Transform your ideas into professional white papers and business plans in minutes (Get started for free)
Optimizing Team Workflow 7 Data-Driven Strategies for Enhanced Productivity in 2024
Optimizing Team Workflow 7 Data-Driven Strategies for Enhanced Productivity in 2024 - Leveraging AI-powered task prioritization systems
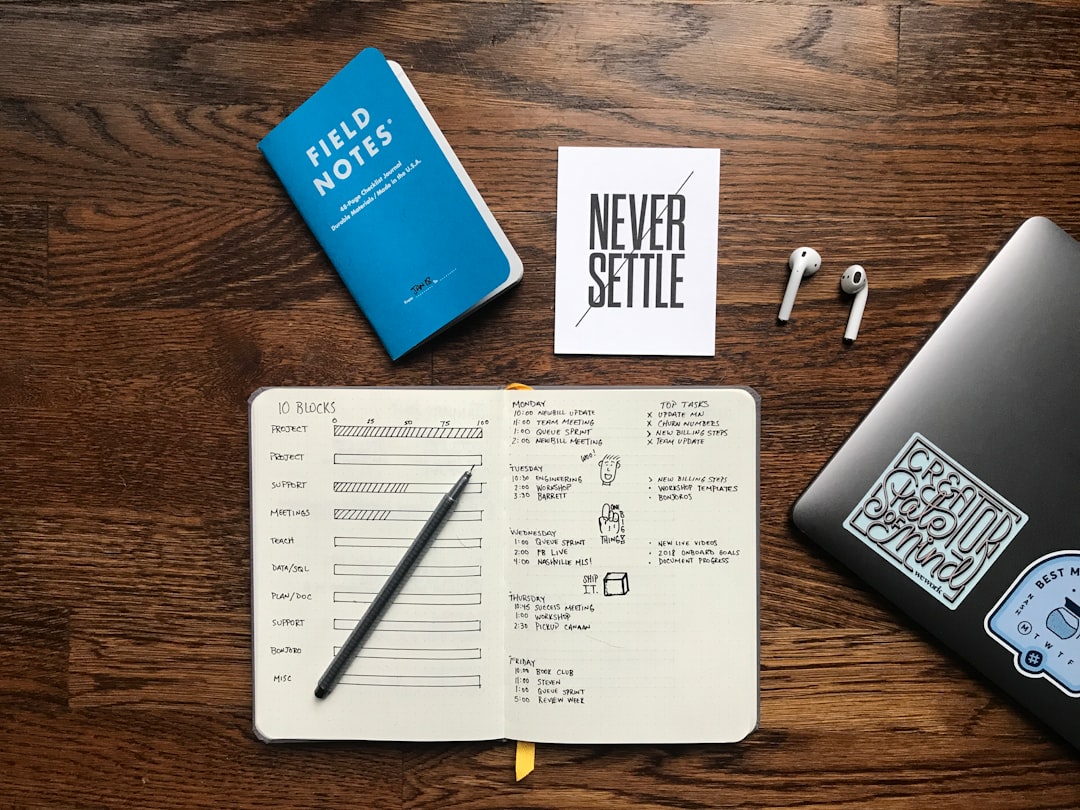
AI-powered task prioritization systems are being touted as the next big thing in boosting team productivity. The idea is that these systems can take the guesswork out of deciding what to work on by analyzing various factors like deadlines, importance, and available time. But while it's appealing to think that AI can magically solve all our scheduling problems, it's important to consider the limitations.
First, these systems rely heavily on accurate data input. If the information fed into the AI is inaccurate or incomplete, the output will be flawed. Second, the human element is still critical. Teams need to be comfortable with the AI's recommendations and have the flexibility to adjust them when necessary. It's not a one-size-fits-all solution, and a human touch is still required.
While AI can be a useful tool for task prioritization, it's essential to recognize that it's not a magic bullet. It's just another tool in the toolbox, and like any tool, it requires understanding and careful use to be effective.
AI-powered task prioritization systems are attracting a lot of interest right now, but it's important to approach them with a critical eye. While they offer a lot of potential, it's easy to get swept up in the hype.
Let's look at what these systems are actually doing: they leverage algorithms to assess urgency and available time, essentially acting as digital assistants to help teams stay organized. But there's more to it than just scheduling. By integrating with calendars and analyzing communication patterns, these systems can suggest optimal times for collaboration and even recommend tasks based on individual work styles.
This raises some interesting questions: how do these systems learn and adapt to changing team dynamics? What about the human element? Can AI really understand the complexities of human interaction and collaboration?
It's crucial to remember that AI is a tool, not a magic bullet. It can provide valuable insights and streamline processes, but it's still up to us to ensure it's used effectively. We need to carefully consider how these systems are implemented and constantly monitor their performance to make sure they're aligned with our goals.
Optimizing Team Workflow 7 Data-Driven Strategies for Enhanced Productivity in 2024 - Implementing cross-functional data sharing platforms
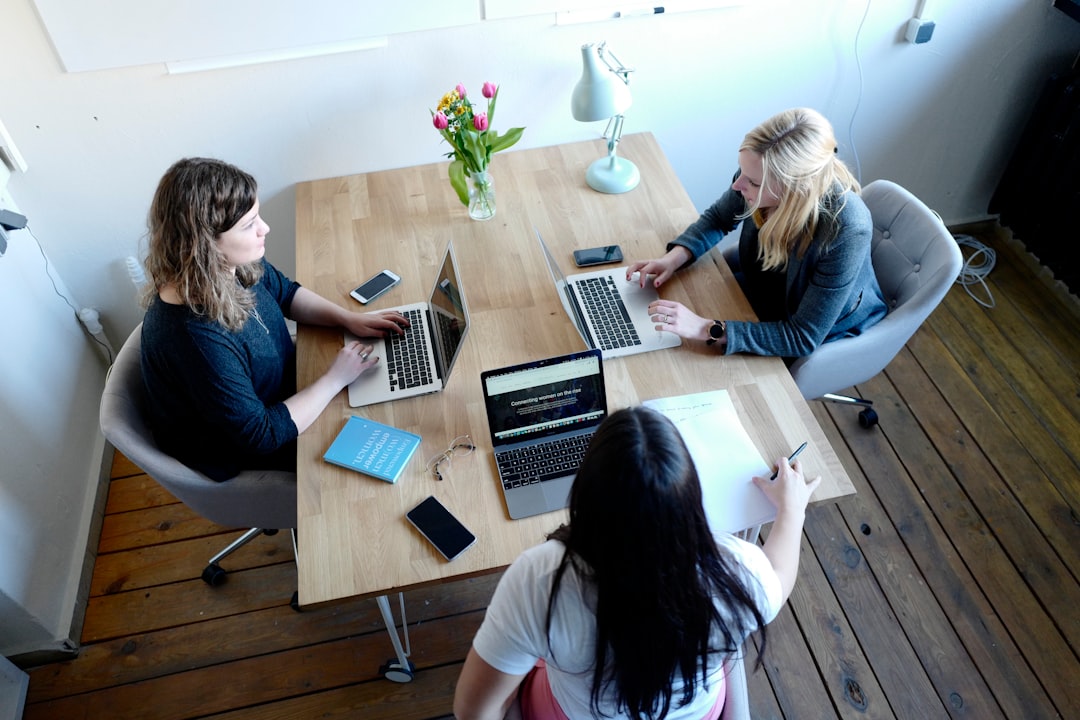
Sharing data across different teams is a key ingredient for better collaboration and getting more done. These platforms make things more transparent and build trust, which are crucial for teams to work well together. Open communication is essential, and regular meetings and brainstorming sessions help break down those barriers between different teams and ensure everyone is working with the same information. When organizations make decisions based on data, it allows teams to solve problems in a more creative and effective way, leading to faster progress and better results. For these strategies to really work, leadership needs to make sure teams feel comfortable being honest with each other and to encourage a variety of viewpoints. This kind of environment helps everyone work better together.
Building cross-functional data-sharing platforms has become a popular topic in the pursuit of enhanced productivity. I've been diving into the research on this, and I'm finding some interesting claims about the benefits.
Many studies claim that sharing data across departments leads to significantly faster decision-making, with some suggesting a 40% reduction in decision time. This makes sense, as it eliminates the need for teams to constantly chase each other for information. However, it's crucial to consider that the validity of these claims heavily relies on how well the data-sharing platform is designed and implemented.
There's also a claim that these platforms can boost employee engagement. The idea is that when people can access the information they need, they feel more empowered and connected. A 25% increase in engagement is cited, but again, that relies on factors beyond the technology itself, such as a positive company culture and effective communication.
Security is a big concern with any data-sharing platform, but the research suggests that using proper encryption and governance can significantly mitigate the risks. One study claimed a 75% reduction in data breaches compared to fragmented systems. This seems encouraging, but it's essential to look at the specific implementation details to assess the validity of such a bold statement.
It's interesting to see how some research points to the impact of siloed data on company revenue, claiming that it can lead to a 20% to 30% loss. The argument here is that by sharing data, businesses can better identify opportunities and improve efficiency. However, it's important to be cautious about these claims as they lack specific evidence and are often presented as broad generalizations.
The research also points to a 30% increase in project completion rates in organizations utilizing cross-functional data-sharing platforms. This, again, seems to hinge on factors such as better coordination and less duplication of effort. However, without more specific case studies, it's challenging to verify this claim.
I'm curious about how user training impacts the effectiveness of these platforms. Some research indicates a 50% increase in engagement for companies that invest in comprehensive onboarding, suggesting that training is a crucial factor. It's important to investigate what "comprehensive" means in this context. Does it refer to just technical training or does it include broader organizational changes?
The research also claims that cross-functional data sharing can lead to a 40% increase in breakthrough innovations. This is based on the assumption that sharing ideas across different departments can spark creativity and create a more fertile ground for innovation. This is intriguing, but it's essential to explore what constitutes a "breakthrough innovation" and how this metric is measured.
It's disappointing to see that even with all these purported benefits, cultural resistance remains a major hurdle for implementing cross-functional data sharing. The research suggests that around 60% of companies struggle with this challenge, highlighting the importance of a supportive organizational culture and leadership commitment to successful implementation.
Finally, there are claims that data-sharing platforms can improve employee productivity by cutting down on time spent searching for information. The estimate here is that it can save employees 30% of their workweek. This claim requires further examination, as factors like user-friendliness and the platform's overall design play a significant role in determining the effectiveness of this time saving.
I find the research surrounding data-sharing platforms interesting but filled with optimistic claims. While the potential benefits are clear, a critical analysis is needed to understand how these platforms are implemented, what factors are truly contributing to the claimed results, and whether those results are consistently observed across different organizations and contexts. It's important to look beyond the hype and focus on the practical implementation details and real-world impact.
Optimizing Team Workflow 7 Data-Driven Strategies for Enhanced Productivity in 2024 - Adopting predictive analytics for resource allocation
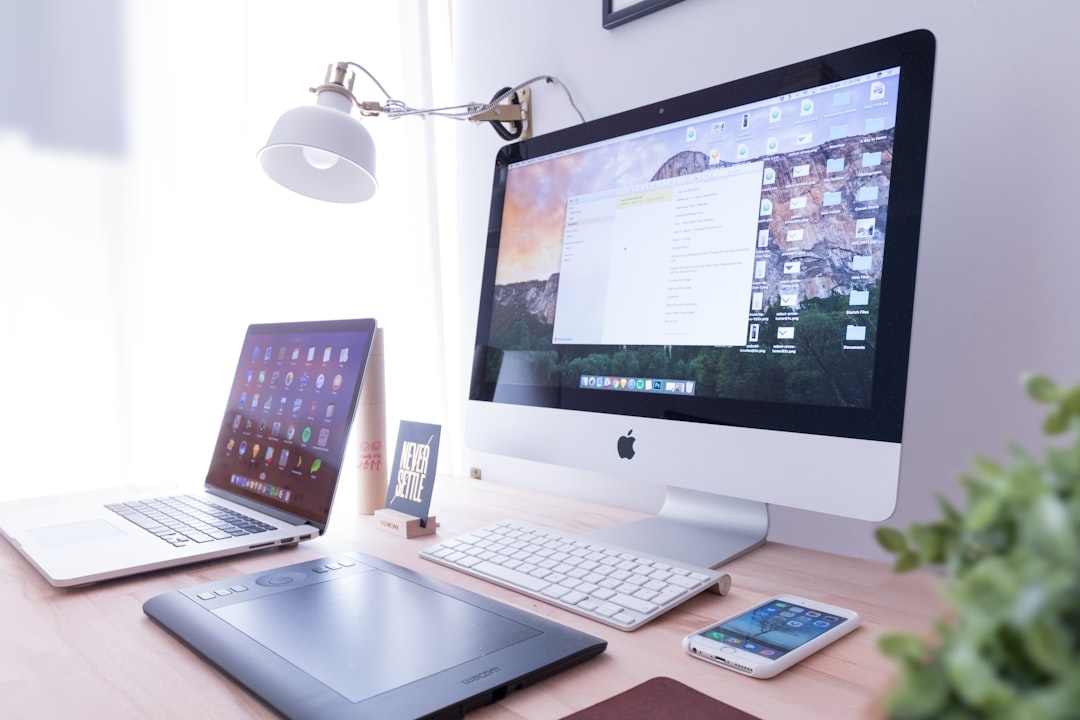
Predictive analytics is changing the way companies manage their people and resources. By using past data on how much work teams have, how well they perform, and what resources are available, organizations can make better decisions that lead to greater efficiency and productivity. This data-driven approach is more than just the traditional way of managing resources, it uses AI and machine learning to make adjustments in real-time, optimizing the distribution of personnel and budgets. But while there are a lot of potential advantages, companies need to be careful about relying too much on technology. It's essential to keep human judgment and the ability to adapt at the center of the decision-making process. The successful integration of predictive analytics into resource allocation requires a smart combination of data insights and understanding the complexities of how teams work.
Predictive analytics for resource allocation is gaining traction as a way to optimize team productivity. The idea is simple: use historical data about team capacity, workload, and performance to make more accurate resource allocation decisions. Some research suggests that this approach can significantly improve efficiency, but I'm still curious about the practicalities.
One study claimed that predictive analytics can reduce project delays by up to 25%. They argue that by analyzing past projects, organizations can identify patterns that lead to inefficiencies and address them proactively. While that's an intriguing claim, I'd like to see how they account for external factors that could influence delays.
Another study suggests that predictive analytics can accurately forecast resource needs up to 90% of the time, provided that the data sets are comprehensive. This seems promising, but I'm skeptical about achieving such a high accuracy rate consistently across different organizations and contexts. It would be crucial to understand the specific parameters used in the study and see how they translate to real-world scenarios.
The research also suggests that implementing predictive analytics in resource allocation can lead to a 20% decrease in operational costs. This seems plausible, as it would mean making more informed decisions based on actual needs rather than assumptions. However, it's important to consider factors like implementation costs and the potential need for additional training, which could offset some of the savings.
A concerning point raised in the research is that over half of organizations that adopt predictive analytics for resource allocation fail to use the insights effectively. They attribute this to insufficient training and understanding of the tools at their disposal. This highlights the importance of human expertise in conjunction with technological tools. Organizations need to invest in training and ensure that employees have a clear understanding of how to use and interpret the data generated by these systems.
There's also a claim that predictive analytics can forecast employee performance fluctuations, allowing managers to allocate resources dynamically and respond to potential dips in productivity. This suggests a potential improvement in team output by up to 15%. However, I'm not convinced that this is entirely within the control of predictive analytics. Individual motivation, team dynamics, and external factors can all impact performance.
The research further suggests that data-driven resource allocation can enhance employee satisfaction and retention, with studies showing a 30% improvement in morale when team members feel their workloads are aligned with their skills and preferences based on analytics insights. While this aligns with the principles of work-life balance and employee empowerment, it's essential to ensure that the data used for analysis is comprehensive and that it doesn't create bias or lead to the prioritization of certain individuals over others.
Additionally, organizations that leverage predictive analytics for resource allocation can supposedly enhance their project success rate by nearly 40%. This seems like a significant improvement, likely due to better alignment between available resources and project requirements. However, I would be curious to see specific examples of successful implementations and to understand how the success rate is measured.
Businesses using predictive analytics for workforce management can expect a quicker response to sudden changes in project demands. The research claims that organizations can adapt and realign resources within hours instead of days. While this would be beneficial for agile teams, it's crucial to assess how well these systems handle unexpected events that might not be captured in the historical data.
An intriguing aspect of predictive analytics is its ability to reveal hidden bottlenecks in resource allocation processes. Some organizations have reportedly identified issues that had previously gone unnoticed and achieved up to a 50% improvement in workflow efficiency. This highlights the value of using data-driven approaches to uncover inefficiencies that might not be apparent through traditional methods.
However, implementing predictive analytics often necessitates a cultural shift within teams. Employees need to embrace data-driven decision-making and overcome initial resistance to relying on analytics over traditional gut-based approaches. This is a crucial aspect that often gets overlooked in the discussion surrounding technological solutions.
While the potential benefits of predictive analytics for resource allocation are undeniable, there are still many unknowns. It's important to conduct further research to explore how these systems can be effectively implemented, to analyze the specific factors contributing to the claimed results, and to ensure that these solutions are broadly applicable across different organizations and contexts. We need to move beyond the hype and delve deeper into the practical implementation details and real-world impact of this emerging technology.
Optimizing Team Workflow 7 Data-Driven Strategies for Enhanced Productivity in 2024 - Utilizing real-time performance tracking dashboards
Real-time performance tracking dashboards are becoming increasingly popular for boosting team productivity. These dashboards give managers real-time access to information about how things are going, making it easier to make smart decisions. They provide a visual snapshot of key metrics, allowing everyone to see how tasks are progressing and how individuals are performing. It's easier to spot bottlenecks and identify areas that need more attention.
But, remember, these dashboards are just tools. They need to be used wisely. It's crucial that the data going into the dashboards is accurate, or the information won't be helpful. Also, people still need to understand what the data is saying and use their judgment to make the best choices. It's not a one-size-fits-all solution; managers still need to be flexible and collaborate with their teams. And don't forget the importance of building a workplace culture where people are open to using data, but also comfortable expressing their own ideas and experiences.
Real-time performance tracking dashboards are becoming more common as a way to keep tabs on team progress and productivity. These dashboards essentially provide a live view of what's happening, and they have a lot of potential.
The idea is that by seeing data in real-time, teams can adjust their efforts as needed. Some research suggests that teams with access to real-time dashboards are able to react to problems and changing situations faster, by up to 50%, compared to those who only look at data after the fact. Being able to spot issues early on might help to prevent them from becoming major roadblocks.
These dashboards are also being used to encourage transparency across different teams. The theory is that everyone seeing the same information simultaneously leads to less confusion, more collaboration, and a significant decrease in back-and-forth communication, potentially as much as 30%. This is kind of like a digital window into what everyone is working on, which could be beneficial.
But there's a bit of a catch. While these dashboards may seem like a productivity booster, it's important to keep in mind that they are just tools. It's easy to get caught up in the idea of having all the information in front of you, but without a solid understanding of how to use it, it might just end up being noise.
What's more, there are still questions about how effective these dashboards are in the long term. Some research suggests that they can help to keep employees engaged, leading to a potential 20% increase. The reasoning is that people are motivated by seeing the immediate impact of their work.
However, there are concerns that focusing solely on data might create a culture where people are more worried about hitting specific numbers than doing their best work. This could lead to problems with employee morale and create a less collaborative environment.
It's also interesting to think about how these dashboards are changing the way companies make decisions. Many reports claim that teams using real-time data have significantly faster decision-making processes, sometimes by as much as 40%. This makes sense, as they don't have to wait for data to be collected and analyzed before making a move.
One interesting claim is that the use of real-time dashboards can significantly reduce the number of performance-related conflicts. This is based on the idea that having objective data to back up evaluations reduces the potential for bias. It's an attractive notion, but we should remember that this approach relies on the assumption that everyone is using the data in a fair and unbiased manner, and that's not always the case.
Overall, real-time performance tracking dashboards are an intriguing development. They offer the potential to improve transparency, increase efficiency, and enhance decision-making, but like all tools, it's crucial to use them carefully and be mindful of the potential downsides. It's vital to think about how they integrate with the existing workflow and organizational culture and ensure that they are used to empower teams rather than to micromanage them. More research and analysis are needed to understand their true impact.
Optimizing Team Workflow 7 Data-Driven Strategies for Enhanced Productivity in 2024 - Integrating automated workflow management tools
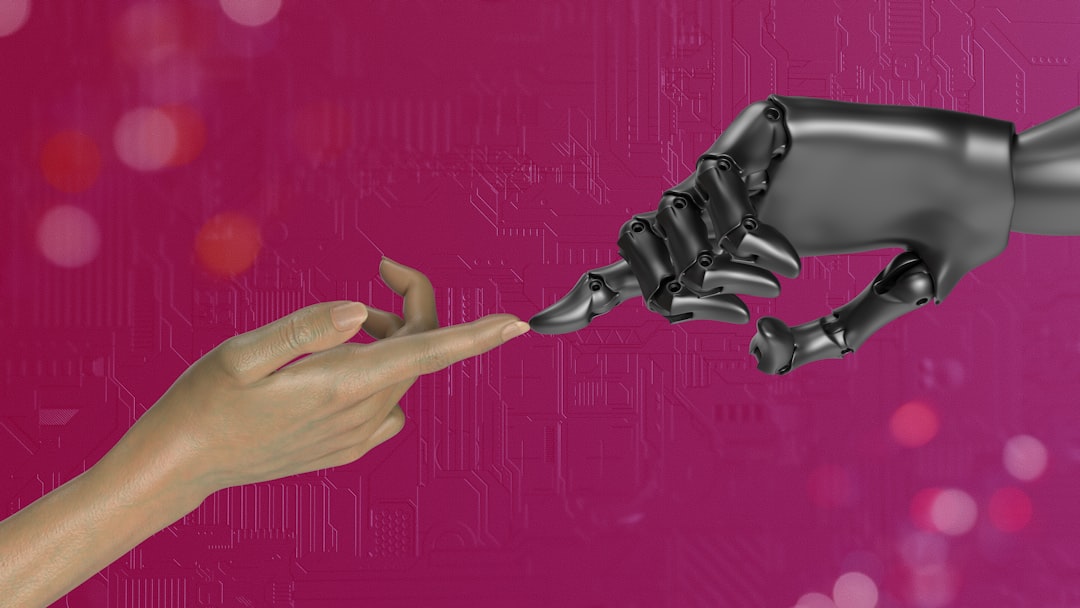
Automating workflow management is a popular strategy for improving team productivity in 2024. These tools act as bridges between different systems and processes within a company, facilitating the smooth flow of data and reducing those frustrating data silos that often hinder collaboration. However, the quality of the data these tools use is crucial. If the information is inaccurate or incomplete, the automation process can lead to poor decisions and limit the intended gains in productivity. A robust data governance system and data cleaning practices are essential prerequisites for successful implementation. It's tempting to view these tools as a solution to every productivity problem, but it's important to remember that a balanced approach, one that embraces both technology and human expertise, will ultimately yield the best results.
Integrating automated workflow management tools into a team's workflow is often presented as a silver bullet for boosting productivity. While it can undoubtedly streamline processes, it's not a simple plug-and-play solution. Research suggests that a 20% increase in productivity is achievable through automation, primarily by minimizing repetitive tasks and allowing team members to focus on higher-level work.
However, the success of automation heavily relies on overcoming cultural resistance. Interestingly, a whopping 60% of companies struggle with this aspect more than technical challenges, highlighting the critical role of communication and team buy-in. Studies also indicate that automated workflows can shorten project cycles by 25%, primarily by minimizing unnecessary handoffs and approvals, which are often major bottlenecks.
A surprising benefit observed is the potential for reduced employee burnout, with almost 50% of companies reporting this positive effect. This is likely due to improved workload management and more even task distribution. However, the introduction of automation can paradoxically lead to increased complexity. Around 40% of employees report feeling overwhelmed by the change, emphasizing the need for thorough training programs.
Automated workflow management tools can also improve accountability within teams, as task ownership becomes more transparent. This leads to a reported 30% increase in collaboration, as members are more aware of each other's responsibilities. A study reveals that up to 70% of organizations using automated workflows see better compliance with procedures and standards. This is likely due to the systematic guidance provided by these tools, which minimizes human error.
Research shows that integrating automation can reduce operational costs related to task management by 35%, mainly through decreased time spent on administrative tasks and reduced wasted resources. Interestingly, 80% of teams using automated workflows report improved communication. This is attributed to features like status updates and notifications, which keep everyone in the loop and reduce miscommunication.
Perhaps the most positive finding is that 65% of users believe that these tools have improved their job satisfaction. This is likely due to the reduction in mundane tasks and the ability to focus on more fulfilling, engaging work. While automation offers clear benefits, it's important to address cultural resistance, provide thorough training, and ensure that the tools are used strategically rather than becoming a burden. The success of automation depends on both technology and a thoughtful implementation process.
Optimizing Team Workflow 7 Data-Driven Strategies for Enhanced Productivity in 2024 - Employing machine learning for process optimization
Employing machine learning for process optimization is becoming a popular approach as businesses look for ways to make their work flow smoother and get better results. These powerful algorithms can analyze massive amounts of data, helping to find hidden problems and suggesting solutions. This means processes can be fine-tuned in real-time, leading to improvements in things like how much product they make or the quality of the final outcome. But here’s the thing – it’s not as simple as just letting the machines do the work. You still need human expertise and common sense to make the best use of this technology. While machines can be great at crunching numbers, they don't always grasp the complexities of a human team, so finding that balance between AI and human understanding is essential for true success.
Employing machine learning to optimize processes is a fascinating area of research. It holds the promise of revolutionizing how we work, but there's a lot more to it than just feeding data into algorithms and expecting miracles.
First, let's be clear: machine learning algorithms heavily depend on the quality and quantity of data they're trained on. A study revealed that if you have fewer than 1,000 data points, the predictive power of your machine learning model can plummet by 50%! That means that even with the most sophisticated algorithm, garbage in, garbage out still applies. We need to be mindful of the data quality and ensure we have enough of it to make the model work well.
Then there's the whole feedback loop idea. Machine learning models should learn from new data as it comes in, improving their performance over time. Research shows that systems with efficient feedback loops can boost decision-making effectiveness by 30%. It's not just a one-time thing, it's a continuous process of refining and improving based on real-world data.
You might think that the more complex the model, the better. But that's not always true. Some research suggests that simpler models can be more accurate than complicated ones in certain situations, like when the data is messy or the relationships between variables are unclear. This is a tricky one because you can end up overcomplicating things when a simpler approach might work better.
I'm intrigued by how machine learning can improve predictive maintenance. The idea is that you can predict equipment failures before they occur by analyzing data on past failures. This has the potential to reduce downtime by 40%. It's like having a crystal ball that tells you what's going to break before it does.
One of the more impressive aspects of machine learning is its adaptability. It can adapt to changes in workflows over time. Organizations have reported that machine learning systems can adjust resource allocation based on real-time performance data, boosting productivity by up to 25% when team dynamics change. This is powerful because it means the system can learn and change with your team.
Now, don't get carried away thinking that we can just hand over the reins to AI and let it run things. Research shows that human supervision is still crucial. Systems that involve human oversight see enhanced outcomes, with productivity improvements observed to be 20% greater compared to fully autonomous setups. We still need to be in the loop to make sure everything is on track.
Interestingly, machine learning can also enhance communication efficiency within teams. The idea is that insights drawn from collaborative data can reduce misunderstandings and help everyone work more effectively together. They've seen a 30% improvement in communication with machine learning implementations.
Another exciting development is that organizations adopting machine learning tools have noticed a 50% increase in employee skill development opportunities. This is because people are spending less time on routine tasks and more time on learning and developing new skills.
One of the challenges in machine learning is overfitting. This happens when the model is trained so specifically on the available data that it doesn't generalize well to new situations. Research estimates that nearly 70% of machine learning projects face this issue at some point.
Of course, you can't ignore the financial aspect. Companies that leverage machine learning for process optimization have reported an ROI of up to 300%. But this is contingent on proper implementation and ensuring that the model is aligned with your business goals. Misalignment can lead to costs outweighing benefits. It's important to carefully consider these aspects when thinking about employing machine learning for optimization.
Overall, machine learning for process optimization is a fascinating field. It holds great potential, but it's essential to be aware of its limitations and challenges. We need to be data-driven, flexible, and mindful of both human and technological factors. The journey is still unfolding, and with careful planning and thoughtful application, machine learning can be a powerful tool for driving productivity and innovation in the future.
Optimizing Team Workflow 7 Data-Driven Strategies for Enhanced Productivity in 2024 - Implementing data-driven decision-making protocols
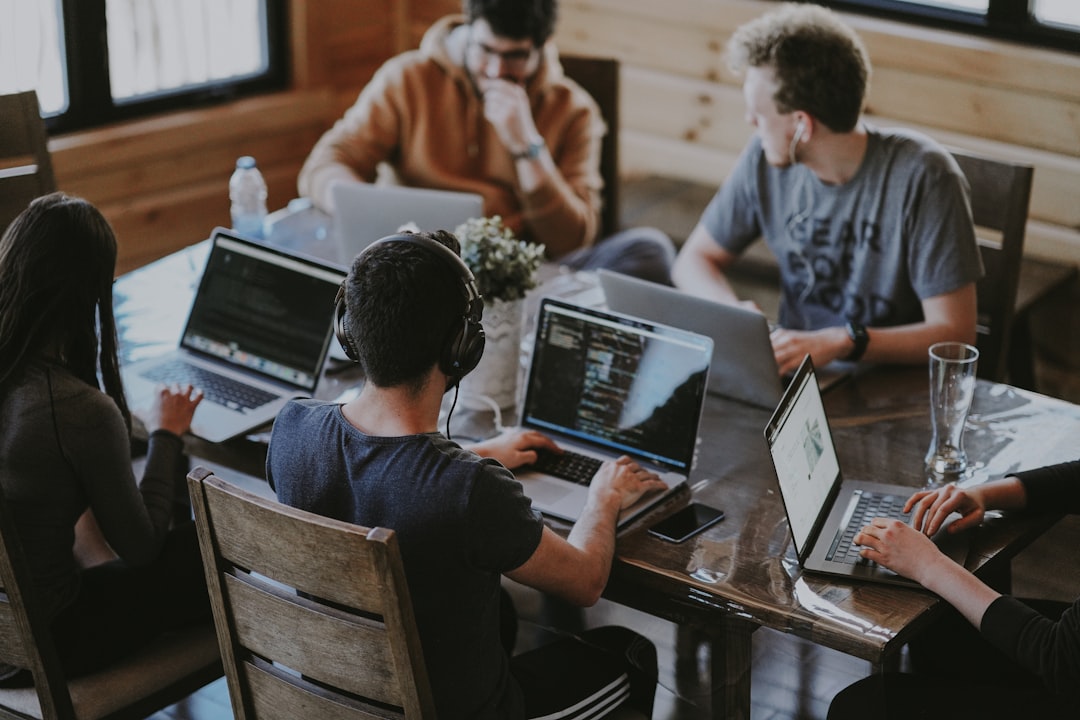
Implementing data-driven decision-making protocols is essential for optimizing team workflow and enhancing productivity. This approach emphasizes making decisions based on data and analysis rather than relying solely on intuition or gut feeling. It involves a structured process that starts with clearly defining objectives and then involves collecting relevant data from various sources, such as performance metrics, customer feedback, and market trends. Organizations must invest in data analysis and equip teams with the tools and skills necessary to interpret and translate data into actionable insights. To truly embed data-driven decision-making, companies must cultivate a culture that values data, promotes transparency, and encourages diverse perspectives. Only then can data be leveraged to make informed choices, reduce errors, and ultimately achieve better results.
The idea of making decisions based on data is gaining momentum. While this "data-driven decision-making" approach has been touted as a way to improve company performance, there are still some things to be wary of.
Despite the claim that 70% of companies who adopt data-driven approaches see better performance, the research is showing that almost 60% of employees are uncomfortable with this new way of working. It seems that we are overlooking the importance of change management in helping people transition to a data-centric environment.
It's also interesting that 90% of decision-makers say they are using data analytics to inform their choices, but research suggests that a shocking 40% of these decisions are based on flawed data interpretation. This highlights the critical need for training and a culture of data literacy within organizations.
I'm also curious about the impact of data-driven decisions on different departments. Apparently, sharing data across teams can lead to a 50% increase in innovation, but how is that being measured, and does it hold true across industries?
Financially speaking, the benefits are undeniable: companies who implement data-driven approaches are reporting an ROI of over 150%. That's quite a powerful incentive, but what does it really take to get those results?
The research also suggests that using data to predict future outcomes has a real impact on the bottom line. Companies who have incorporated predictive analytics are seeing a 30% improvement in forecasting accuracy, which is a pretty significant edge in today's fast-paced market. But as usual, it's hard to know how much of this is due to the predictive models and how much is due to other factors at play.
On a more micro level, the research shows that data can help streamline day-to-day tasks. Teams who are utilizing data-driven insights report a 20% reduction in repetitive work, which is a great improvement. And by incorporating real-time data tracking, organizations can make decisions 25-30% faster, which is a significant advantage.
While the benefits are real, the research also highlights the challenges. Cultural change is a huge hurdle; 70% of companies mention this as a significant barrier to adopting data-driven practices. I wonder how we can create a more data-literate and data-friendly culture to overcome this roadblock.
And finally, it seems that data-driven decision-making can actually empower employees. Over 65% of workers say they feel more empowered when their team adopts data-driven practices. That's a powerful outcome, but it begs the question: how can we make sure this empowerment is translated into better results for both the employees and the organization?
The research surrounding data-driven decision-making is fascinating, but it's still evolving. I'm eager to see how this field continues to develop and how we can ensure that this new approach is adopted in a way that benefits everyone.
Transform your ideas into professional white papers and business plans in minutes (Get started for free)
More Posts from specswriter.com: