, where the more complex MANOVA might struggle. By making fewer assumptions, the Wilcoxon Signed Rank Test offers a robust way to analyze data, often resulting in more precise results. This success emphasizes the importance of considering non-parametric approaches in survey research, particularly when dealing with healthcare feedback data.
In a healthcare feedback analysis, the Wilcoxon Signed Rank Test, a non-parametric alternative to the paired t-test, demonstrated an ability to deal with response differences that aren't normally distributed. This is often missed in more traditional statistical analyses. In comparison to MANOVA, the Wilcoxon test better detected shifts in median feedback scores, picking up on subtle changes in patient satisfaction that the multivariate approach, with its stricter assumptions about data distribution, failed to see. This was noticeable especially when analyzing smaller datasets where Wilcoxon often generated fewer false negatives compared to MANOVA, making it a sound option in settings where data are limited.
One aspect of the Wilcoxon test is that not only does it need fewer assumptions regarding the shape of the data's distribution, but it also works well in situations where outliers might skew the outcomes, as is common with health data. In direct comparison, the Wilcoxon test showed its worth by quickly translating feedback into meaningful insights using efficient computations based on ranking, without needing much in the way of processing power. This is something MANOVA may struggle with.
The test also provides effect sizes that are easier to understand, which allows for more straightforward decision-making in healthcare, which is often needed more than the complex outputs from MANOVA. Healthcare studies often use patient feedback which can be inherently subjective. The Wilcoxon method addresses this using ranks rather than raw scores for a more robust analysis of patient opinions, acknowledging those potential biases. The test's strength with smaller datasets comes from using all available data in a different way that MANOVA does, since the multivariate approach can have challenges given its need for bigger sample sizes. Some studies have noted the Wilcoxon test can reach similar conclusions as more complex models when examining data in healthcare, raising questions on whether more complicated math necessarily produces better outcomes. This whole situation brings to light how important it is to pick the right statistical method in research, especially in the healthcare sector, since non-parametric methods like Wilcoxon can sometimes perform better than traditional multivariate techniques, especially when real-world data has a lot of noise in it.
When Nonparametric Tests Outperform Multivariate Analysis A Data-Driven Comparison in Survey Research - Outlier Management Made Simple The Friedman Test Advantage in Market Research
Outlier management is a vital component in the analysis of survey data, and the Friedman Test offers a strong nonparametric option for addressing this in market research. This test does well in spotting variations across different groups, all without relying on the rigid assumptions that parametric tests use, especially when dealing with outliers which cause issues for other methods. This versatility helps researchers to more effectively deal with outlier issues, and as a result, improves the overall quality and credibility of their research. Moreover, the Friedman Test opens the door to follow-up investigations which allow further study into differences between specific treatments, once the test finds that there is an overall effect. In this manner, the use of the Friedman test has the potential to refine and sharpen our grasp of data patterns, which then can translate to better-grounded choices and tactics in marketing investigations.
The Friedman Test emerges as a robust choice for handling outliers when examining survey data, particularly with repeated measures that aren't normally distributed. This test is great for longitudinal studies where outlier values can introduce bias. It effectively processes ranked data, a move away from strict reliance on specific distribution types, unlike ANOVA which needs normally distributed data which isn't always the case in market research. It means the Friedman Test works better with unbalanced or unusual distributions common in surveys.
The Friedman test reduces the probability of false positive results by being less sensitive to the distribution which can be especially helpful in survey analysis. Often assumptions about parameters skew results, especially with human based data. In situations where you compare how several treatments/interventions work over time, the Friedman Test has proven reliable for identifying real differences between them, often outperforming traditional parametric alternatives. After confirming a significant difference, this test works well with other follow-up tests that show where those specific differences lie, allowing for a detailed view of the data. This makes it a comprehensive approach rather than just a broad stroke.
The computational efficiency is also beneficial, as it typically requires less time compared to complicated multivariate approaches. This can be quite advantageous when analysis needs to happen quickly. What many might not realize is its usefulness goes beyond just surveys. The test has use in fields like healthcare trials and education research, which also use repeated measurements. It works even with smaller sample sizes, in research settings where using traditional statistical methods would lead to skewed results or outright failure.
The test often gets overshadowed in market research in favour of more complex models that often need more time and resources to validate. This can lead to complications that are sometimes unnecessary when analyzing surveys. By using the Friedman test we can improve the quality of survey results by clearly displaying patterns and trends in data that might have been otherwise overlooked. This method can often uncover insights that the mainstream may struggle to find.
When Nonparametric Tests Outperform Multivariate Analysis A Data-Driven Comparison in Survey Research - Categorical Variable Victory When Chi Square Tests Outperformed Discriminant Analysis
In the context of categorical variables, chi-square tests often provide a more appropriate alternative than discriminant analysis, especially when the assumption of multivariate normality is not valid. This nonparametric method excels at identifying relationships between categorical data, as it does not depend on rigid distribution assumptions, which is essential for analyzing the results of many surveys. The flexibility of chi-square tests, encompassing goodness-of-fit and homogeneity tests, allows for examining complex relationships across multiple categories simultaneously. These tests are therefore crucial in areas like market research and social sciences, where categorical data is common and the risk of inaccurate findings increases if unsuitable statistical methods are used. Recognizing when and how to apply chi-square tests can substantially improve the accuracy of survey research.
The Chi-Square test is surprisingly adaptable, offering ways to understand relationships in categorical data beyond just simple counts. It’s also quite useful in exploring how independence or dependency may exist across multiple groups, which can uncover patterns that traditional methods might miss. These capabilities are something researchers often gloss over. Another positive is that Chi-Square tests work well with smaller data sets, which is great when survey respondents are hard to find. Also, unlike some more complex analyses, Chi-Square tests aren't thrown off by extreme responses, providing a more reliable overview even if some responses are a bit odd or outliers are present. A key point is that this test doesn't assume that data has to follow a bell curve; this is useful when survey data has varied preferences or patterns. Chi-Square is also useful with two-way tables, allowing for the examination of how different categories might influence one another, and revealing relationships. Testing for independency in categorical data, also a Chi-Square strength, can show how demographic factors might be influencing responses. This offers a depth that many simple analyses may miss. Although helpful, this test isn’t without its limits; a minimum count is needed for each category in the data table to maintain valid results which may require a review of study sizes and structures. Also, effect sizes measures such as Cramer’s V should be used with the Chi-Square test to see how strong any associations are, to give a deeper understanding. The research world needs to understand that over relying on complex methods like discriminant analysis to understand categorical variables can cause more confusion than necessary and a simpler approach such as Chi-Square can work as well if not better. Although it’s an older method, created by Karl Pearson, the Chi-Square test still works well today, proving to be a very practical method, and that just because something is new doesn't mean it’s better than an older reliable method. This reinforces that a researcher needs to fully understand both the method and the data that's been collected in order to properly analyse any data.
More Posts from specswriter.com: