Transform your ideas into professional white papers and business plans in minutes (Get started for free)
AI Attractiveness Ratings Analyzing the Accuracy and Limitations of Beauty Algorithms in 2024
AI Attractiveness Ratings Analyzing the Accuracy and Limitations of Beauty Algorithms in 2024 - AI Beauty Algorithms Accuracy Rate in 2024
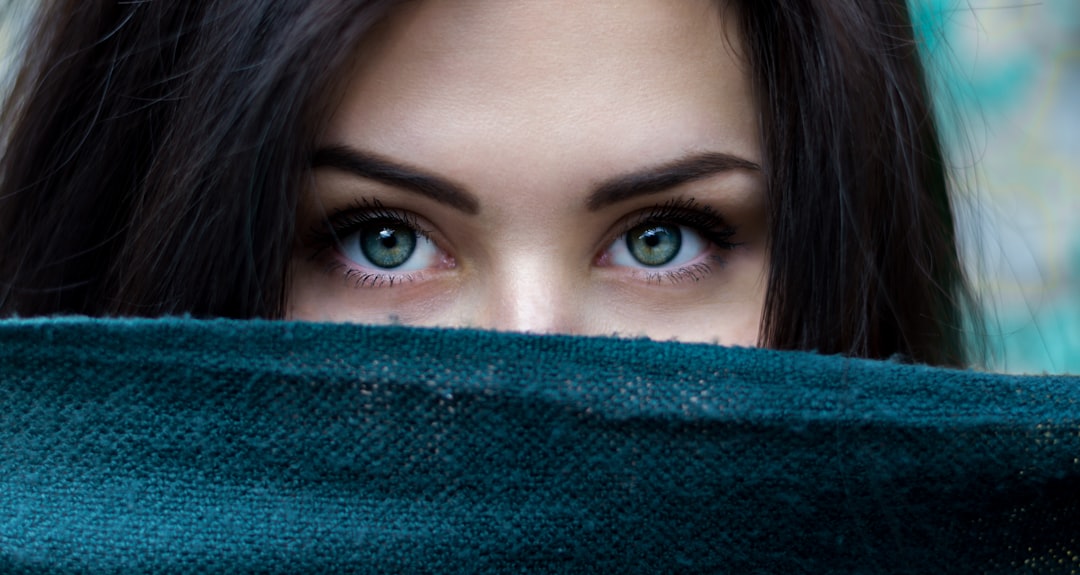
The accuracy of AI algorithms in determining beauty remains a topic of discussion in 2024. These tools, such as those found in Face Its AI and Face Rating AI, employ intricate algorithms to assess facial features like symmetry and characteristics tied to evolutionary psychology, generating attractiveness scores. A notable finding is the frequent disparity in scores between genders, indicating potential biases embedded within the algorithms themselves. While individuals might utilize these scores for self-improvement, the inconsistencies in results highlight concerns about their reliability and whether they truly reflect a diverse range of beauty standards. The burgeoning AI beauty market underscores the urgent need for a thorough analysis of these technologies, considering their possible impact on society.
Currently, AI beauty algorithms are showing a reported accuracy rate of approximately 75% in 2024. This signifies substantial leaps in image analysis and processing. However, it also emphasizes their ongoing reliance on established, and potentially subjective, standards of beauty. These algorithms primarily focus on quantifiable aspects like facial symmetry, skin condition, and other measurable traits. However, they often overlook the diverse range of cultural and personal factors that influence how individuals perceive beauty. Interestingly, beauty ratings from these algorithms can differ considerably depending on the group evaluating the images, suggesting that some algorithms may prioritize features popular within a specific training dataset.
Transparency remains a challenge in 2024, as many beauty algorithms lack clarity in how they assign weight to different facial characteristics. This raises valid ethical concerns about potential biases in the generated ratings. Additionally, some of these algorithms appear to struggle when encountering unconventional beauty features. While they seem to effectively assess common facial traits associated with traditional beauty standards, they often stumble when presented with unique or non-standard aesthetics.
Furthermore, the influence of external factors such as lighting and background can significantly alter the outcomes of these algorithms. Studies have revealed noticeable differences in ratings under varying conditions, questioning the perceived robustness of these assessments. There's a concerning tendency for these algorithms to reinforce current beauty standards instead of challenging them, which could inadvertently perpetuate narrow perspectives and potentially marginalize diverse appearances.
While the implementation of advanced machine learning techniques has significantly enhanced the capabilities of these algorithms, they still regularly produce inconsistent results compared to human evaluations. This highlights their existing limitations. Moreover, a curious phenomenon has emerged where individuals often adjust their photos to conform to AI-generated beauty standards. This can contribute to a cycle of unrealistic expectations surrounding personal appearance.
Researchers are actively working on reducing bias in these systems by introducing diversity in the training datasets. However, a widely accepted definition of an "inclusive" beauty standard remains elusive. As a result, these AI algorithms are continuously evolving and undergoing refinement.
AI Attractiveness Ratings Analyzing the Accuracy and Limitations of Beauty Algorithms in 2024 - Facial Features Analyzed by AI Attractiveness Tools
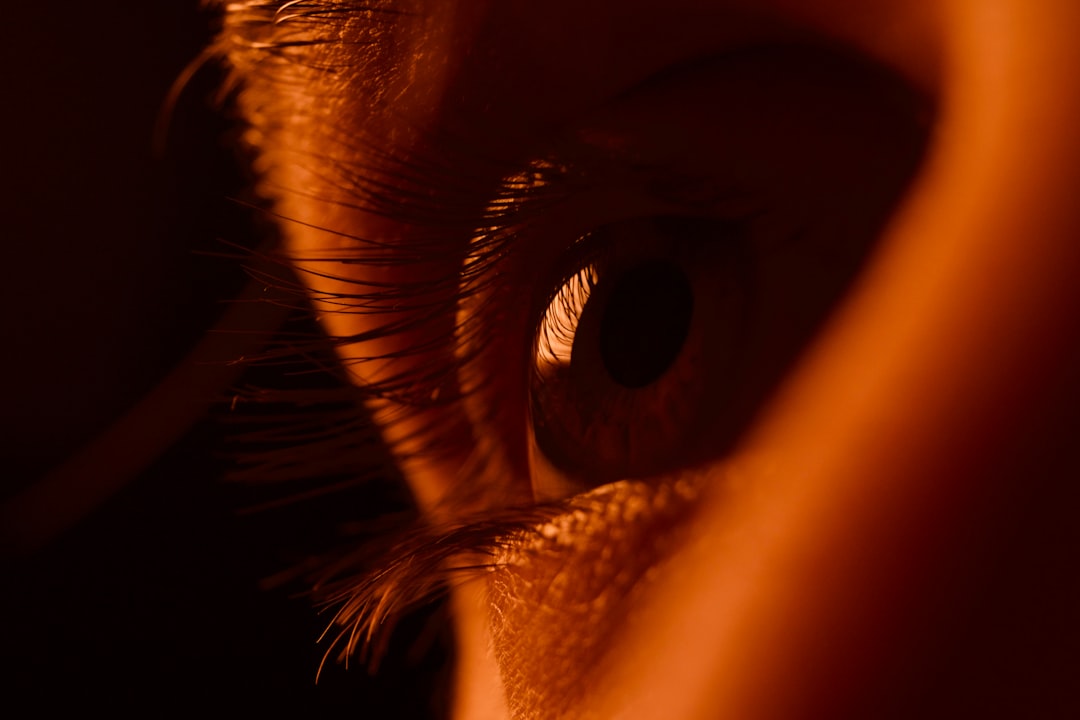
In 2024, AI attractiveness tools are scrutinizing facial features in an attempt to objectively measure beauty. These tools analyze aspects like symmetry, proportions, and the spatial arrangement of features like eyes, nose, and lips, assigning numerical scores that represent perceived attractiveness. While aiming for a quantifiable approach to beauty, these systems often produce varying attractiveness ratings for the same face across different AI platforms, questioning their consistency. This raises concerns about whether these scores accurately reflect a wide range of beauty standards, particularly across cultures and individual preferences. While the algorithms try to identify traits linked to conventionally attractive features, they often fall short when it comes to recognizing a diversity of beauty ideals. This can lead to the reinforcement of existing beauty norms and potential biases in the scoring process. The quest to develop AI attractiveness tools that are genuinely inclusive and encompass a broad understanding of beauty remains a developing and complex task.
AI attractiveness tools, in their quest to quantify beauty, often analyze facial features like symmetry, proportions, and the spatial relationships of elements like eyes, nose, and lips. These assessments are translated into scores, typically ranging from 1 to 10, offering a seemingly objective measure of attractiveness. While designed to be engaging and potentially humorous, the reliance on these scores can be problematic.
Different AI systems can produce significantly different attractiveness ratings for the same photograph. This variation in outcomes highlights inconsistencies in the algorithms themselves. Studies have illustrated this, showing that algorithms can assign scores like 74.8 for men and 77.9 for women for the same individual, like Beyoncé Knowles, revealing possible biases in how the algorithms process certain facial features.
To achieve these scores, AI models require extensive datasets of images paired with human-provided attractiveness ratings. These pairings are used as training data, enabling the models to learn patterns that are statistically linked to perceived beauty. These learning processes involve AI algorithms scrutinizing facial features, leveraging deep learning and geometric morphometrics to determine aspects like symmetry and proportions.
One example of these tools is FACE CHECK, which uses AI to provide beauty ratings. The stated goal of FACE CHECK is to be inclusive, considering a broader definition of beauty. However, studies show that intelligent systems can, to some degree, learn and predict aspects of facial attractiveness based on existing human evaluation norms.
Tools like the AI Face Analyzer adopt a different approach. They prioritize specific facial characteristics like overall clarity and aesthetic quality, eventually resulting in a numerical beauty score. Other applications, like PrettyScale and SeaArt, take a more straightforward approach, categorizing appearances as either "pretty" or "ugly," illustrating the diverse strategies in AI attractiveness evaluations.
Research suggests that the accuracy of these AI beauty algorithms varies considerably. For instance, some algorithms achieve impressive R² values like 0.9902 during specific testing phases. However, these technologies are still under development, and their overall accuracy and consistency need improvement. It's crucial to acknowledge that the underlying algorithms may have limitations in their ability to fully reflect a truly diverse and inclusive definition of beauty.
AI Attractiveness Ratings Analyzing the Accuracy and Limitations of Beauty Algorithms in 2024 - Cultural Biases in AI Beauty Assessment Data
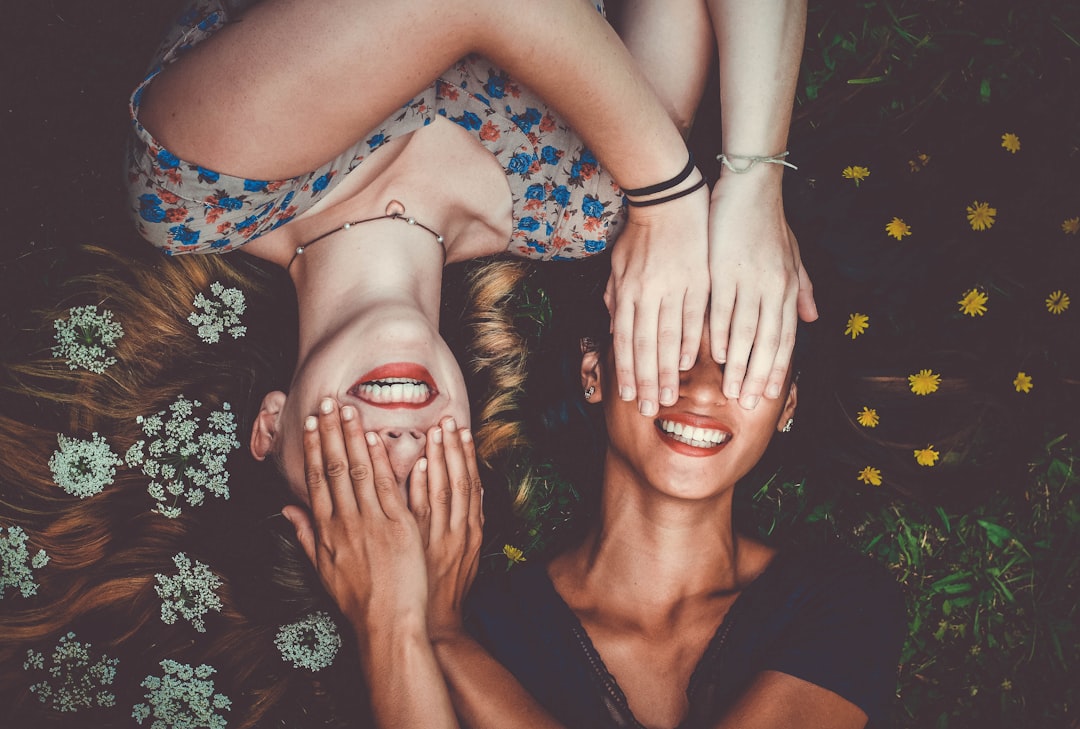
AI systems designed to evaluate attractiveness often struggle with the inherent complexities of beauty, particularly when it comes to cultural biases embedded within the data they learn from. The training datasets used to develop these algorithms frequently lack diversity, leading to an overrepresentation of specific aesthetic norms and an underrepresentation of others. This can inadvertently amplify existing biases in society's perception of beauty, favoring certain features and potentially overlooking the vast spectrum of attractiveness across cultures and ethnicities.
The very notion of beauty is subjective, a concept shaped by cultural and personal preferences that are difficult to quantify. AI algorithms, despite their sophistication, struggle to account for this subjective nature, attempting to impose objective standards on a fundamentally fluid and context-dependent idea. The result is often a narrow interpretation of attractiveness that can inadvertently marginalize individuals whose features fall outside of the limited range captured within the training data.
The ethical implications of this are significant. As these technologies evolve, it becomes increasingly critical to question the fairness and inclusivity of AI-driven beauty evaluations. Moving forward, it's essential that the datasets used to train these systems be more comprehensive and representative of global beauty standards. This requires a nuanced understanding of the cultural and societal factors that influence how beauty is perceived, and a deliberate effort to ensure that AI algorithms are not simply perpetuating existing biases.
AI systems designed to assess beauty often inherit biases from the data they're trained on, which can vary considerably depending on the cultural context of that data. This means that beauty standards that are common in one region or culture might be heavily favored by the AI, potentially leading to skewed attractiveness ratings for individuals from different backgrounds. For instance, certain facial features, like smooth skin or large eyes, might be given more weight in the algorithm's scoring system, which could inadvertently prioritize aesthetics associated with particular ethnic or cultural groups, ultimately perpetuating existing societal biases in beauty ideals.
Furthermore, the datasets used to train these algorithms often lack sufficient representation of minority ethnic groups, potentially causing these groups to be under-represented or misrepresented in the algorithm's output. This creates a limited view of beauty, failing to accurately capture the diversity of attractive features across different populations.
Adding to this complexity, the context surrounding a person's image, such as lighting or background, can significantly impact the AI's rating. The same face might receive wildly different scores depending on these external factors, highlighting a potential weakness in the robustness of the beauty assessments. And, while these algorithms attempt to quantify beauty based on patterns in their data, they often struggle to fully capture the subjective nature of beauty, which is so strongly influenced by personal and cultural tastes. As a result, AI-generated beauty ratings can deviate from what an individual or a community considers attractive.
Complicating matters further, beauty standards evolve across cultures and societies. However, many of these algorithms are based on older or fixed conceptions of beauty, creating a discrepancy between the current societal norms and the beauty that the AI algorithms deem most attractive. The problem is further compounded by the rise of photo editing tools that allow people to adjust their appearances to align with AI-generated beauty ideals. This continuous feedback loop risks reinforcing a very narrow definition of beauty, further marginalizing those who don't conform to these standards.
It's also interesting to note that some AI systems consistently rate men and women differently for similar features, often exhibiting a preference for certain characteristics in women over men. This gender bias, embedded within the algorithm, could potentially shape and influence societal expectations of beauty. And the pervasive influence of social media platforms can amplify this issue. Platforms frequently feature heavily curated images that often align with AI-driven beauty standards, inadvertently reinforcing these standards across digital spaces.
Despite these challenges, there's an opportunity for progress. Resolving the biases in beauty algorithms requires more than just refining existing models. It requires actively seeking out and incorporating more diverse data sets and critically rethinking the very definition of beauty. This approach could lead to a more equitable and inclusive representation of beauty across different cultures and communities.
AI Attractiveness Ratings Analyzing the Accuracy and Limitations of Beauty Algorithms in 2024 - Limitations of Machine Learning in Judging Human Attractiveness
While AI algorithms have shown promise in assessing human attractiveness, their reliance on machine learning presents several limitations. These algorithms often rely on established beauty standards, which can be narrow and fail to encompass the diverse range of features considered attractive across cultures and individuals. Furthermore, the datasets used to train these algorithms can introduce biases, leading to skewed evaluations that may favor specific facial traits over others. This raises concerns about fairness and inclusivity in beauty assessments. The very concept of attractiveness is subjective and influenced by cultural and personal preferences, making it difficult for algorithms to capture the full spectrum of human perception. Although machine learning has significantly advanced the field of AI attractiveness ratings, these technologies still struggle to fully grasp the complexity of beauty. The need to critically examine the outcomes of these algorithms and ensure inclusivity remains a significant challenge for developers and users alike.
While machine learning models have demonstrated a capacity to predict facial attractiveness, aligning with human judgments to a degree, several limitations hinder their ability to offer a comprehensive or unbiased assessment of beauty. Human perception of attractiveness is profoundly subjective, varying greatly across individuals and cultures. Consequently, algorithms trained on datasets that primarily represent specific demographics can inadvertently favor beauty standards associated with those groups, overlooking the diversity of global aesthetics.
Furthermore, the influence of contextual factors like lighting and background on attractiveness ratings underscores the sensitivity of these tools. Frequently, algorithms prioritize traits traditionally associated with attractiveness, like facial symmetry and skin clarity, thereby creating a narrow interpretation of beauty that can marginalize diverse appearances. Interestingly, research indicates potential gender biases within some algorithms, where comparable facial features might receive different attractiveness scores depending on whether they're associated with male or female faces.
The training process of these algorithms also raises ethical concerns, as they learn from existing human-provided beauty ratings that can harbor societal biases. This potential for perpetuation of biases becomes problematic as individuals adjust their appearance in response to these AI-generated standards, creating a feedback loop that reinforces narrow beauty ideals. The absence of standardization further complicates matters, as the same person might receive substantially different attractiveness scores across different AI systems.
Finally, beauty standards evolve over time, yet many of these models rely on datasets that capture older notions of beauty. This discrepancy between AI assessments and modern cultural standards contributes to a challenge in aligning algorithms with contemporary definitions of beauty. These factors highlight that, despite advancements in AI beauty assessment, the creation of truly inclusive and objective beauty algorithms remains a significant hurdle. Continued research into diverse datasets and improved transparency in algorithms' decision-making processes will be crucial in ensuring that these tools reflect a more nuanced and equitable understanding of beauty.
AI Attractiveness Ratings Analyzing the Accuracy and Limitations of Beauty Algorithms in 2024 - Ethical Concerns Surrounding AI-Based Beauty Ratings
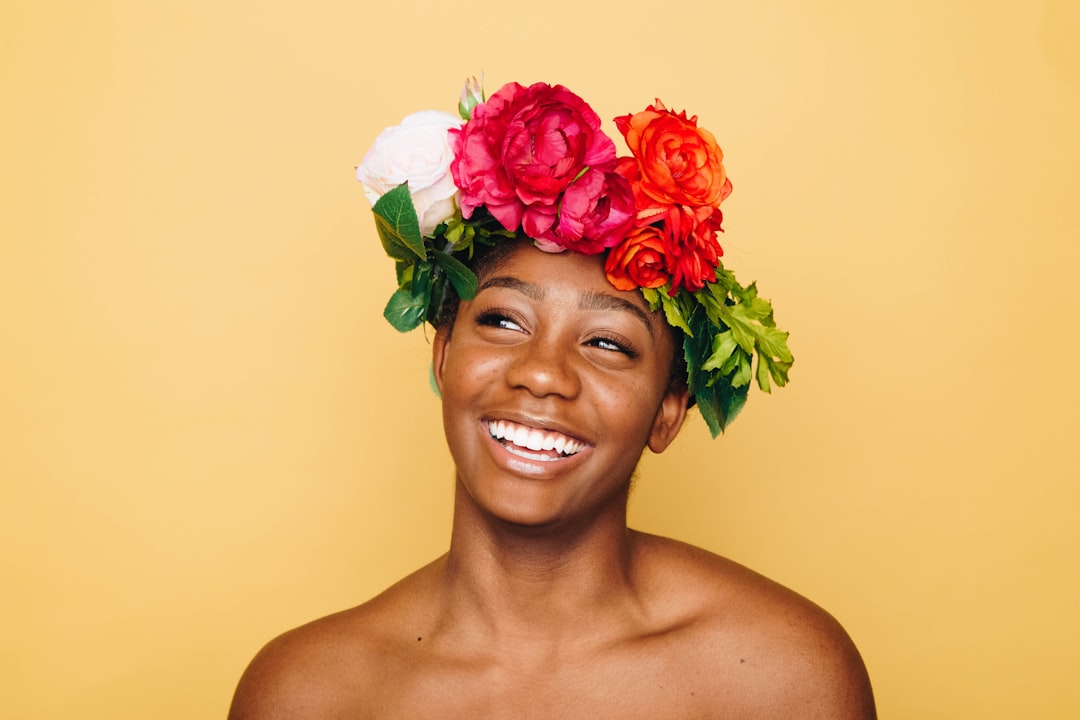
The increasing use of AI to assess beauty introduces a range of ethical concerns that demand attention. One primary worry is that these AI systems often reflect and amplify existing societal biases about attractiveness. This happens because the data used to train these algorithms frequently favors traditional beauty standards, neglecting the diversity of beauty found across cultures and ethnicities. This can lead to distorted ideas of beauty, particularly among younger users who might internalize the AI's skewed judgments as the norm. Furthermore, inconsistencies within these AI ratings can create a cycle where individuals alter their appearances to match AI-generated ideals, inadvertently contributing to the perpetuation of narrow views on attractiveness. Transparency and ethical frameworks governing AI beauty evaluations become critical to ensure a fairer and more inclusive understanding of what beauty encompasses.
The application of AI to assess beauty presents a number of ethical dilemmas, particularly regarding the privacy and management of the data used to train these algorithms. It's become clear that the training data often reflects existing societal biases, which can unfortunately amplify and reinforce pre-conceived notions of attractiveness in the ratings generated. We've seen in studies that images modified to appear more conventionally attractive tend to receive higher ratings across various attributes, including perceptions of intelligence and trustworthiness – a phenomenon often termed the "attractiveness halo effect." This influence of modified images raises interesting questions about how our minds process beauty in the context of AI-altered visuals.
One study involving a large participant pool helped illustrate how cognitive biases can affect our perception of beauty when we interact with AI-based filters and rating systems. A competition launched several years ago, BeautyAI, used automated judgment to assess beauty. It highlighted inconsistencies in its winner selection based on racial diversity; out of a massive number of participants, only a single dark-skinned individual was named a winner. This result raises important questions about the training data and the algorithms used in AI beauty assessments.
Ethical principles for creating and employing AI emphasize the importance of aligning with fundamental human rights and societal values. The ability of AI to predict attractiveness can strongly influence how people perceive others. This is supported by studies that compare how individuals rate attractiveness in images of public figures, where varying characteristics of the image can lead to different ratings. There is, however, a lack of comprehensive research exploring how AI-based beauty ratings impact behavior and self-image in digital environments. It's important to consider the implications of AI-distorted beauty standards on how we view our bodies and our self-esteem, especially for younger audiences.
Current discussions surrounding AI ethics highlight the crucial need for transparency regarding the algorithms used to assess beauty. Transparency is key to minimizing biased outcomes and ensuring a fair representation of diverse beauty standards. There's an opportunity to improve these systems and create a more inclusive view of beauty, but it's imperative that we address these ethical considerations head-on as this field progresses.
AI Attractiveness Ratings Analyzing the Accuracy and Limitations of Beauty Algorithms in 2024 - Future Developments in AI Attractiveness Analysis Technology
The future of AI attractiveness analysis holds both promise and peril. AI algorithms are increasingly sophisticated, employing neural networks to analyze facial features and generate attractiveness predictions with greater precision. While these advancements are notable, the technology continues to be shaped by conventional beauty norms, often failing to capture the diverse range of attractiveness across cultures and individual preferences. Despite improvements, concerns about bias within the datasets used to train these algorithms persist. These biases can lead to a skewed perception of beauty, potentially reinforcing unrealistic ideals and inadvertently marginalizing individuals with unique features.
Moving forward, the ethical considerations surrounding AI beauty assessments will become increasingly crucial. Striking a balance between technological advancement and the need for ethical development will be necessary to ensure that future AI tools for beauty analysis contribute to a more inclusive and equitable understanding of what constitutes beauty. The journey towards developing truly objective and universally accepted measures of beauty using AI is complex and demands a careful consideration of the societal implications that accompany technological innovation in this realm.
The field of AI attractiveness analysis is evolving rapidly, with researchers exploring ways to make beauty assessments more comprehensive and nuanced. We're likely to see a shift towards what are called multimodal approaches, where algorithms consider not just facial features but also aspects like voice tone, body language, and even personality traits. This could offer a far richer understanding of attractiveness beyond just how someone looks.
There's a growing interest in incorporating emotion recognition into these algorithms. Studies have shown that things like happiness can influence how attractive we find someone, and incorporating that into AI assessments could lead to more sophisticated beauty ratings. It's also likely that future beauty algorithms will be much more personalized, adapting their assessments to individual preferences and cultural backgrounds. This could potentially move us away from a single, "one-size-fits-all" standard of beauty.
We can also expect to see AI models that take into account the context in which someone is seen. This means things like the setting, what they're doing, and even the social environment could factor into how an algorithm assesses their attractiveness. This type of contextual awareness would create more realistic beauty assessments aligned with real-world situations.
One of the biggest challenges facing the field right now is algorithmic bias. However, developers are working on solutions. We're likely to see increased focus on algorithmic transparency and accountability, as well as feedback mechanisms to allow for continuous improvement and addressing biases across diverse demographics.
Advances in computing are leading to real-time beauty assessments. It's not hard to imagine future applications of this tech via webcams and smartphones, impacting everything from social media to dating platforms. And as AI continues to advance, we might see a greater emphasis on recognizing and rating non-traditional beauty features, opening the door to celebrating a broader spectrum of attractiveness.
Further down the road, crowdsourced validation may play a role in calibrating AI-generated beauty assessments. Gathering input from a wide range of people across different cultures could help establish a more universal and representative standard. Additionally, some researchers are exploring the links between perceived beauty and health indicators like skin condition. If this line of research progresses, we could see beauty standards shift towards a more holistic approach, incorporating wellness and overall health.
Finally, ongoing research into the societal impact of AI-generated attractiveness norms is vital. It's crucial to understand how AI-driven ideas of beauty influence our culture and the potential impacts on identity and self-esteem. These are complex questions, but it's vital we grapple with them as this field of AI develops further.
Transform your ideas into professional white papers and business plans in minutes (Get started for free)
More Posts from specswriter.com: