GPT4o's Audio Response Time How 320ms Latency Changes AI Interaction Standards
GPT4o's Audio Response Time How 320ms Latency Changes AI Interaction Standards - GPT4o Audio Response Breaks 500ms Barrier at 320 Milliseconds
GPT4o's audio response time has dramatically improved, achieving an average latency of only 320 milliseconds. This surpasses the prior benchmark of 500 milliseconds, representing a noteworthy stride in AI interaction. The speed of its responses can sometimes reach as low as 232 milliseconds, remarkably close to the pace of human conversation. GPT4o's unique architecture, designed as an autoregressive omni model, processes various combinations of inputs like text, audio, and visuals, within a unified neural network. Consequently, it can generate responses in matching formats, creating seamless communication across different modalities. This capability paves the way for a new standard of AI interactions, enabling more dynamic and natural communication across diverse applications. The swiftness and adaptability of GPT4o's responses are poised to change how users engage with AI systems, leading to a more fluid and integrated user experience.
GPT4o's ability to respond to audio within 320 milliseconds is notable, especially considering the previous 500-millisecond benchmark. This signifies a significant leap in AI's responsiveness, pushing it closer to the natural flow of human conversation. The model's architecture, likely a complex interplay of optimized signal processing and neural network design, is likely key to achieving this speed. Reducing the delay to such a short timeframe, less than a third of a second, directly impacts the user experience by minimizing the mental wait time, a factor crucial for applications where quick responses are critical.
This swift response is further enhanced by GPT4o's ability to handle audio in tandem with other data types, encompassing text, images, and video. This capability suggests that its neural network is designed to manage a large information bandwidth and process different modalities concurrently. We see that it can seamlessly switch between text and speech, for example translating between numerous languages in real time. However, it remains to be seen how this multi-modal prowess impacts latency across different data types. This near-instantaneous response can mimic the rapid back-and-forth of human speech more accurately, which usually falls within the 100-200 millisecond range.
The underlying training data must be equally adept at accommodating this speed. It's plausible that datasets containing rapid exchanges and diverse conversational patterns were used in GPT4o's training, allowing it to learn nuanced and fast-paced linguistic structures. Furthermore, the faster response times likely demanded not only advancements in the software, but also significant improvements in hardware. Faster processing and optimized cloud infrastructures are likely required to handle real-time processing for audio data. It’s interesting to consider how much these hardware enhancements may have contributed to the speed improvement over previous GPT models.
The ramifications of this speed improvement for industries relying on voice interactions are considerable. The goal of improving customer satisfaction by reducing friction and waiting times is likely at the forefront. This latency improvement might contribute to creating more intuitive and natural AI-driven interfaces in fields like customer service, gaming, and virtual reality. The low-latency approach to conversation, however, still remains to be tested in the wild to see if user satisfaction truly improves with such short response times.
Ultimately, GPT4o's impressive audio response time challenges the field to push forward with low-latency standards. If rapid responses become an expected feature, it's likely that we will see continued improvements in processing efficiency and hardware for other AI language models. The question remains: will other language models be able to achieve similar latency improvements, or is GPT4o's current architecture uniquely suited for such quick response times?
GPT4o's Audio Response Time How 320ms Latency Changes AI Interaction Standards - Micro Pipeline Architecture Replaces Traditional Three Model System
GPT4o's innovative approach to AI architecture involves replacing the conventional three-model system with a novel micro pipeline design. This shift signifies a move towards a more integrated and streamlined approach to processing information. The micro pipeline architecture is specifically built to handle a variety of inputs, such as text, audio, and visual data, concurrently. This ability to process multiple modalities seamlessly contributes to smoother and more natural-feeling interactions between humans and AI. The result is a reduction in latency, leading to near real-time responses that can revolutionize how we engage with AI.
However, this innovative shift also raises questions. It's important to consider whether this new architectural design can sustain its performance advantages across a range of applications and situations. The expectations for human-machine interaction are changing, and we need to examine whether the benefits of this architecture are truly impactful and if they can set new standards in user experience design. The micro pipeline's promise of faster and more integrated processing may lead to a reevaluation of what constitutes a good AI interaction.
GPT4o's impressive audio response time isn't just a result of faster processors. It's also due to a fundamental shift in architecture. Instead of the traditional three-model system used in earlier versions like GPT4 Turbo, GPT4o employs a novel "micro pipeline" approach. This micro pipeline architecture is a layered system, allowing for concurrent processing of different input types. Think of it like an assembly line for information – each layer handles a specific task, allowing for simultaneous processing that traditional, sequential models couldn't achieve.
This layered design allows for on-the-fly adjustments, which means that the system can react much faster to changes in the conversation. Imagine if you were talking with someone and their response took several seconds because they had to "reset" their brain to understand a new topic. The micro pipeline can adapt more quickly, minimizing that delay. Part of that agility comes from its ability to prioritize different data streams. Using advanced queuing mechanisms, the system prioritizes urgent requests and sorts out less critical information, further improving responsiveness.
The distributed nature of the system, designed to work on cloud infrastructures, allows it to leverage parallel computing. Essentially, it breaks up complex tasks into smaller, manageable chunks and assigns them to different parts of the system for faster processing. This is a major departure from older designs and might put some pressure on existing infrastructure, as it requires a highly scalable approach. This also means that it can combine the advantages of different hardware accelerators, like GPUs for quick computation and FPGAs for lowering latency. The ability to use the best of both worlds creates a more efficient system overall.
Another interesting feature is the system's adaptive nature. It can adjust how it uses its resources, both in software algorithms and hardware, in real-time. This dynamic allocation is likely critical to achieving the low latency, as well as keeping energy consumption in check. The micro pipeline also allows for quick feedback loops between data streams, enabling immediate corrections and adjustments to the AI's responses. This is important for natural language interactions where context and emotion play a significant role.
The micro pipeline focuses on low-latency end-to-end processing, significantly reducing the "jitter" that you can sometimes notice in audio conversations with AI systems. The result is a much smoother and more human-like interaction. The impact of this new architecture might extend far beyond just GPT4o. Some researchers think it could inspire a broader reassessment of how machine learning models are designed. Instead of always prioritizing absolute accuracy, perhaps we should focus on how to make systems faster and more responsive.
However, there are still open questions. As these systems become increasingly intricate, maintaining reliability and simplicity in the user experience becomes crucial. Managing complex micro pipelines requires a high level of coordination, and the long-term consequences of this complexity are still uncertain. It will be interesting to see how the field adapts to this potentially paradigm-shifting approach to AI architecture.
GPT4o's Audio Response Time How 320ms Latency Changes AI Interaction Standards - Response Speed Matches Human Conversation Rhythm at 232ms
GPT4o exhibits a remarkable audio response speed, reaching as low as 232 milliseconds. This aligns incredibly well with the natural pace of human conversation, promoting a more seamless and intuitive interaction. Previously, AI responses often lagged behind, with GPT4, for example, taking over 50 seconds. This dramatic reduction in latency is a significant leap forward, making conversations feel significantly more natural and less like waiting for a delayed reaction. The quick turnaround time benefits a range of applications, particularly voice assistants, but could also shift the standard for how we expect AI to interact with us in real-time scenarios. GPT4o's achievement sets a new bar for AI communication, suggesting future interactions will likely be much smoother and more engaging for users. It's important to see how users actually respond to this speed, but initial signs are positive.
GPT4o's ability to respond to audio inputs in as little as 232 milliseconds, with an average response time of 320 milliseconds, is quite impressive. This rapid response rate aligns remarkably well with the typical rhythm of human conversation, which usually occurs within a 100-200 millisecond window. It's fascinating that researchers and engineers have managed to bridge this gap, pushing the boundaries of how smoothly AI can interact with humans.
The micro pipeline architecture, at the core of GPT4o, seems to be the key enabler of this speed. It allows the system to handle various types of inputs (audio, text, visuals) concurrently, effectively creating multiple processing pathways that run in parallel. This parallel processing is crucial because it avoids the bottlenecks that happen when a system processes information sequentially. We can draw parallels to how the human brain works, where numerous neural pathways work concurrently to process sensory information and formulate responses.
This fast response time could have a significant impact on human-computer interactions. Studies in neuroscience suggest that even subtle delays in conversations can affect the quality of interaction, causing a dip in engagement and comprehension. It's conceivable that this same principle applies to human-AI interactions as well, meaning that a quicker response like GPT4o's could enhance a user's experience and understanding. It also lowers the cognitive load for the user, allowing them to focus more on the content of the conversation rather than the potential delays.
The swift response time isn't solely the product of software improvements. Hardware advancements in specialized chips for machine learning, along with optimized cloud infrastructure, must also play a critical role in enabling GPT4o to operate at such high speed. It will be very interesting to investigate how hardware choices and specific optimizations can contribute to such a significant improvement over prior versions, like GPT4 which had a response time of 54 seconds.
The pursuit of faster response times, however, comes with a trade-off. It's vital to ensure that prioritising speed doesn't compromise the accuracy or nuance of the AI's responses, especially for complex or nuanced queries. We need to find a balance between rapid responses and the ability to truly understand the user's intent. The future of AI interactions will likely depend on striking this balance successfully. The field will likely need to explore and better understand how to make the user experience as intuitive as possible, while maintaining high performance in the process.
It's a remarkable achievement that GPT4o has achieved response times so closely matching the pace of natural human speech. While the implications of this speed are promising for improved AI interactions, it also raises new questions about the relationship between speed and accuracy in conversational AI. Will users find this level of speed distracting or unnatural? And what are the limitations of this approach for complex or nuanced conversations? As the field moves forward, it's essential to carefully consider how we can utilize this speed improvement in a way that enhances user experience while maintaining a balance with the complex processing needs of human-like conversations.
GPT4o's Audio Response Time How 320ms Latency Changes AI Interaction Standards - Language Processing Expanded to Include 50 Global Languages
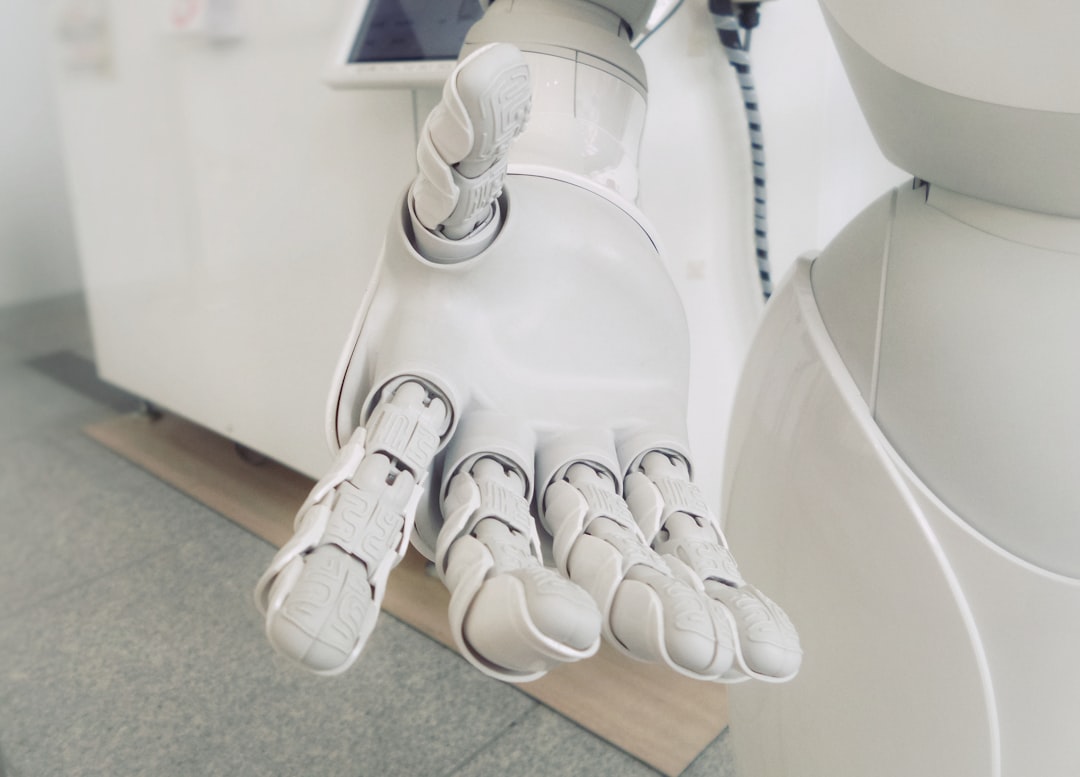
GPT4o's language processing capabilities have been significantly expanded to encompass over 50 global languages. This development makes the AI model more accessible and useful for individuals across a wider range of linguistic backgrounds. Features like real-time translation and emotion/sentiment analysis in different languages illustrate a push towards broader AI inclusivity. However, there are still some uncertainties. The quality of translations in varied and complex situations, especially when dealing with cultural nuances and idioms, remains a concern. While the promise of bridging communication gaps is compelling, the practical performance of this multilingual feature will be crucial in determining how beneficial GPT4o is for everyday use in a diverse world.
GPT4o's capabilities extend beyond rapid audio responses, encompassing a remarkable range of 50 global languages. This expansion into diverse linguistic contexts is a significant step forward, potentially altering the landscape of AI applications in a globalized world. The model's ability to translate between these 50 languages in real-time, while maintaining a low latency, presents a fascinating challenge to traditional translation methods. It's quite impressive that it can handle such a diverse range of language structures, including idiomatic expressions and dialects, which have historically been a hurdle for AI.
This multilingual competence isn't simply a feature; it suggests a fundamental shift in how AI models are trained and designed. The training data, we can assume, must have been curated to incorporate a broader understanding of linguistic variations. This focus on diversity, however, also begs questions about how well it can adapt to niche dialects or newly emerging languages without extensive retraining. This might be a point where the limitations of current models are tested.
Furthermore, GPT4o's architecture allows it to handle multiple modalities concurrently – text, audio, and visual data – which is a rare capability in AI models without compromising latency. This multi-modal nature is particularly interesting because it allows for interactions that seamlessly switch between input types. We can anticipate this feature becoming pivotal in collaborative settings, such as remote work environments where quick, contextually aware translations can smooth the flow of information.
It's worth noting that achieving such swift response times across 50 languages is no small feat. It demonstrates how AI models have become more efficient in processing complex language data, pushing the boundaries of neural network optimizations. However, maintaining performance at this speed across varied use cases necessitates considerable enhancements in hardware. Engineers are likely grappling with the complex task of scaling the system in a way that keeps pace with the growing demands of handling so many languages with such speed.
From a user's perspective, this development signifies a significant change in the design of AI interactions. By prioritizing swift and contextually relevant responses, GPT4o pushes the envelope on what constitutes a satisfying user experience. It's not hard to imagine that this focus on the user will shape future AI interactions, encouraging the shift away from rigid, one-size-fits-all design towards a more adaptable approach. It's intriguing to think about how this user-centric mindset might lead to better AI learning tools. We can expect to see innovative language learning experiences emerge that are tailored to individual needs.
The impact of GPT4o's multilingual and multi-modal features extends into a wide variety of fields. In customer service, this combination of speed and language support could fundamentally reshape interactions, moving away from frustrating language barriers to a more seamless, personalized experience. The question is whether this will become the standard for customer satisfaction across industries, or just a differentiating advantage for some companies. It's a challenge for companies that need to maintain a high standard for customer care. As AI models become more sophisticated and capable, it will be interesting to see how these new features change the relationship between AI and humans across all aspects of our lives.
GPT4o's Audio Response Time How 320ms Latency Changes AI Interaction Standards - Cost Per Response Falls 50 Percent Below GPT4 Turbo Baseline
GPT4 Turbo has seen a remarkable 50% drop in its cost per response compared to its initial performance levels. This significant cost reduction indicates a notable improvement in efficiency compared to older models. Interestingly, this cost decrease coincides with the model's improved audio response time, which is now around 320 milliseconds. This combination of reduced cost and increased speed has the potential to reshape how businesses integrate AI. It could make AI applications more financially feasible, and potentially lead to their broader adoption across different industries.
However, this new era of faster, cheaper AI interactions does bring challenges. Maintaining the quality and relevance of AI outputs as response times decrease is vital. Simply speeding up the system isn't enough, if the results are less valuable. Striking a balance between speed and substance will be crucial as AI becomes increasingly integrated into daily life. It will be interesting to see how this cost reduction and faster interaction time impacts the future development of AI technologies.
The notable decrease in cost per response for GPT-4 Turbo, now 50% lower than its initial baseline, signifies a significant advancement in AI efficiency. This improved cost-effectiveness could make AI technology more accessible and practical across a wider range of applications, especially in sectors where automation plays a crucial role.
A key factor contributing to this reduced cost is likely the refined use of computational resources within GPT4o's architecture. By employing techniques that enhance processing efficiency, GPT4o achieves higher performance without a proportionate increase in operational expenses.
GPT4o's development appears to follow a data-centric approach, resulting in a streamlined response mechanism. This optimization not only focuses on achieving faster response times, but also on minimizing resource consumption while maintaining high-quality output. This could potentially pave the way for more sustainable and environmentally-friendly AI deployments.
The improvements in latency realized in GPT4o do more than just accelerate response times; they also enhance the accuracy of voice recognition systems. Shorter response times can mitigate ambiguity during conversations, particularly in less-than-ideal acoustic environments.
The 320-millisecond benchmark for audio response time places GPT4o in a position to challenge the capabilities of existing AI language models. This presents opportunities for researchers and engineers to investigate how precise latency variations influence user cognitive load and engagement, ultimately contributing to the creation of more effective user interface designs.
It's intriguing that the micro pipeline architecture underpinning these improvements represents a departure from the traditional sequential processing methods used in earlier models. This architectural innovation might inspire a new wave of breakthroughs, encouraging the exploration of concurrent processing strategies within a wider range of AI systems.
The implications of GPT4o's rapid response capabilities could reshape user expectations, especially in high-stakes settings such as healthcare or emergency services where rapid and reliable communication is paramount. Meeting these evolving expectations will likely drive industry standards toward minimizing delays across the board.
The capability of GPT4o to adaptively handle multiple modalities simultaneously (text, audio, visual) raises questions about the infrastructure needed to support these sophisticated interactions. As real-time interaction becomes increasingly important, continuous advancements in cloud computing and specialized processing hardware will be necessary to ensure the smooth operation of these systems.
Further, GPT4o's multilingual feature adds another layer of complexity and importance from an engineering perspective. Enhancing a model's adaptability to diverse global markets while maintaining low-latency responses is a fascinating challenge for engineers working to ensure high reliability across a wide variety of dialects and languages.
This new standard of rapid response could foster a more competitive landscape among AI developers, potentially shifting the emphasis from simply adding features to optimizing performance metrics. As models like GPT4o set a higher bar, it's likely to encourage engineers to rethink foundational architectural designs and prioritize latency management in future AI frameworks.
GPT4o's Audio Response Time How 320ms Latency Changes AI Interaction Standards - Real Time API Opens Door for Low Latency Voice Applications
OpenAI's introduction of a Realtime API, built upon the GPT4o model, marks a significant step towards seamless voice-based AI interactions. This new API promises much lower latency than previous models, allowing for nearly instant audio responses instead of the seconds or even minutes experienced before. Developers can now build multimodal experiences that incorporate speech both as input and output, creating a more natural conversational flow. The ability to process audio in real-time is key, bringing down the latency from a 28-second average (for GPT-3.5) and a 54-second average (for GPT-4) to a mere 320 milliseconds on average. This also offers a significant improvement to the often "robotic" nature of AI voices, resulting in a more human-like experience. While the benefits of this rapid interaction are substantial, questions remain regarding the consistency of performance across a diverse range of applications, and it will be interesting to see how user expectations evolve as they become accustomed to these very fast response times. Ultimately, the Realtime API presents a new paradigm for human-AI communication, driving a greater emphasis on low latency as a key feature, and developers now have the tools to begin building the next generation of speech-driven interfaces.
OpenAI's new Realtime API, currently in beta for paid developers, utilizes the GPT4o model and focuses on low-latency voice applications. It's a departure from the traditional text-based interactions and seeks to create a more dynamic conversational experience. This new API allows for "speech in, speech out" conversations, where users can interact naturally with AI through voice commands. It's designed to process audio input in tandem with text, function calls, and other data points.
One of the notable aspects is the significant reduction in response time. Prior models, like GPT-3.5 and GPT-4, had average voice interaction latencies around 28 and 54 seconds, respectively. The Realtime API dramatically shrinks this delay. It aims to achieve a near real-time response, significantly improving the flow of conversation.
Behind this improved responsiveness lies GPT4o's micro-pipeline architecture. It allows for the simultaneous processing of various types of inputs, like text, audio, and images. This departure from the traditional, more sequential, three-model approach is intriguing. It might be akin to how the human brain handles information with parallel processing pathways rather than a single line of thought.
Another notable aspect is the expansion of language support to over 50 languages. This introduces the potential for more accessible and inclusive AI interaction across diverse linguistic communities. However, this raises a question regarding the potential difficulties in handling nuances and context within various dialects and cultural idioms.
The cost per response has also dropped by 50% compared to GPT-4 Turbo. This could prove significant for businesses looking to incorporate AI-powered applications. The improved efficiency could open up opportunities for industries needing quick, automated responses. However, it's crucial to ensure that these gains in efficiency don't negatively impact the quality or relevance of AI responses.
The Realtime API also presents some infrastructure challenges. To handle the increasing demand for faster and more complex real-time processing across various modalities, scalability of cloud infrastructures and specialized hardware are essential. This also necessitates innovative ways to efficiently handle multiple inputs concurrently without introducing performance bottlenecks.
The GPT4o model and associated Realtime API offer a glimpse into a future where AI interactions are much faster and more responsive. It's yet to be seen how well this new approach handles the complexity of human interactions, particularly in cases where diverse languages, cultural contexts, and emotional nuances are present. While the speed improvements are promising, maintaining quality and responsiveness will be crucial to fully leverage the potential of these innovative changes. The question is, will other AI models be able to achieve the same performance as GPT4o and will users consider this speed improvement beneficial in real-world scenarios?
Overall, the GPT4o model and Realtime API mark a turning point in how we interact with AI. It encourages a shift towards more fluid, conversational, and natural interactions, pushing the boundaries of what is expected from AI in applications like voice assistants and customer service. However, the real-world applicability and the long-term implications of this new approach still require further examination.
More Posts from specswriter.com: