Turnitin's AI Detection Accuracy and Limitations in 2024
Turnitin's AI Detection Accuracy and Limitations in 2024 - Turnitin's claimed 98% accuracy rate in AI detection
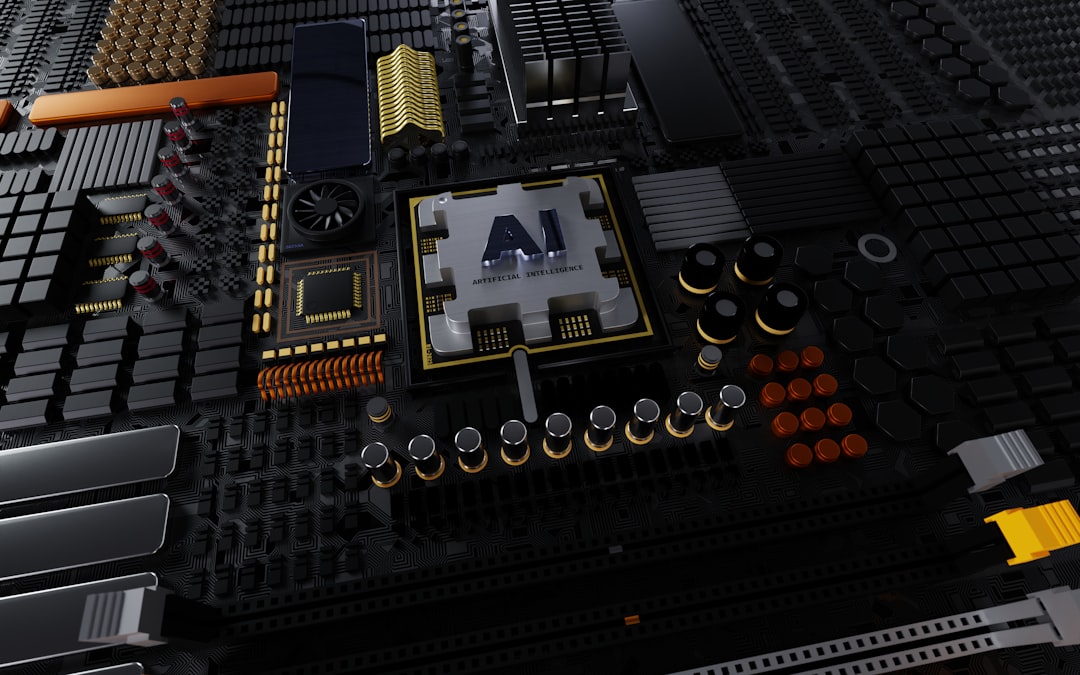
Turnitin promotes its AI detection tool with a 98% accuracy claim, but this comes with a caveat: a 1 in 50 chance of mistakenly flagging human-written work as AI-generated. Efforts to reduce these false positives have included adjustments like raising the minimum word count and refining how sentences are analyzed, but questions remain about the tool's overall reliability. Since its introduction, the tool has processed a vast number of student papers and has flagged a considerable portion as primarily AI-written, leading to concerns regarding its effectiveness. As Turnitin continues to refine the tool based on user feedback, it's faced with the challenge of achieving strong AI detection while minimizing the risk of mislabeling authentic student writing.
Turnitin promotes a 98% accuracy rate for its AI detection feature, but it's crucial to consider how that figure is derived. It relies on Turnitin's internal algorithms and the specific training data they've used, raising questions about how well it performs across the vast range of AI-generated text out there. This percentage primarily focuses on whether something is AI-written or human-written. However, it doesn't necessarily guarantee the system can pinpoint the specific type of AI model used or understand the subtle contexts in which AI-generated content is employed.
Researchers have observed that AI detection performance can vary depending on the specific AI model generating the text. This suggests that Turnitin's accuracy could fluctuate depending on which AI platform is used. The 98% figure also assumes that AI-generated content has consistent patterns, which may not hold true as AI language models continue to evolve. The more sophisticated they become, the harder it might be to distinguish their output from human-written content. Furthermore, humans can, in some cases, produce text that unintentionally mimics AI patterns. This introduces the possibility of incorrect flagging, questioning the reliability of Turnitin's claim.
Turnitin's AI detection is constantly being refined, meaning the effectiveness can vary, especially as new AI models emerge. Since the algorithms learn primarily from known AI examples, novel or less-common AI systems might slip through the cracks, affecting the claimed accuracy. While a high accuracy rate gives us some confidence in the tool, it could also breed over-reliance. Educators might not scrutinize flagged content as closely as they should, relying too much on the 98% figure.
The 98% statistic doesn't delve into whether biases may exist in the data used to train the AI detector. This raises the possibility that the tool might perform inconsistently across different writing styles or demographic groups. Finally, it's important to remember that Turnitin's accuracy claims don't encompass the full complexity of academic dishonesty. They're primarily focused on AI-generated text, not necessarily plagiarism in its broader forms. Educators need to recognize this distinction and fully understand both AI content detection and plagiarism when interpreting the results from Turnitin.
Turnitin's AI Detection Accuracy and Limitations in 2024 - 10% of papers contain significant AI-generated content
Based on Turnitin's analysis of a large number of student papers, roughly 10% are found to contain a significant portion of content generated by AI—at least 20% of the text. This indicates a growing presence of AI-written work in academic settings. This trend highlights the challenges educators face in ensuring academic integrity in an era where AI writing tools are readily accessible. While Turnitin's AI detection technology aims to identify these instances, concerns remain regarding its complete effectiveness. The reliability of these tools in consistently differentiating between AI-generated and human-written content is still being debated. As AI continues to advance, the methods used to detect its presence in academic work require ongoing evaluation to keep pace with the evolving capabilities of these technologies. The need to balance maintaining academic standards with the opportunities presented by AI remains a central issue for educational institutions.
The finding that roughly 10% of papers assessed contain a substantial amount of AI-generated content highlights the swift integration of AI into academic writing. This statistic, while seemingly modest, prompts contemplation regarding the future of originality and authorship in scholarly work. Many educational settings may not be completely prepared for the ramifications of this trend, particularly when evaluating student assignments and maintaining academic integrity.
The degree of AI usage in student papers can differ across disciplines. For instance, technical fields, where automated code generation is more common, might exhibit higher AI integration compared to humanities disciplines. The 10% figure could, in fact, underestimate the actual prevalence of AI usage as AI-generated content becomes increasingly sophisticated and more difficult to detect.
It's been observed that AI-generated text can frequently blend seamlessly with human writing. This presents challenges in effectively detecting AI content and raises questions about how accurately we can gauge student learning when AI assistance is involved. Moreover, the 10% statistic hints at a larger pattern—the widespread adoption of AI writing tools in academic environments, potentially leading to blurred lines of authorship and a diminishing sense of individual contribution in scholarly work.
While not necessarily indicative of malicious intent, student use of AI-generated content might be motivated by a desire for enhanced understanding or streamlined writing. This introduces a dilemma for educators, who must carefully navigate between what constitutes appropriate collaboration with AI and instances of academic misconduct during the process of student evaluation.
The rise of AI-powered content generation tools introduces the risk of unintended consequences. One concern is the potential suppression of creativity and critical thinking among students. These issues warrant thoughtful discussion within the education community.
Furthermore, this statistic brings to light the possibility of biases and inaccuracies within AI-generated content. This raises concerns about the reliability of information presented in academic works produced with AI assistance. As a researcher, it's crucial to be mindful of this potential, as we navigate an era where the boundaries of human and AI contributions in knowledge creation are becoming increasingly intertwined.
Turnitin's AI Detection Accuracy and Limitations in 2024 - Recognition at 2024 Bett Awards for academic integrity support
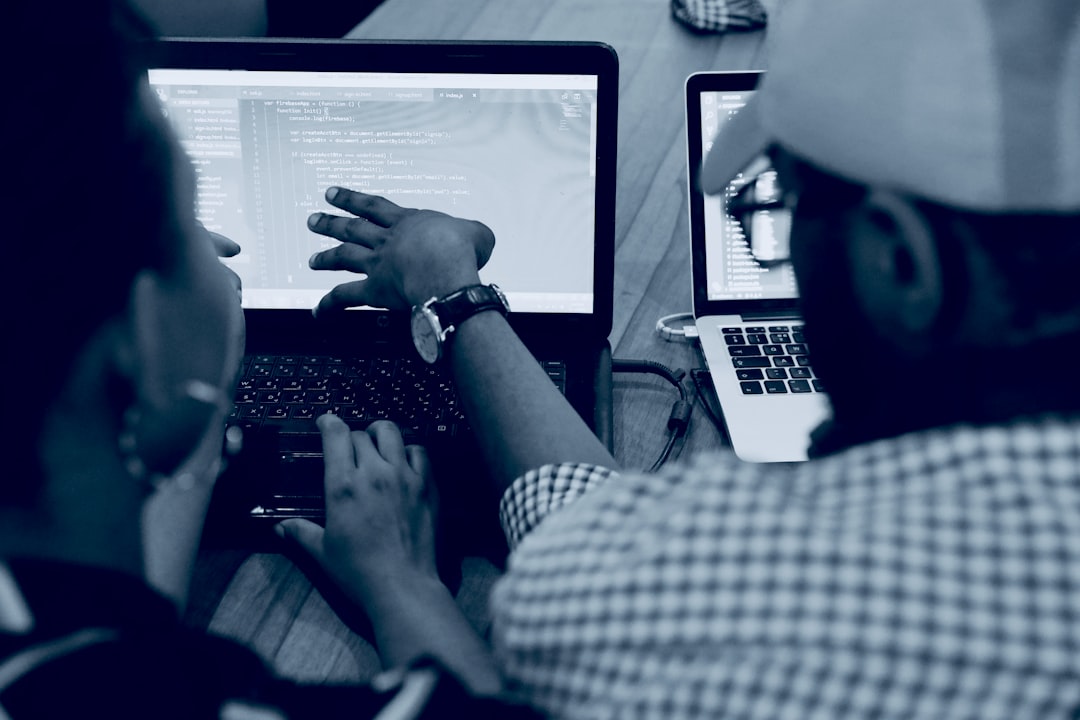
Turnitin received recognition at the 2024 Bett Awards for its contributions to supporting academic integrity, specifically through its AI writing detection tool. This recognition, within the "AI in Education" category, highlights the innovative nature of the technology designed to identify content generated by AI writing tools. The tool's impact has been acknowledged beyond this award, with past recognition such as "Best in Show" at ISTE 2023, indicating its role in shaping how educators approach the use of AI in classrooms. While Turnitin's AI detection tools aim to help maintain academic integrity in a changing educational environment, the system’s limitations, particularly the possibility of mistakenly flagging human-written text as AI-generated, remain a cause for concern. This calls into question how effectively the tool can promote true understanding of students’ original work when AI is readily accessible. The ongoing evolution of AI and its integration in educational settings requires continued examination of the impact of these technologies on academic integrity.
Turnitin's AI writing detection feature received recognition at the 2024 Bett Awards, specifically for its contribution to supporting academic integrity. This recognition, within the context of the "Oscars of the education world," highlights the increasing awareness of the challenges AI presents in education. It seems to mark a shift in how educational institutions are grappling with technological advancements and their impact on integrity.
During the Bett Awards judging process, Turnitin's AI detection feature was noted for prompting conversations among educators about the ethical use of AI in academic work. This suggests a move towards a more comprehensive understanding of AI's role in education, beyond just its technical capabilities.
Turnitin's AI detection is an ongoing project, needing continuous adjustments as AI models continue to develop. This illustrates the dynamic landscape of AI technology, where detection tools must adapt quickly to avoid falling behind in the arms race with new AI-generated content.
This Bett Award, alongside the previous ISTE recognition, emphasizes Turnitin's dedication to managing the challenges posed by AI in writing. The award acknowledges their efforts and further underscores the need for frameworks promoting ethical writing practices among students.
We've seen roughly 10% of student papers flagged for substantial AI-generated content, revealing the increasing adoption of AI by students. This raises substantial questions about the future of originality and authorship in academia. AI's potential to reshape traditional education norms is now undeniable.
The Bett Awards' focus on academic integrity support further emphasizes the difficulties educational institutions face in distinguishing between AI assistance and academic misconduct. This dilemma reflects a broader societal need for clearer ethical guidelines regarding AI use in educational contexts.
Turnitin's approach has led to a heightened interest among educators on integrating AI detection tools into their teaching practices. This reflects a growing acknowledgment that technology can enhance education when implemented responsibly.
The Bett Awards panel also stressed the importance of creating educational resources for both students and faculty regarding AI ethics and usage. This indicates a recognition that knowledge and understanding are fundamental for upholding integrity in the digital age.
With increased AI writing assistance tool availability, there's understandable concern that student critical thinking skills might be impacted. This crucial discussion is gaining momentum, leading to innovative approaches to education that promote independent learning.
The conversation surrounding academic integrity is shifting. Instead of primarily focusing on punitive actions for violations, educational leaders are advocating for proactive education. This new perspective underscores the importance of cultivating an environment of ethics and responsibility as AI capabilities accelerate.
Turnitin's AI Detection Accuracy and Limitations in 2024 - Specialized design for identifying AI in student writing
Turnitin's 2024 introduction of a specialized AI detection system for student writing represents a significant step in addressing the challenges of AI-generated content in education. This system, built on years of experience in academic integrity, is designed to specifically identify text created by large language models (LLMs) and AI-paraphrased material. It provides a unique AI writing detection score, separate from the usual similarity scores, offering educators a breakdown of potential AI usage in student submissions. While Turnitin boasts a high accuracy rate, the possibility of false positives, where genuine human writing is misidentified as AI, remains a point of concern. The tool's integration into the familiar Turnitin Feedback Studio makes it easy to access, but its overall reliability and the long-term impact on academic integrity are ongoing discussions. With the widespread adoption of AI writing tools by students, questions about originality and authorship within education continue to surface as standards adjust to this new era.
Turnitin's AI detection system is built upon sophisticated algorithms that analyze the intricate nuances of language, including patterns, sentence structure, and meaning. These algorithms are trained to recognize subtle differences between human and AI-written text, which can be surprisingly difficult to distinguish. However, the effectiveness of these tools hinges on their ability to adapt to the ever-evolving landscape of AI models. As new AI models emerge, detection systems must continuously adapt and refine their approach to keep up with the evolving capabilities of AI writing.
The training of these algorithms relies heavily on vast datasets of writing samples. However, there's always a risk that these datasets may contain biases, potentially leading to inconsistencies in the tool's performance across various writing styles or demographics. It's vital to ensure that the training data represents a broad range of writing to minimize any potential biases. While Turnitin promotes a high accuracy rate, the actual error rates can vary widely depending on the specifics of a student's writing style and the content being addressed. This variability means we need to interpret the results with caution, understanding that there's a potential for both false positives and false negatives.
Turnitin's AI detector incorporates a feature that learns from past results in real time. This allows the system to adapt and improve accuracy as it processes new student work. It learns from its mistakes and incorporates feedback, which is crucial for refining its performance over time. The adoption of AI writing tools varies considerably across different academic disciplines. For instance, fields like engineering, where code generation is common, might see more AI usage than fields like literature. This means that developing detection mechanisms might require tailored approaches for different disciplines to account for the unique characteristics of writing styles within those areas.
One intriguing aspect is that human writers can occasionally unknowingly produce text that mimics the patterns of AI-generated content. This can make detection challenging, potentially leading to the misidentification of human-written work as AI-generated based on superficial structural similarities. The continuous use of AI detection systems can help reveal trends in student AI usage. Analyzing this data over time can help educators anticipate challenges to academic integrity and proactively develop strategies to address them. It's important to note that while Turnitin's tool excels at differentiating AI from human writing, it doesn't necessarily cover all forms of plagiarism. This limitation highlights the need for educators to also consider broader plagiarism concerns when evaluating student work.
Finally, the design of AI detection tools also strives to be user-friendly for instructors. They are designed to deliver readily interpretable results, making it easier for educators to quickly evaluate submissions. This is particularly helpful given the anticipated increase in AI-generated content in educational settings. In essence, the aim is to streamline grading while ensuring the integrity of academic work.
Turnitin's AI Detection Accuracy and Limitations in 2024 - Integration with existing Turnitin systems and pre-ChatGPT development
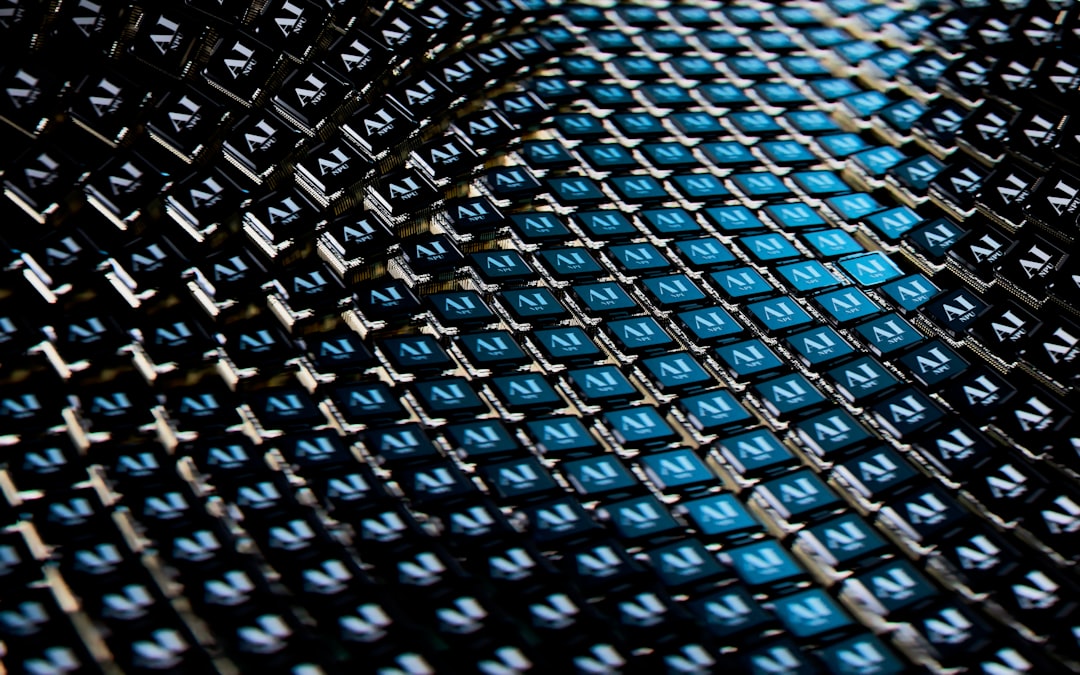
Turnitin has seamlessly incorporated its AI writing detection features into its existing systems, such as Feedback Studio and Originality, making it readily accessible to educators. This integration aims to enhance the detection of AI-generated text, especially content produced by tools like ChatGPT, with the goal of helping maintain academic integrity. These AI detection capabilities, which are available to over 62 million students, are a response to the increasing use of AI in academic writing.
While the intention is to minimize incorrect classifications, it's a continuous process of refinement. Turnitin is actively working to enhance its AI detection algorithms to keep up with the ever-changing landscape of AI writing tools. It's a significant challenge since the line between human and AI-generated content continues to blur, making it difficult to be absolutely sure of detection accuracy. The integration of these features into pre-existing Turnitin systems is designed to be user-friendly, requiring no significant changes for current users and offering an additional layer of assessment for educators. This approach highlights the importance of staying ahead of AI advancements in educational settings, recognizing the evolving nature of student work and ensuring instructors have the tools needed to maintain academic integrity in the face of these changes.
### Integration with Existing Turnitin Systems and Pre-ChatGPT Development
1. **Early Stages**: Turnitin's journey into AI detection began as an expansion of their existing services, moving beyond traditional plagiarism checks to confront the emerging issue of AI-generated text. This shift was evident even before ChatGPT's popularity, as educators started encountering AI-generated content from various, less sophisticated models.
2. **Initial Learning**: The first AI detection tools were built using machine learning models trained primarily on pre-existing human-written academic works. This approach, though foundational, raised concerns about how well these tools could adapt to newer AI models that utilized different learning methods and language styles.
3. **Focusing on Structure**: The initial focus of these AI detection algorithms leaned heavily on identifying patterns in sentence structures and common phrases. However, as more advanced AI models emerged, they became increasingly capable of mimicking human writing styles, making it harder for the earlier detection methods to accurately identify AI-generated text.
4. **Blending Old and New**: Combining the new AI detection functionalities with Turnitin's existing infrastructure wasn't easy. Ensuring that the upgrades didn't disrupt the core plagiarism detection system required extensive testing, since educators were accustomed to and relied upon the established methods of assessing similarity scores and historical data within Turnitin.
5. **Learning from Feedback**: Turnitin actively sought feedback from early users of their AI detection features. This iterative process, where the tool was continuously refined based on user experience, emphasized a key principle of engineering: improving tool reliability through ongoing user interaction.
6. **Limited Early Models**: Before ChatGPT's rise and the proliferation of similar powerful generative models, the early AI detection systems faced limitations in their ability to accurately detect text created by less complex AI tools. This impacted the reliability of their initial performance measurements, and underscored the need for continuous evaluation of their effectiveness.
7. **Early Insights**: Prior to widespread AI tool adoption in classrooms, Turnitin could only offer preliminary estimates about how often AI-generated content was being used in student submissions. Gaining a more accurate understanding of AI's prevalence within student work required substantial data collection, which was still in its initial phases before the widespread use of ChatGPT.
8. **A Wider Scope**: A critical part of developing accurate AI detection was ensuring the training data included a variety of writing styles, genres, and academic fields. This effort aimed to minimize potential biases within the algorithm, but achieving comprehensive representation in the training data was a challenge in the early stages of development.
9. **Shifting Landscape**: The introduction of AI detection tools reflected a broader shift in educational practices towards a re-evaluation of academic integrity. This wasn't just about technology; it involved grappling with evolving ideas about originality and authorship in a digital age.
10. **Keeping it Familiar**: Even in the early stages of development, Turnitin prioritized a user-friendly approach. The AI detection interface was designed to feel familiar to educators already accustomed to the system. This emphasis on user experience aimed to streamline the transition to incorporating AI detection capabilities into their workflows.
Turnitin's AI Detection Accuracy and Limitations in 2024 - AI detection separate from standard similarity scoring
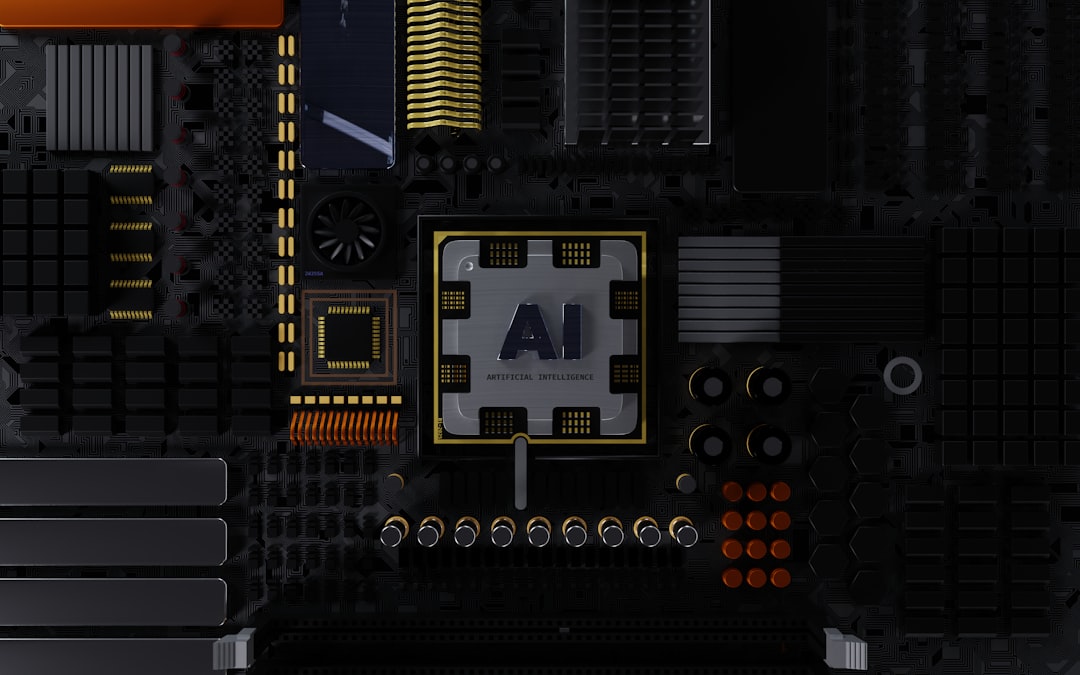
Turnitin's approach to AI detection is unique in that it operates independently from its established plagiarism detection system. This means educators can now assess AI-generated content apart from traditional similarity scores, enabling a more comprehensive understanding of student work. While Turnitin touts a high level of accuracy for its AI detector, the potential for errors, specifically incorrectly identifying human-written work as AI-generated, is a recurring concern. A new AI detection report within Feedback Studio provides more context for interpreting the results, but the need for continued adjustments highlights the ongoing struggle to reliably distinguish human and AI writing. The core challenge persists: AI's continuous improvement necessitates the constant refinement of tools designed to assess its role within academic contexts and maintain integrity in student work.
### Surprising Facts About AI Detection Separate from Standard Similarity Scoring
AI detection tools represent a distinct approach compared to traditional similarity scoring methods. They don't just look for exact matches to existing content; instead, they employ specialized algorithms to analyze text characteristics in a more nuanced way. This means focusing on factors like sentence structure, word choice, and how the language flows.
Interestingly, these tools can be more sensitive to context than basic similarity checks. They try to understand how the text fits within a broader conversation, making them capable of noticing subtle changes in meaning that could suggest AI involvement. But it's not without its challenges. AI detection systems are constantly learning through machine learning, adapting to new AI writing styles they encounter. This ongoing learning process helps improve the accuracy of detection over time, but also adds complexity to the evaluation process.
One of the trickier aspects of AI detection is that sometimes, skilled human writers can unconsciously create text that has patterns similar to AI-generated content. This is something that isn't a concern with traditional plagiarism checks. It introduces the possibility of mistaken classifications, which can be problematic for educators. It appears that the accuracy of AI detection is heavily influenced by the particular AI tool used to create the text. Each AI model generates language in a slightly different way, making some outputs easier to detect than others.
Also, the effectiveness of AI detection isn't consistent across all academic fields. Subjects that rely heavily on structured data, like engineering, might be more prone to generating AI-written content that is easier to detect than more creative fields, like philosophy. This highlights a need for potential discipline-specific detection methods. Even though these tools aim to be objective, it's important to recognize that their training data can have biases, potentially leading to different outcomes depending on the writing style or the background of the author. This could result in certain types of writing being flagged more frequently than others.
Another issue is that AI detection tools seem to produce false positives more often than the standard similarity scoring. This can lead to educators questioning the system's trustworthiness if they see their students' work mistakenly identified as AI-written. Moreover, AI-generated text patterns are constantly changing as AI models evolve. This fast-paced change necessitates that the detection algorithms continuously adapt, presenting a constant engineering challenge for developers. This contrasts with standard similarity scoring, where the core technology is relatively stable.
Finally, the development of AI detection has introduced concerns about its potential for misuse, as educators need to be careful not to over-rely on these tools as the sole indicator of academic misconduct. It's important to maintain a healthy skepticism, and a balanced approach is needed when it comes to assessing the reliability of AI detection as a part of a teacher's toolkit.
More Posts from specswriter.com: