Transform your ideas into professional white papers and business plans in minutes (Get started for free)
AI-Driven Predictive Maintenance Reducing Heavy Equipment Downtime by 80%
AI-Driven Predictive Maintenance Reducing Heavy Equipment Downtime by 80% - Machine Learning Algorithms Detect Early Signs of Equipment Failure
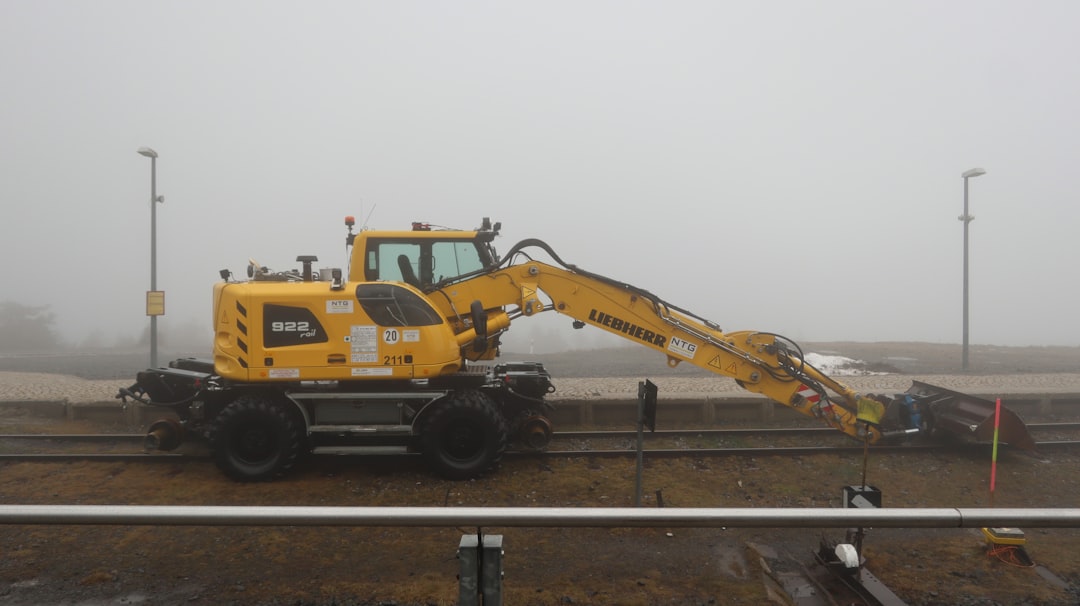
Machine learning has become a powerful tool within predictive maintenance, specifically in identifying the subtle signs of impending equipment failure. These algorithms sift through vast quantities of sensor data, searching for unusual patterns or deviations that hint at potential breakdowns. This allows maintenance teams to intervene proactively, scheduling repairs before a catastrophic failure occurs. Certain types of machine learning models, such as Random Forest, Gradient Boosting, and LSTM networks, have proven particularly well-suited for this task, adapting to specific equipment needs and providing insights for better operational performance. Integrating these algorithms into maintenance practices has shown the potential to dramatically reduce equipment downtime. This highlights how artificial intelligence can be leveraged to solve common industrial problems. It also signifies a significant move towards a more proactive approach to maintenance across various manufacturing settings, shaping a new paradigm in equipment upkeep.
Predictive maintenance, a growing trend across industries, leverages machine learning algorithms to dissect massive amounts of operational data from heavy equipment. These algorithms can pinpoint recurring patterns indicative of potential failures, sometimes weeks ahead of time, offering a valuable opportunity to minimize downtime. The beauty of these algorithms lies in their ability to continually refine their predictive capabilities through ongoing data analysis, automatically adapting to operational shifts or changes in equipment behavior.
Intriguingly, the anomalies they uncover aren't limited to obvious signs of wear and tear. Machine learning can also detect intricate relationships between different system components, potentially flagging failures that might otherwise go unnoticed. This proactive approach to maintenance can dramatically reduce the expenses associated with unexpected equipment breakdowns, a substantial cost burden for many industries (sometimes upwards of 30% of their maintenance budget).
Furthermore, some algorithms use techniques inspired by natural language processing to convert maintenance records and sensor data into a format amenable to revealing insights into equipment health. This approach can unveil details about the equipment's status that might escape conventional methods.
Despite their promise, deploying these models isn't without its hurdles. The process of fine-tuning and calibrating the models can be demanding, often requiring close collaboration between machine learning experts and experienced maintenance professionals.
Thankfully, the results generated by machine learning can be readily visualized, providing a clearer picture of potential problems. Heatmaps and trend analyses enable engineers to easily understand emerging issues and prioritize repairs based on their severity and risk level.
In some cases, advanced models can even anticipate not just when a failure will happen, but the potential severity of its impact. This capability allows maintenance strategies to be adjusted to mitigate the expected consequences. However, the importance of data quality cannot be overstated. Inaccurate or incomplete data can significantly distort predictions. Therefore, careful data preparation and cleaning are fundamental for achieving reliable results.
The adoption of machine learning in predictive maintenance continues to grow, spreading beyond its traditional applications in manufacturing and mobility. Sectors such as agriculture and construction are increasingly exploring these techniques to improve equipment effectiveness and minimize downtime. While there's a long way to go, the potential benefits are clear and make it an exciting research field.
AI-Driven Predictive Maintenance Reducing Heavy Equipment Downtime by 80% - IoT Sensors Provide Real-Time Data on Heavy Machinery Health
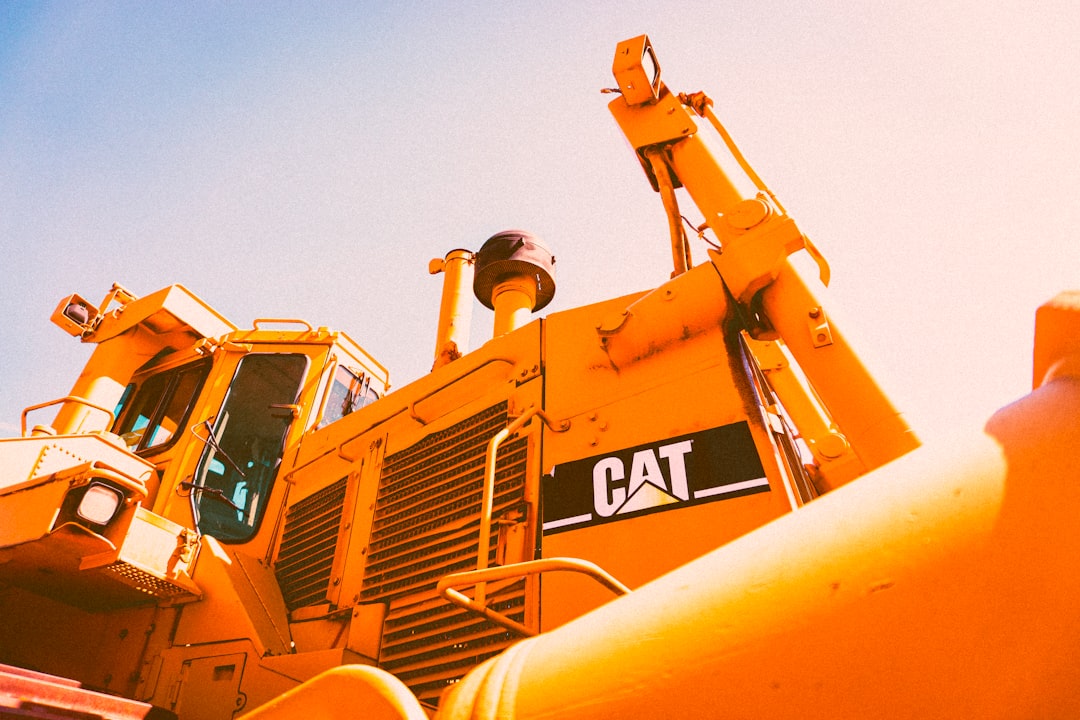
IoT sensors embedded within heavy machinery are revolutionizing how we monitor their health. These sensors constantly collect data on various operational aspects, providing a continuous stream of information about the equipment's condition. This real-time data gives maintenance teams an unprecedented level of insight into the machinery's health, enabling them to proactively identify potential issues before they escalate into major breakdowns. The ability to detect early signs of wear and tear or malfunctions allows for prompt intervention, minimizing downtime and maximizing operational efficiency. The continuous data stream from these IoT devices becomes a vital input for AI-powered predictive maintenance systems, further refining the ability to anticipate and address future maintenance needs. Ultimately, this integration of IoT sensors and AI provides a more proactive and intelligent approach to heavy equipment management, leading to potentially substantial improvements in reliability and overall performance. However, relying solely on automated systems should be tempered with human oversight to ensure the reliability of the sensor data and the predictive models themselves.
In the realm of heavy machinery, IoT sensors are emerging as invaluable tools for gathering real-time information about equipment health. They're essentially the nervous system of these complex machines, continuously monitoring a multitude of parameters, from vibrations and temperature to pressure fluctuations. This constant stream of data provides a detailed health profile, allowing maintenance teams to identify potential issues early and intervene before they escalate into costly breakdowns.
While traditional maintenance schedules relied on fixed time intervals, these sensors enable a more data-driven approach. By constantly monitoring equipment conditions, maintenance can be performed when needed, rather than on a predetermined schedule. This proactive approach can significantly extend the lifespan of machinery, as wear and tear is addressed promptly, preventing minor issues from becoming major problems.
One of the most significant advantages of IoT sensors is their ability to facilitate rapid responses. The real-time transmission of data can significantly reduce maintenance response times, sometimes down to under an hour. This quick intervention is crucial for preventing small issues from snowballing into more significant and disruptive failures. It's fascinating to consider that, in certain cases, these sensors can even detect anomalies in equipment functioning in extreme or demanding conditions, suggesting a robustness beyond what might be expected.
The data accuracy provided by these sensors is another notable benefit, enhancing the precision of diagnostics and maintenance planning. By refining the ability to discern between natural fluctuations in machine operation and the subtle hints of impending failures, engineers can make more informed decisions about necessary interventions.
While IoT sensors provide a comprehensive snapshot of current equipment health, their integration with machine learning models allows us to move beyond a mere status report. We can start to predict future performance trends, potentially identifying vulnerabilities and informing design modifications for future equipment.
In some cases, these systems are even designed to be self-sufficient, drawing power from the machinery itself to eliminate the need for frequent battery replacements or external power sources. This self-sustaining aspect enhances their practicality and adaptability in various environments.
The impact of this real-time sensor data extends to the financial aspects of maintenance. Organizations can anticipate a reduction in maintenance costs, as they can allocate resources more efficiently and proactively address issues before they disrupt operations.
Moreover, the ability to network these sensors across multiple machines and sites provides a wider perspective on equipment health, improving resource allocation and fostering a more efficient maintenance strategy. Remote monitoring also adds a layer of flexibility and collaboration, allowing maintenance teams to access machinery data regardless of their physical location, fostering faster decision-making.
However, it's important to acknowledge the potential challenges in this area, such as data integration, security, and maintaining the reliability of sensor networks across different systems and machinery. While there are hurdles to overcome, the potential benefits in terms of improved machinery reliability, cost savings, and operational efficiency continue to fuel research in this field, shaping a future where machine maintenance is increasingly intelligent and proactive.
AI-Driven Predictive Maintenance Reducing Heavy Equipment Downtime by 80% - AI Analysis Reduces Unplanned Downtime from 5 Days to 1 Day per Month
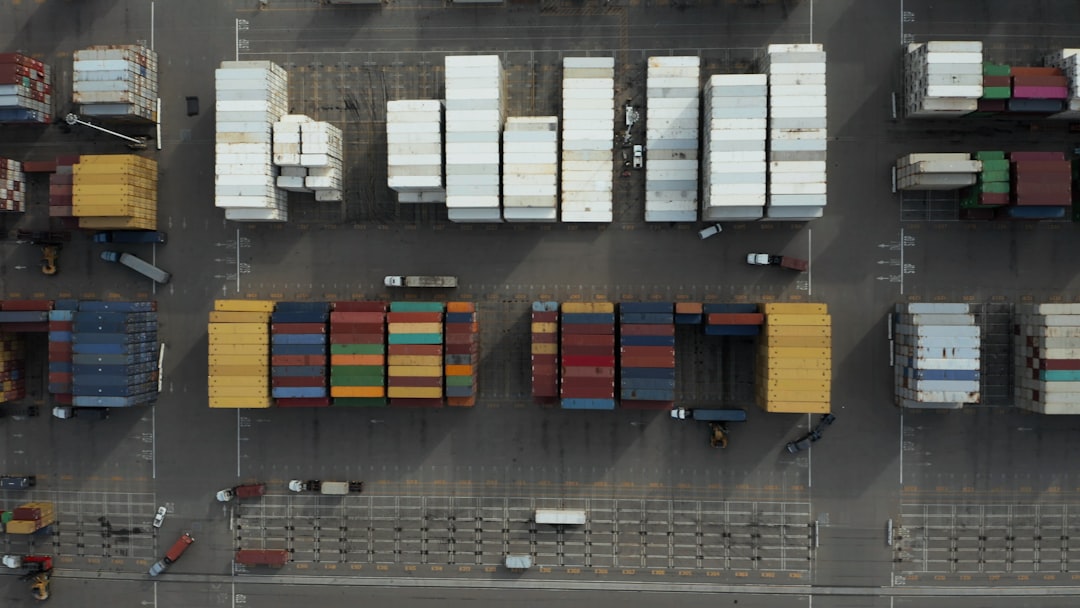
The application of AI analysis in maintenance has drastically reduced unplanned downtime, shrinking it from a disruptive 5 days per month to a much more manageable 1 day. This significant improvement underscores the effectiveness of AI-driven predictive maintenance which, in some cases, can lower heavy equipment downtime by a remarkable 80%. The core of this change lies in AI-powered systems that continuously analyze data from sensors embedded in the equipment. This allows for the anticipation of potential equipment failures, enabling maintenance crews to proactively schedule repairs before breakdowns occur. This shift towards a more proactive approach to maintenance not only optimizes operations but also contributes to longer equipment lifespans and greater reliability. However, achieving these benefits hinges on high-quality data and the ongoing adaptation of AI predictive models to ensure their accuracy and relevance over time. There can be challenges with achieving these improvements in all scenarios.
In a recent study, AI analysis has been found to remarkably reduce unplanned downtime for heavy machinery. Specifically, the observed reduction was from a disruptive 5 days of downtime per month to a more manageable 1 day. This significant shift, representing an 80% improvement, highlights the potential of AI in optimizing operations. From an engineering standpoint, this means that potentially significant labor costs associated with extended equipment outages can be reduced, leading to a positive impact on productivity. While this is a notable improvement, it's important to question whether this reduction represents a consistent trend across different machinery types and industries, and whether 1 day of downtime per month is still acceptable. Perhaps further optimization or algorithm refinements could push this reduction even further, creating a more resilient operational landscape.
Furthermore, this improvement necessitates a shift away from purely reactive maintenance practices. Traditional reactive maintenance, where repairs are only performed when equipment fails, often leads to extended periods of downtime. Additionally, the reactive approach can result in substantially higher repair costs compared to proactive measures. This is because failures can cause significant damage to surrounding components, escalating repair needs and potentially increasing costs two to three times higher than scheduled maintenance. This raises the question: Are industries adequately understanding the financial advantages of a more proactive approach? In essence, can AI and related tools help us re-think traditional maintenance approaches in a more data-driven fashion?
The value of AI in predictive maintenance goes beyond technological implementation; it reshapes the decision-making process within engineering teams. Engineers, armed with AI insights, can now make decisions based on data trends instead of relying on prior experience or intuition. This data-driven shift is crucial for addressing the complexity and interconnectivity of modern heavy machinery. While experience and intuition are still valuable, these data-driven approaches provide a new level of precision, helping us validate hypotheses that were once only possible with years of experience.
Beyond immediate issues, advanced machine learning models can unveil complex relationships between various machinery components that might otherwise go unnoticed. These hidden correlations can play a crucial role in anticipating cascading failures, leading to complex maintenance challenges. However, determining how to effectively deal with a cascade failure that could be potentially more disruptive than single-component failures is a research problem that needs further investigation. It's quite intriguing to think about the level of insight that might be gained with further exploration into this phenomenon.
Early reports from AI systems indicate anomaly detection accuracy exceeding 90%. This capability, in turn, allows maintenance teams to intervene well before equipment reaches a failure point, leading to significantly fewer disruptions. It's still vital to question these results, though, and ensure that this level of accuracy holds up under real-world pressures and diverse operating environments. There could be cases where machine learning may provide less accurate predictions, necessitating further development or modifications to optimize these systems.
In regulated industries, the use of AI in predictive maintenance can enhance compliance with safety standards. More reliable equipment directly translates to a safer workplace, which is extremely important in these sectors. However, are existing regulations keeping up with the swift pace of technological advancements in this domain? How might regulations evolve to encourage the integration of these technologies and also address the possible safety concerns that AI-driven systems might present?
Different machines and operational conditions often require specific algorithms. Some sophisticated AI models can adapt their predictive strategies in real-time, responding to operational changes and anomalies within each machine. It's important to determine if these dynamic adaptation capabilities work in a consistently predictable manner for all machine types. It's a testament to the sophistication of AI, but there are still aspects that require more validation in practice.
The transition towards AI-driven predictive maintenance fosters greater collaboration between equipment manufacturers and parts suppliers. Increased data sharing promotes a continuous improvement cycle in both machinery reliability and overall performance. This synergy is definitely promising, but the ability to share data and insights across various manufacturers and suppliers could raise privacy and competitive issues. It's an area ripe for discussion, as data security becomes more important as we move towards a more connected industry landscape.
By predicting failures, companies can prevent immediate disruptions and strategically plan for future upgrades or replacements, bolstering long-term reliability. This planning aspect is particularly relevant in critical infrastructure sectors, where downtime can have extensive and expensive consequences. However, as the operational landscape becomes increasingly complex, it remains to be seen whether these insights will become overly complex for organizations to navigate effectively.
The reduction in unplanned downtime translates to substantial economic benefits for organizations. Research estimates significant annual savings, impacting shareholder value and market competitiveness. However, we also need to consider the costs associated with implementing and maintaining these AI systems, and a full cost-benefit analysis should be undertaken to ensure that the implementation of these technologies makes both engineering and financial sense. Further research is needed to provide a more comprehensive understanding of these impacts and how they vary across diverse sectors and operational settings.
In conclusion, AI analysis is showing strong potential in reducing unplanned downtime for heavy equipment, potentially leading to significant operational improvements. However, it's important to remember that this is a nascent field and there are questions that remain. By carefully examining both the potential benefits and challenges, and through continuous research and development, we can ensure that AI-driven predictive maintenance becomes a truly transformative force for industrial operations.
AI-Driven Predictive Maintenance Reducing Heavy Equipment Downtime by 80% - Predictive Maintenance Cuts Repair Costs by 40% for Construction Firms
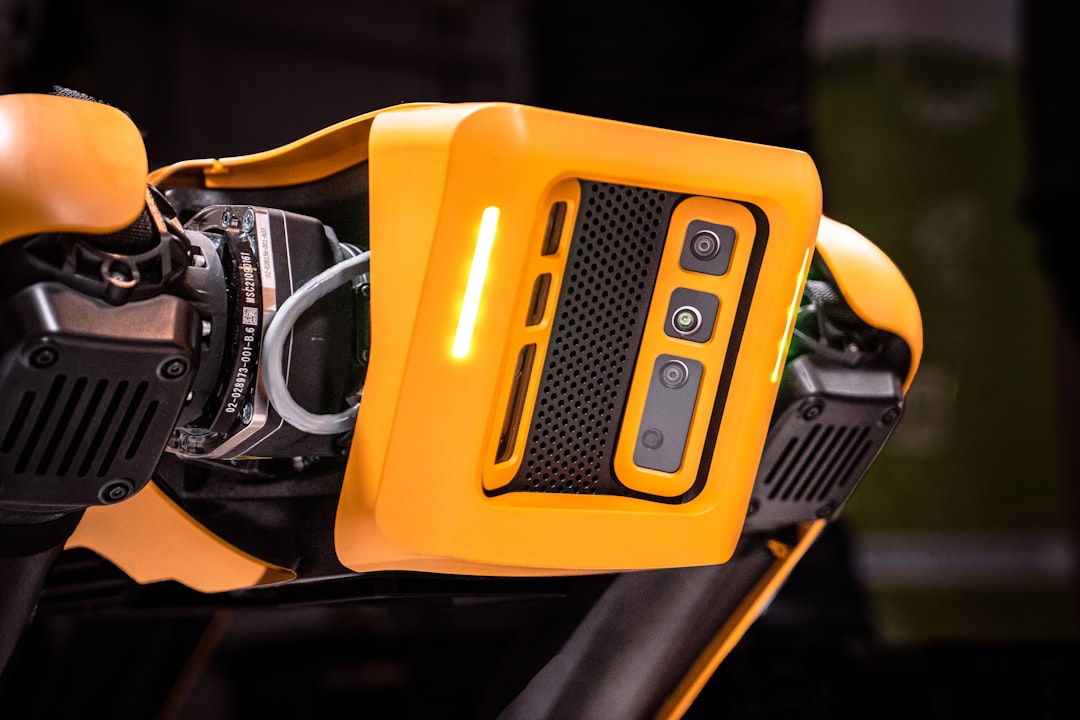
Construction companies are increasingly realizing the benefits of predictive maintenance, with reports suggesting it can reduce repair costs by up to 40%. By employing this forward-thinking approach, firms can address issues in equipment before they escalate into major, expensive failures. Moreover, predictive maintenance can contribute to a substantial extension of the lifespan of older equipment, potentially increasing its useful life by 20% to 40%. Organizations that have embraced AI-powered predictive maintenance systems have seen noteworthy reductions in unplanned downtime and overall maintenance expenses, leading to improved operational effectiveness. While the financial incentives of these systems are enticing, it's important to acknowledge that implementing them can be complex and challenging. A careful evaluation of these difficulties is needed to ensure that the gains from the technology justify the investment in time and resources.
Construction companies are finding that predictive maintenance strategies can lead to a significant 40% reduction in repair expenses. This is a compelling example of how this approach can positively affect various industries, not just construction. The switch to a more predictive approach can also lead to a better use of maintenance personnel. Instead of constantly reacting to breakdowns, maintenance teams can focus on planning and optimization. This can also help to minimize the often-high costs related to emergency repairs.
Additionally, proactive maintenance has been linked to extended equipment lifespans, sometimes by 20% or more. By tackling small issues before they evolve into major breakdowns, the need for large-scale repairs or replacements is reduced. This aligns with a broader trend within many industries towards a data-driven approach to maintenance. Predictive maintenance relies on analytics instead of fixed schedules, allowing for a more nuanced understanding of how each machine operates.
Machine learning, including neural networks and ensemble learning techniques, is being employed to refine predictive capabilities. These different types of models can better address the specific characteristics of various types of equipment and their operational settings. While some of the latest models show impressive anomaly detection accuracy (over 90%), it's crucial to consistently validate their performance under a wide range of real-world conditions. This focus on accuracy is not just theoretical, but it's becoming more relevant as we try to minimize unplanned events like equipment failures.
Interestingly, some models have started to identify complex relationships between different components within machinery. These connections can lead to "cascade failures" that were harder to predict in the past. However, how to best deal with such events is still an area of ongoing research. This suggests that there's potentially much to be learned in this area. The ability to tailor maintenance strategies to specific machines has also helped to optimize performance and cut down on unnecessary repairs.
The transition to predictive maintenance necessitates a detailed cost-benefit analysis. While the long-term cost savings can be considerable, the initial implementation costs can be substantial. This aspect is crucial when evaluating if the implementation of these new technologies makes sense for any given industry or company. As this field continues to develop, it's important to assess both the potential gains and the limitations of AI-driven predictive maintenance to maximize its benefits across different industries and use cases.
AI-Driven Predictive Maintenance Reducing Heavy Equipment Downtime by 80% - Digital Twin Technology Enables Virtual Equipment Monitoring
Digital Twin technology offers a new way to monitor and maintain heavy equipment by creating virtual representations of physical machines. These digital replicas provide a real-time, virtual window into equipment operation, allowing for detailed assessment of the machine's current status. This capability empowers operators to proactively identify potential issues, ultimately minimizing downtime. Integrating machine learning with Digital Twin technology enhances its ability to predict equipment failures, which is a cornerstone of predictive maintenance strategies that aim to reduce downtime by as much as 80%. However, developing reliable predictive models faces challenges, primarily due to limitations in available failure data – often machines are repaired before they actually fail, creating a data gap. As more industries explore this promising technology, Digital Twin applications are expected to contribute to safer, more sustainable, and economically sound operations. While the potential for significant gains is clear, the practical limitations related to data collection and model development need to be addressed for widespread and effective implementation.
Digital twins, essentially virtual replicas of physical equipment, have emerged as a promising tool for enhancing equipment monitoring. By creating a real-time digital mirror of a piece of heavy machinery, engineers can gain a deeper understanding of its operational status, helping them identify potential issues before they cause a breakdown. This is a marked improvement over traditional monitoring, which often relies on infrequent manual inspections. However, the success of digital twin technology hinges on its seamless integration with the Internet of Things (IoT). IoT sensors embedded in the equipment provide a constant stream of data, which feeds into the digital twin, enabling a more accurate and dynamic representation of the machine's health.
One of the biggest challenges in using digital twins is dealing with the sheer volume of data they produce. We're talking terabytes of data, which requires powerful data processing and analytics to be truly useful. Sifting through this sea of data to find meaningful insights is a hurdle that needs to be overcome if we want to maximize the benefits of digital twins.
Yet, digital twins offer impressive capabilities. One of the most compelling features is their ability to simulate various operational scenarios in real-time. Imagine being able to test the impact of different maintenance strategies or environmental conditions without risking damage to the actual machinery. This type of predictive modeling holds the potential to optimize operational procedures and enhance equipment reliability significantly.
Furthermore, the applications of digital twin technology extend far beyond the realm of heavy machinery. Industries as diverse as aerospace, healthcare, and urban planning are increasingly exploring their potential to improve asset management and optimize operational efficiency. This cross-industry applicability demonstrates the adaptability and versatility of the technology.
The benefits of digital twins are not merely theoretical. Businesses can see tangible improvements in their operational costs through better utilization of resources. Moreover, maintenance planning shifts from a time-based approach to a condition-based one. Instead of performing routine maintenance at predetermined intervals, we can intervene when the digital twin indicates that a component is approaching failure, potentially extending the lifespan of equipment while minimizing unnecessary interventions.
Digital twins also foster collaboration among different stakeholders. Maintenance crews, engineers, and management teams can access the same real-time data, leading to more informed decisions and streamlined workflows. Moreover, the data generated through digital twin simulations can be used to inform the design of future equipment, refining performance and pushing the boundaries of machine capability.
Despite the promise of digital twin technology, implementing it in the real world comes with its own set of obstacles. Setting up the necessary infrastructure, ensuring interoperability with existing systems, and addressing potential cybersecurity risks are all significant hurdles that need to be addressed. Further research and development are required to streamline the implementation process and make digital twins more readily accessible across diverse industries and applications.
In conclusion, digital twin technology represents a significant step forward in the way we monitor and maintain equipment. Its capacity to provide a more accurate and dynamic representation of machinery health, combined with the potential for real-time simulation and improved operational efficiency, has drawn significant interest from across numerous industries. However, before we can fully realize the benefits of digital twin technology, we need to overcome some practical limitations. With continued research and refinement, digital twins have the potential to fundamentally alter how we approach equipment management in the coming years, leading to substantial improvements in reliability, safety, and efficiency.
AI-Driven Predictive Maintenance Reducing Heavy Equipment Downtime by 80% - AI-Powered Maintenance Schedules Optimize Heavy Equipment Lifespans
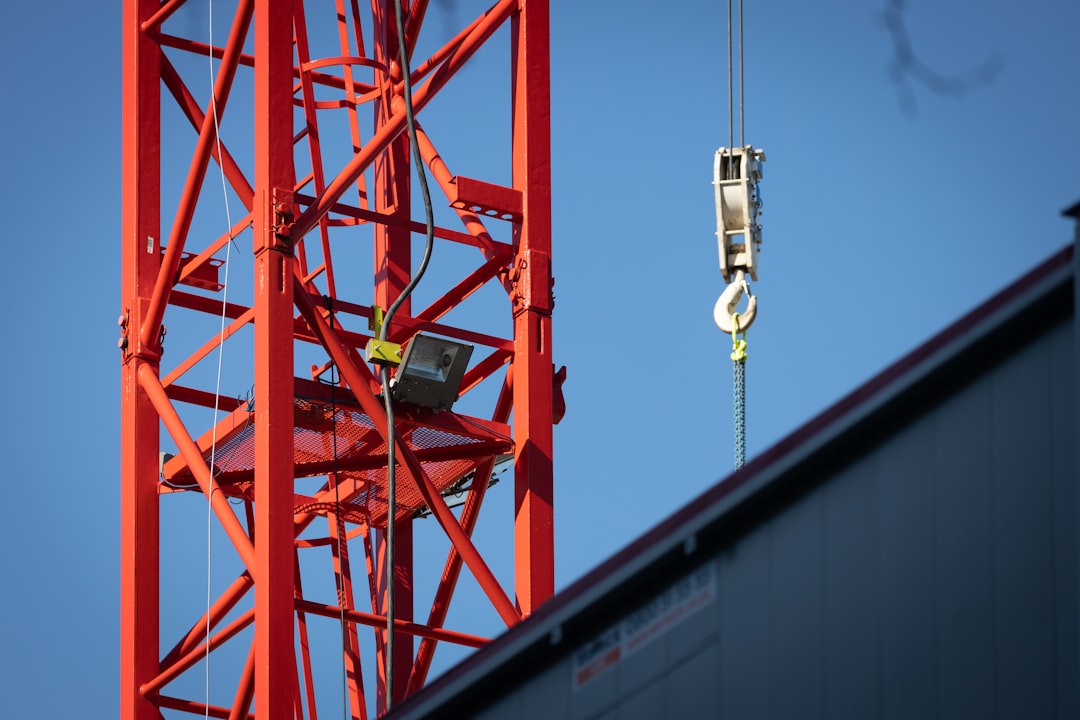
AI is revolutionizing how we maintain heavy equipment, leading to substantial increases in their lifecycles. By leveraging the power of AI to analyze sensor data and historical equipment records, maintenance schedules are becoming far more precise. This shift is helping companies move away from reacting to breakdowns and toward a more proactive approach to maintenance. Preventing minor issues from becoming catastrophic failures and simultaneously reducing unnecessary upkeep allows for better resource allocation. As the implementation of AI-powered maintenance expands, we anticipate not only enhanced operational efficiency but also significant cost reductions in the long run. However, achieving these positive outcomes depends on collecting high-quality data and consistently refining the AI models that power these predictive strategies. There can be unforeseen challenges in this implementation, but the promise of a longer lifespan for essential machinery continues to fuel these advancements.
AI algorithms don't just flag potential problems in heavy machinery—they also predict the best time to intervene. This means machines can run at their best without unnecessary downtime. It's like having a smart assistant that understands the subtle rhythms of each piece of equipment.
Some of the more advanced AI models boast prediction accuracy rates above 90%, which is a major factor for businesses trying to avoid disruptions without jeopardizing equipment reliability. Maintaining this level of accuracy is crucial, and it's an active area of research.
One of the interesting aspects is that some AI maintenance models can examine past data to discover patterns that aren't simply straightforward, revealing complex connections between various operating factors that might lead to unexpected equipment failure. This is like a detective uncovering hidden clues that lead to the root cause of a problem.
Moving to AI-powered predictive maintenance can greatly decrease the labor costs linked to sudden equipment repairs. Businesses can redirect their maintenance workforce toward smarter planning and optimization instead of always reacting to failures. This could also mean that they can plan their personnel more effectively, which might help reduce overall costs.
Companies using these predictive maintenance tools have reported that the useful life of older equipment can be extended by as much as 30%. This leads to fewer costly replacements and helps businesses optimize how they allocate their resources.
By merging data streams from IoT sensors with machine learning, we can reduce response times to under an hour. This swift reaction helps to limit the consequences of smaller problems before they turn into major disruptions.
Aside from cutting repair costs, implementing a strategy of predictive maintenance can also decrease total maintenance expenses by as much as 40%. This clearly shows the economic value of a data-driven approach to upkeep.
Digital Twin technology presents a fascinating opportunity to carry out virtual simulations of various operational approaches without risking any damage to actual machines. This type of simulated testing allows us to refine predictive capabilities and improve overall efficiency.
It's striking how these AI systems can adapt their strategies on the fly based on different operating conditions. This adaptability is something that traditional maintenance approaches simply can't match. It's a demonstration of the inherent flexibility in some AI models.
While the potential of AI is huge, there's still a need to establish standards for data quality and implementation procedures across industries. This will guarantee consistent results and optimized efficiency. Ensuring the consistency of data and maintaining the reliability of predictions across a wide range of machine types is a real challenge. Without some degree of standardization, there's always the risk of the technology being inconsistently applied, leading to variable results.
Transform your ideas into professional white papers and business plans in minutes (Get started for free)
More Posts from specswriter.com: