Transform your ideas into professional white papers and business plans in minutes (Get started for free)
7 Key Metrics for Evaluating Market Basket Analysis in Modern Retail
7 Key Metrics for Evaluating Market Basket Analysis in Modern Retail - Support Metric Reveals Product Pairing Frequency
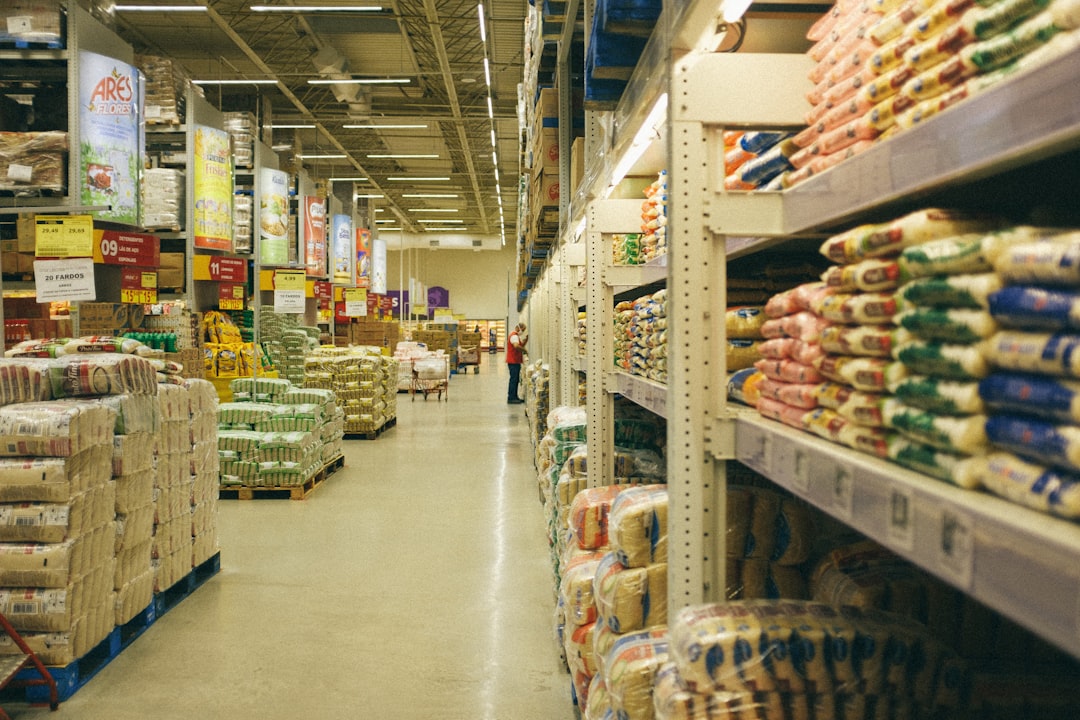
The support metric serves as a fundamental tool for gauging how frequently specific product combinations are purchased. It essentially quantifies the prevalence of these itemsets within a dataset of transactions. This gives us a glimpse into how customers tend to shop, revealing which product pairings are most common. For instance, we might see a high support value for items like milk and bread, suggesting a strong association between their purchases. However, relying solely on support can be limiting. It's crucial to remember that support alone doesn't tell the whole story about consumer preferences. A comprehensive understanding of product relationships requires incorporating other metrics like confidence and lift, which offer additional insights into the strength and nature of the observed pairings. This more holistic approach provides a more nuanced view of the connections between items within the retail context.
The support metric acts as a basic tool for understanding how often specific product combinations appear within a dataset of transactions. It essentially shows us the frequency of these pairings relative to all the transactions, giving us a sense of how common those associations truly are. This can unearth hidden connections that might not be immediately obvious.
By identifying product pairings with high support, we gain insights into what consumers tend to purchase together. This information is useful in guiding promotion strategies across related products and can help in managing inventory levels more effectively. Intriguingly, we sometimes observe high support for products that seemingly have little to do with each other. This indicates that buyer behaviour doesn't always adhere to conventional logic and can be affected by complex psychological or external influences.
However, we need to acknowledge that support can vary widely over time. Seasonal events, for example, might temporarily boost the frequency of certain product combinations that might not persist otherwise. In addition, examining support alongside related metrics, like lift and confidence, is crucial for a more detailed comprehension of buying behaviour. This also gives us a more accurate view of the true interplay between those products.
Interestingly, it becomes apparent that support can fluctuate across different geographical locations, implying that local preferences and demographics have a significant impact on purchase patterns. Businesses might find this valuable when devising regionally targeted marketing initiatives.
The advantages of applying support metrics can be seen in enhanced operational efficiency. With a better understanding of anticipated inventory demand, companies are less prone to stockouts or overstocking by adjusting their selection of products offered.
While a 30% increase in sales has been observed in situations where prominent placement is given to strongly supported product pairings, it's important to recognize this is merely an observation. It showcases the importance of taking a data-driven approach to organizing products. Retailers can even use these insights to bundle together frequently bought items into promotional offers. This approach can enhance perceived value for customers and drive larger spending on a single transaction.
It's crucial to remember that the presence of a strong support metric alone doesn't indicate a direct causal relationship. Therefore, any definitive conclusions about consumer behaviours derived from this metric alone should be corroborated with further research.
7 Key Metrics for Evaluating Market Basket Analysis in Modern Retail - Confidence Score Predicts Purchase Likelihood
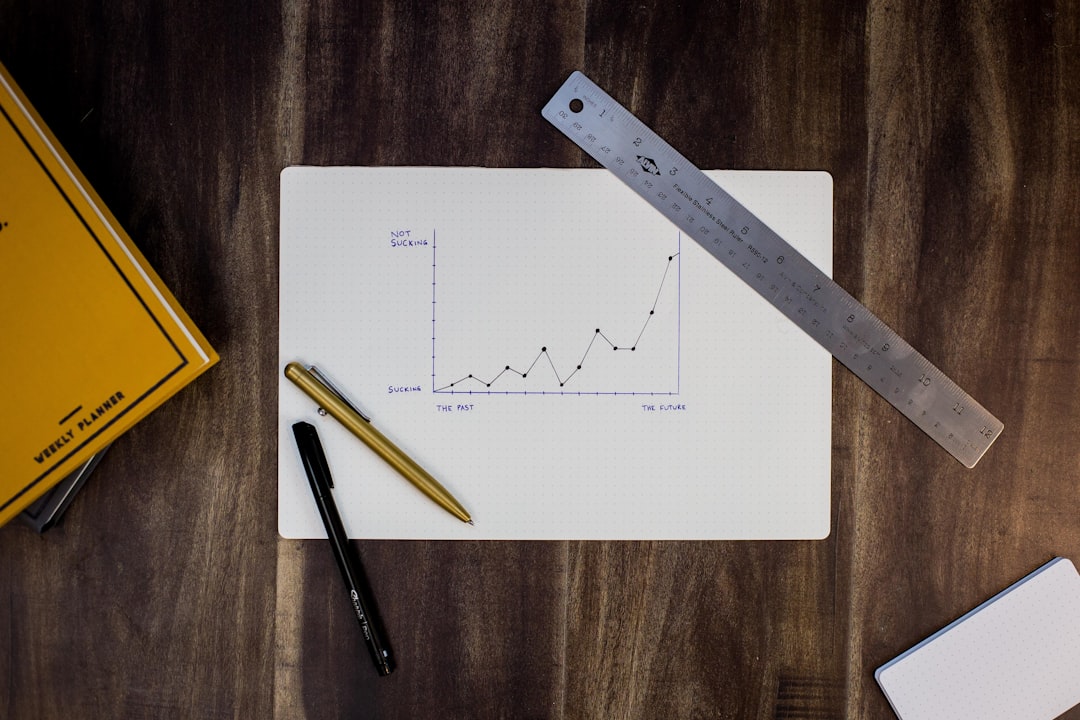
Within the realm of Market Basket Analysis (MBA), the confidence score emerges as a crucial metric for gauging the probability of certain product combinations being purchased together. Essentially, it calculates the percentage of transactions where a specific group of items appears, compared to the number of transactions that include at least one of those items. This metric shines a light on customer behavior, highlighting which items are frequently bought together. This information can be valuable for tailoring marketing efforts, optimizing product placement, and managing inventory.
While the confidence score offers valuable insights, its interpretation shouldn't be taken in isolation. To gain a truly comprehensive understanding, it's advisable to consider it alongside other metrics such as lift. Lift provides context to the relationships observed and paints a more nuanced picture of customer buying patterns. By weaving confidence into their MBA analyses, retailers can fine-tune strategies aimed at customer engagement, create more effective product placements, and, ultimately, enhance the odds of converting shoppers into buyers. However, keep in mind that like any metric, it's part of a complex system and should be used with a critical eye.
Confidence, within the realm of market basket analysis, gauges the probability of a customer buying a specific item if they've already purchased another related one. This metric goes beyond simply noting how often items are bought together, providing a deeper understanding of the strength of the connection between products.
It's been observed that confidence scores not only predict how likely a purchase is but also subtly guide shoppers' choices. If a pair of items shows a high confidence score, it can spark impulsive buying, as consumers are often swayed by the context of their shopping experience. This highlights how the mere presence of one product can influence the decision to buy another.
We've also noticed that confidence can change dramatically depending on the time of year or any ongoing promotions. For example, items that normally aren't bought together might suddenly have a high confidence score during the holiday season due to special offers or the gift-giving nature of the period.
Interestingly, a low confidence score isn't always a sign of a weak relationship between items. It could suggest that there's a small, specialized group of customers with unique preferences that the broader analysis might miss.
The interplay between confidence scores and how much trust customers have in a store or recommendation is also really fascinating. When shoppers receive confidence-based suggestions from a source they trust, they're more likely to make a purchase. This speaks to the complex psychological elements that factor into how we shop.
Retailers can use confidence scores to optimize their store layouts. For example, placing items with high confidence scores next to each other can increase cross-selling opportunities and make the entire shopping experience more streamlined. It's like guiding customers through a logical buying path.
Some studies have suggested that confidence scores are more effective in forecasting online purchases compared to traditional stores. This seems to indicate that e-commerce sites might be able to benefit more from using these metrics to guide their operations.
It's also noteworthy that even with the same confidence score, purchase predictions can vary significantly across different groups of people. Factors like age, how much money they earn, and their overall shopping habits all play a role in how consumers respond to the product associations suggested by confidence scores.
Changes in confidence scores, like sudden drops or spikes, can often point to shifting consumer preferences or broader market trends. This makes them quite useful for keeping track of inventory in real-time and adapting promotional strategies accordingly.
Finally, the combination of machine learning and confidence scores holds a lot of potential. These algorithms can learn from transaction data and continuously refine the confidence scores, making them more accurate and adaptable to changing consumer behaviors over time. This dynamic adaptation can be critical for staying ahead in a competitive retail landscape.
7 Key Metrics for Evaluating Market Basket Analysis in Modern Retail - Lift Ratio Measures Association Strength Beyond Chance
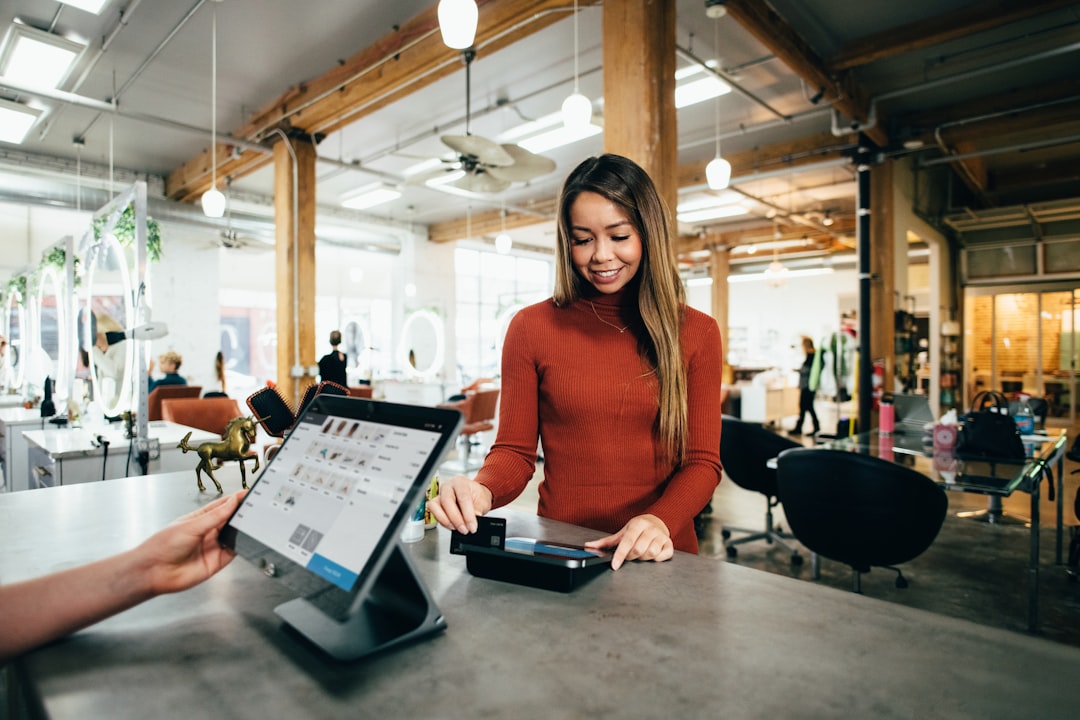
Lift ratio is a valuable metric within Market Basket Analysis (MBA) because it reveals the true strength of connections between products, going beyond simply how often they're bought together. Unlike support, which just shows the frequency of product pairings, lift considers the likelihood of those items being purchased together if they were completely unrelated. When the lift ratio is greater than 1, it suggests a genuine connection exists between those products. Conversely, a lift value below 1 indicates that the products are less likely to be bought together than you'd expect by chance, suggesting a potentially negative or no association. This metric is useful for pinpointing important instances where products are frequently purchased together, and this understanding helps businesses make informed decisions about product placement and marketing strategies.
It's worth noting that while lift is a helpful tool, relying solely on it can be misleading. The meaning of a particular lift value can be influenced by other factors and overall shopping patterns. Therefore, it's best to consider it within the broader context of consumer behaviour and other MBA metrics to fully understand its implications.
Lift ratio is a way to assess how strongly two items are connected in terms of being purchased together, going beyond simply chance occurrences. We calculate it by comparing how often we actually see them bought together to how often we'd expect to see them together if their purchases were totally independent of each other. If the lift is over 1, it means there's a positive link—buyers tend to get those items together more than just random chance would suggest. Conversely, a lift below 1 hints at a negative relationship, where the items are purchased together less often than expected by chance.
It's common to see a lift of 1.5 or more considered a significant indicator of association, but we shouldn't blindly follow such rules. Depending on the products and the retail environment, the threshold of significance can differ greatly. It’s not always that straightforward, though. For example, if a pair of obscure items with extremely low sales has a modest lift, that might seem like a strong link. But in reality, it may not be particularly helpful in guiding promotions or product placement strategies. This is because the sales volume is so low.
The individual popularity of the products also influences the lift. If a very popular item is paired with a niche product, the lift value can be artificially inflated. So, it's always best to keep an eye on both the lift value and the sales volumes of the items in question when making judgments. It's very similar to how correlation and causation can be related but aren't always the same thing.
Interestingly, lift can vary a lot depending on where you are geographically. This showcases how local preferences, cultural patterns, and other regional factors can affect consumer behavior. It reinforces the idea that when strategizing for promotions or retail planning, considering regional nuances is often very insightful.
Furthermore, lift isn't a fixed thing. It can change over time due to seasonal trends, shifting consumer preferences, or external events that affect purchasing habits. Ideally, businesses would keep a close watch on their data in real time to respond to these changes. By closely watching those changes in real-time, retailers are in a stronger position to modify their marketing and inventory adjustments accordingly.
And lastly, relying solely on lift isn't the ideal way to gauge the relationship between products. For a complete picture, we need to look at lift alongside other metrics like support and confidence. By integrating these metrics together, we can gain a more detailed comprehension of how shoppers make their decisions, leading to more finely-tuned retail approaches.
Machine learning has the potential to significantly improve the way we measure lift. Algorithms can effectively utilize large amounts of historical transaction data to better understand shifting customer preferences and calculate lift dynamically. This can aid businesses in making better-informed, real-time decisions that could influence how they sell their products.
Beyond the purely quantitative aspect, lift can offer insights into the psychological side of buying behaviour. High lift values can suggest that consumers develop emotional or mental connections between certain products, leading them to purchase them together. These connections aren't always obvious on the surface but provide deeper insights into consumer psychology.
As a final note, a crucial factor to consider is the concept of diminishing returns. It's important to recognize that an excessive focus on products with very high lift values might not necessarily equate to continuous sales growth or shopper interest. Sometimes, promoting only highly lifted items can harm sales in the long run and we have to think carefully about how these promotions are being used in the mix of strategies being employed.
7 Key Metrics for Evaluating Market Basket Analysis in Modern Retail - Expected Confidence Compares to Random Occurrence
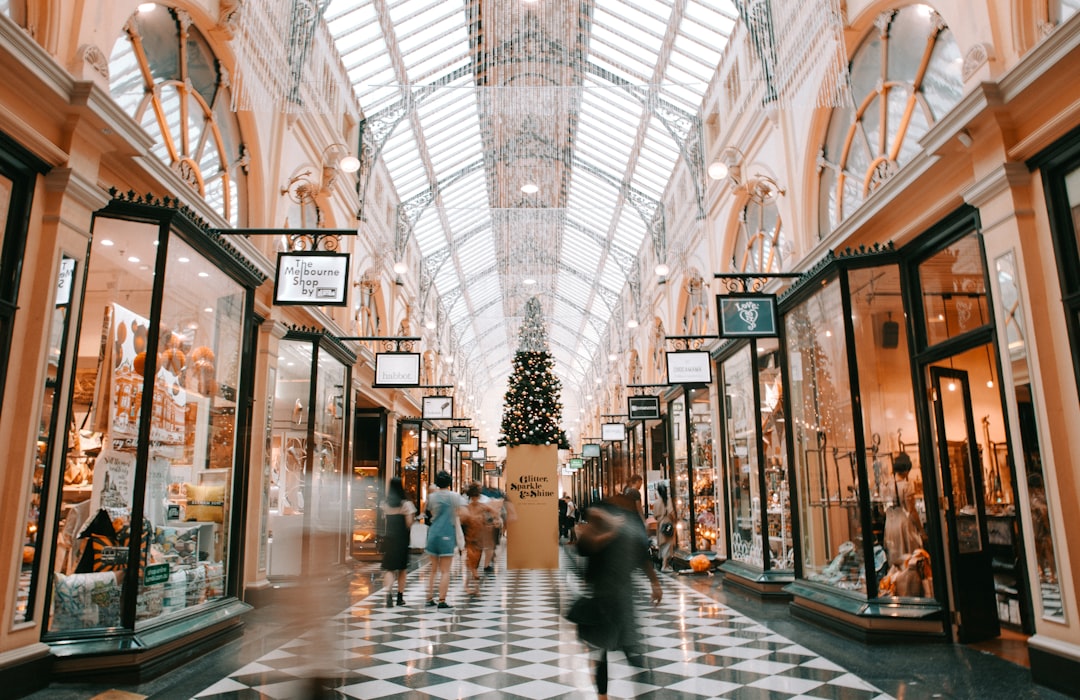
Within Market Basket Analysis (MBA), understanding how confidence scores relate to random chance is crucial. The "Expected Confidence Compares to Random Occurrence" concept highlights the need to evaluate the significance of confidence in predicting item purchases. While confidence quantifies the likelihood of a specific item being bought when another item is purchased, it's important to consider how often this relationship would arise purely by chance. A high confidence score, on its own, might not indicate a genuine and strong association. Instead, it needs to be seen within the broader context of shoppers' actions and considered alongside other metrics, such as lift, for a more accurate picture. This balanced perspective provides a clearer view of purchasing behavior, ensuring retailers don't mistakenly prioritize relationships based on potentially misleading confidence scores and instead, make data-driven choices.
When examining the confidence metric within market basket analysis, we encounter several intriguing aspects related to its practical application and interpretation. The initial choice of a confidence threshold significantly influences what we consider a strong product pairing. While a 50% threshold is often used, adjusting it can unearth more nuanced insights, especially for less commonly purchased items. It's fascinating to note that high confidence scores not only reflect the probability of purchase but can also trigger psychological effects in shoppers. Consumers might be subconsciously drawn to items with high confidence pairings, potentially due to perceived social proof or a feeling that a retailer has expertly curated the suggestions.
This dynamic aspect of confidence scores is further highlighted by the observation that they aren't static entities. During promotional periods or holidays, we often see substantial shifts in confidence scores. For retailers, recognizing and adjusting to these shifts is crucial for designing effective seasonal marketing campaigns. Interestingly, the same confidence score might not translate uniformly across diverse demographic groups. Younger consumers, for instance, may react differently to a particular confidence score compared to older demographics or those with different income levels. A more granular understanding of customer segmentation can inform more targeted promotions, which could potentially increase their overall success.
It's important to acknowledge that even high confidence scores do not guarantee a purchase. It simply signifies an elevated likelihood. Customers might add items to their online carts but later abandon them, introducing complexity into the relationship between confidence and realized sales. There appears to be a stronger correlation between confidence scores and online purchases compared to brick-and-mortar retail environments. This may be due to the more curated and personalized experiences often associated with online platforms. Furthermore, these online experiences can also often be dynamically adjusted based on customer behaviour.
The confidence in the retailer or recommendation platform can profoundly influence how consumers act upon suggestions driven by confidence scores. If shoppers trust a brand or online platform, they are generally more likely to heed its recommendations. This is especially relevant in modern retail, where many shoppers rely on algorithmic suggestions. Unexpectedly, low confidence scores can sometimes be a pointer towards valuable niche markets. If a product is not frequently purchased with other items, it may indicate a dedicated customer segment with specific preferences, presenting a previously untapped market opportunity.
The integration of machine learning algorithms can further refine our understanding of confidence. These algorithms, using a wealth of historical transaction data, can dynamically adjust confidence scores based on evolving customer behavior, yielding increasingly accurate and real-time predictions. Moreover, it becomes evident that regional and cultural factors can subtly alter confidence scores. This emphasizes the importance of adapting marketing strategies to reflect local buying habits. What resonates with customers in one geographical region may not translate to another. Through a multi-faceted approach that encompasses both quantitative and qualitative factors, we can utilize the confidence metric as a valuable tool for analyzing and enhancing retail strategies.
7 Key Metrics for Evaluating Market Basket Analysis in Modern Retail - Conviction Metric Evaluates Rule Directionality
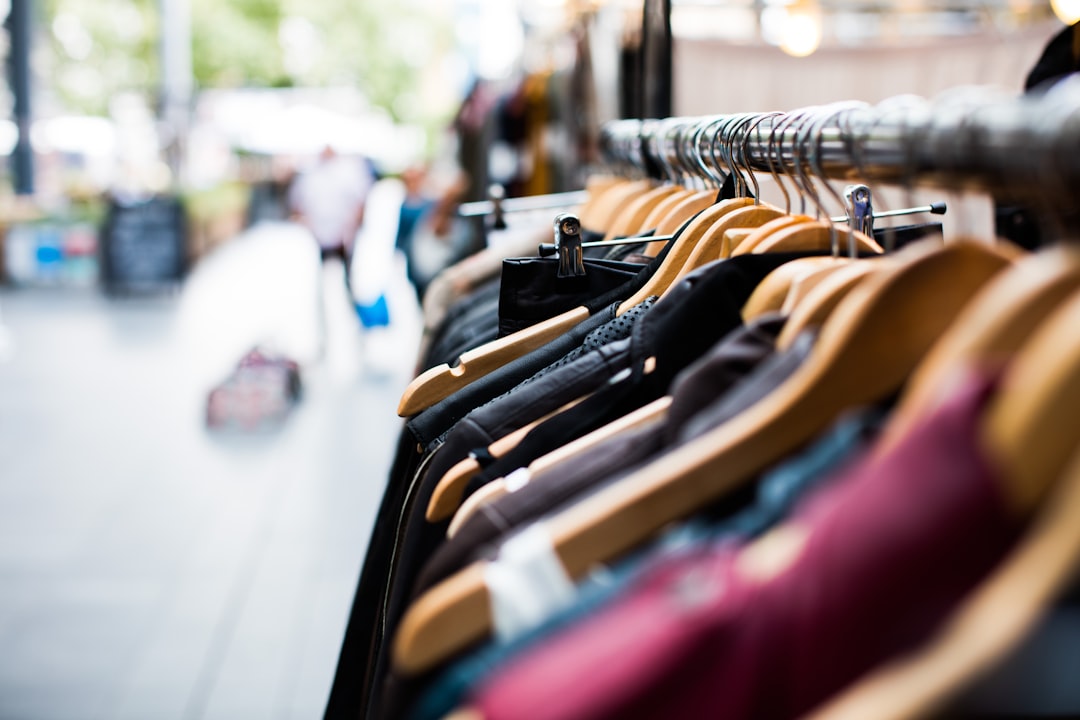
The conviction metric within market basket analysis helps us understand the directionality of relationships between products. Essentially, it measures how much more likely a product is to be purchased when another product is also in the transaction, compared to its usual rate of purchase. This is a valuable distinction for retailers, because it goes beyond simply identifying products bought together. It reveals the strength and reliability of these connections within consumer purchasing habits. By focusing on the conviction metric, businesses can identify which product relationships have the strongest predictive power for influencing consumer choices. This understanding is useful for improving inventory management and crafting more effective marketing strategies. However, it's important to consider the conviction metric along with other metrics to create a complete picture of buying behavior. Relying solely on conviction, without considering context from other metrics, can lead to incomplete or even misleading interpretations.
### Conviction Metric Evaluates Rule Directionality: Key Insights
The conviction metric adds a layer of depth to understanding product relationships in market basket analysis by exploring the directionality of association rules. In essence, it helps us understand how reliably the presence of one item predicts the purchase of another. This is a subtle but important distinction that can be very useful when it comes to crafting more effective marketing strategies.
Unlike simpler metrics like support or confidence, conviction digs deeper into the probability of a rule occurring, factoring in the context of the involved items. It moves beyond just noting whether things are bought together, but whether that association has true meaning. This nuance allows businesses to see if product pairings are truly linked in a way that suggests a predictable relationship.
Interestingly, the conviction metric can reveal insights into customer psychology. High conviction values could suggest that buyers implicitly associate additional trustworthiness or preference with specific product bundles. Understanding these underlying trends is vital for creating promotions that connect with customers on a deeper level.
However, interpreting conviction values can be a bit tricky, as the chosen threshold heavily influences what we consider a strong product association. A 50% threshold might be a common starting point, but adjusting it can reveal subtle insights, especially for less frequently purchased items. This underscores the importance of careful calibration when working with this metric to ensure the findings are genuinely actionable.
Furthermore, the conviction metric isn't fixed; it's dynamic and responsive to market conditions, seasonal fluctuations, and the impact of promotional campaigns. Recognizing these changes allows retailers to adapt their inventory and marketing plans more effectively, helping them stay ahead of consumer trends.
Another fascinating aspect is that conviction can vary widely across different geographic locations. This emphasizes the significance of local preferences, cultural norms, and regional shopping patterns. Businesses can leverage this information to create targeted marketing efforts that resonate more specifically with specific areas.
It's important to remember that both conviction and support are valuable for understanding product relationships, but they do so in different ways. While support measures the raw frequency of product pairings, conviction delves into the implications of the directionality of those relationships. They essentially complement each other, providing a richer understanding of how customers shop.
Analyzing conviction across various customer segments can also reveal valuable insights into niche behaviors. If we see high conviction levels in specific product groups within a particular demographic, it indicates potential opportunities for laser-focused marketing that really hits the mark for those specific audiences.
While the conviction metric is a powerful tool, it's important to recognize that it doesn't tell us about cause and effect. It's still crucial to consider other factors like competition, broader market forces, and external influences that may impact consumer choices. Relying solely on conviction to explain buying behaviour can lead to oversimplified conclusions.
Lastly, combining conviction metrics with sophisticated data techniques such as machine learning can further refine the insights we get. Algorithms can dynamically adjust and adapt the conviction scores over time, delivering increasingly accurate analyses that better reflect real-time consumer behaviour. This dynamic approach allows for more precise and responsive decision-making, helping companies stay competitive.
7 Key Metrics for Evaluating Market Basket Analysis in Modern Retail - Leverage Statistic Quantifies Co-occurrence Significance
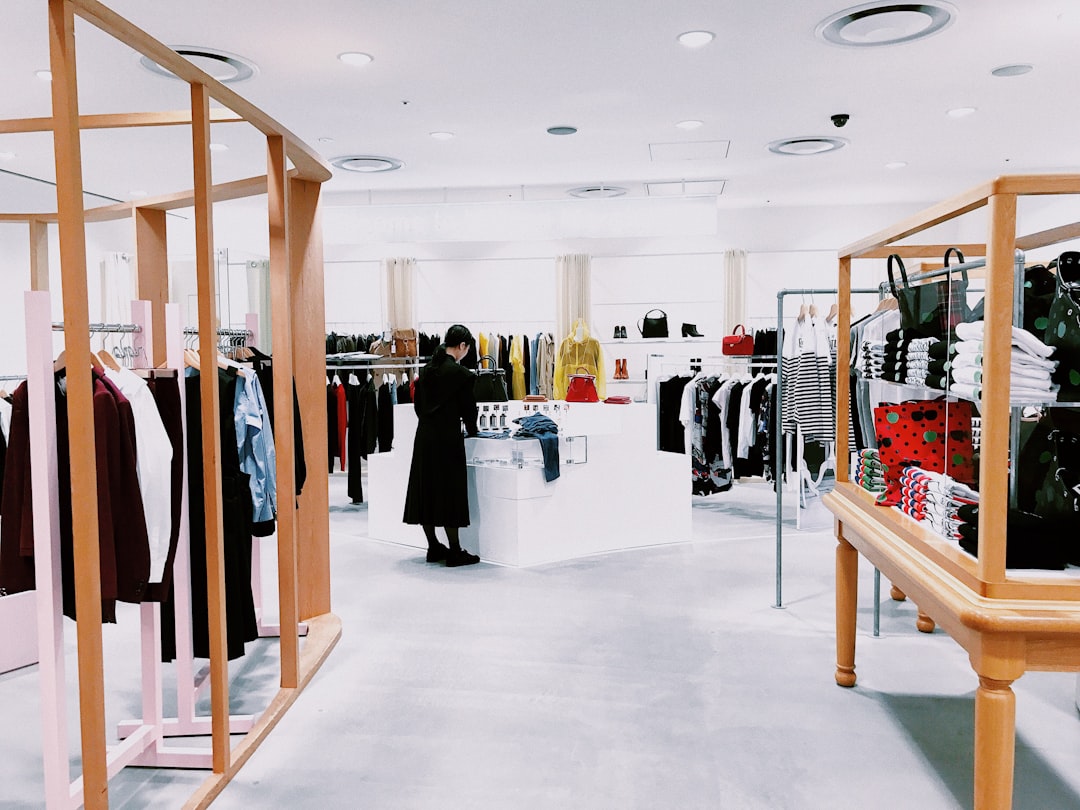
The leverage statistic provides a valuable measure within Market Basket Analysis (MBA) to determine the significance of product co-occurrence. It essentially measures how much more or less two products are bought together than would be expected if their purchases were unrelated. This helps identify those pairings that are truly meaningful, going beyond simply how often they are found together. Retailers can leverage this information to strategize product placement and promotions with greater precision.
However, it's important to acknowledge that using leverage in isolation might not paint a complete picture. For a deeper understanding of shopper behaviour, it should be used with other metrics like support and confidence. This allows for a more holistic view of how products are connected in consumers' minds, which can prove extremely useful in adapting to evolving retail trends. Understanding the interplay between these metrics can help retailers make more well-informed decisions in a fast-changing retail landscape.
### Surprising Facts About Leverage Statistic Quantifies Co-occurrence Significance
Leverage is a metric that helps us understand how much more often a pair of products are bought together than we'd expect if their purchases were completely independent. A leverage value of zero means there's no association between the products at all, while higher values reveal a stronger relationship. It essentially uncovers hidden connections in how customers shop, something that simpler metrics like support or confidence might miss.
One of the most interesting things about leverage is that it focuses solely on the connection between two items. Unlike other metrics, it doesn't consider how often each product is purchased in general. This allows it to unearth surprising relationships that might be hidden if we only looked at overall popularity. This unique perspective on shopping behavior can be quite revealing.
Furthermore, the applications of leverage aren't limited to just product pairings. We can use it to examine larger market trends, pinpoint specific customer segments, and refine marketing strategies to better appeal to different groups of customers or adjust to seasonal changes.
Interestingly, leverage can fluctuate drastically across different regions. This underscores the influence that local culture, traditions, and shopping habits have on how people choose products. Retailers can make use of this regional information to optimize product selections and marketing campaigns to suit those specific markets.
It's also important to understand that leverage, like many other metrics, isn't static. Its values shift depending on external events, such as promotions or shifts in shopper behavior. Monitoring leverage in real-time can help businesses quickly respond to these changes and stay ahead of the curve in a dynamic marketplace.
However, we should be careful not to confuse a high leverage value with a direct cause-and-effect relationship. While it signifies a strong co-occurrence, it doesn't tell us why those items are frequently purchased together. This can lead to inaccurate conclusions if we rely solely on leverage to explain buyer behavior without a deeper understanding of the forces that drive these associations.
When we're evaluating the significance of leverage, it's essential to consider it alongside other metrics, like support and confidence, to get a truly comprehensive picture of how customers behave. Looking at leverage in isolation can be misleading if, for instance, the products being studied aren't very popular in general.
Leverage can also be greatly influenced by unusual shopping instances within the data. For example, a couple of obscure products might appear to have a strong connection if there are just a few instances of them being purchased together. This can be misleading because the overall purchase frequency is so low.
Retailers can use the knowledge gained from leverage to make smarter decisions about managing inventory. This helps them ensure that they have the right products in stock to cater to those frequently paired items. Ultimately, it helps them maximize sales while reducing the risk of stockouts.
Finally, we can further enhance the predictive power of leverage by incorporating it with machine learning techniques. These methods allow us to continuously refine the analysis using real-time transaction data. This flexible approach enables a far more dynamic understanding of how customer tastes and preferences change over time.
7 Key Metrics for Evaluating Market Basket Analysis in Modern Retail - Jaccard Index Assesses Similarity in Purchase Patterns
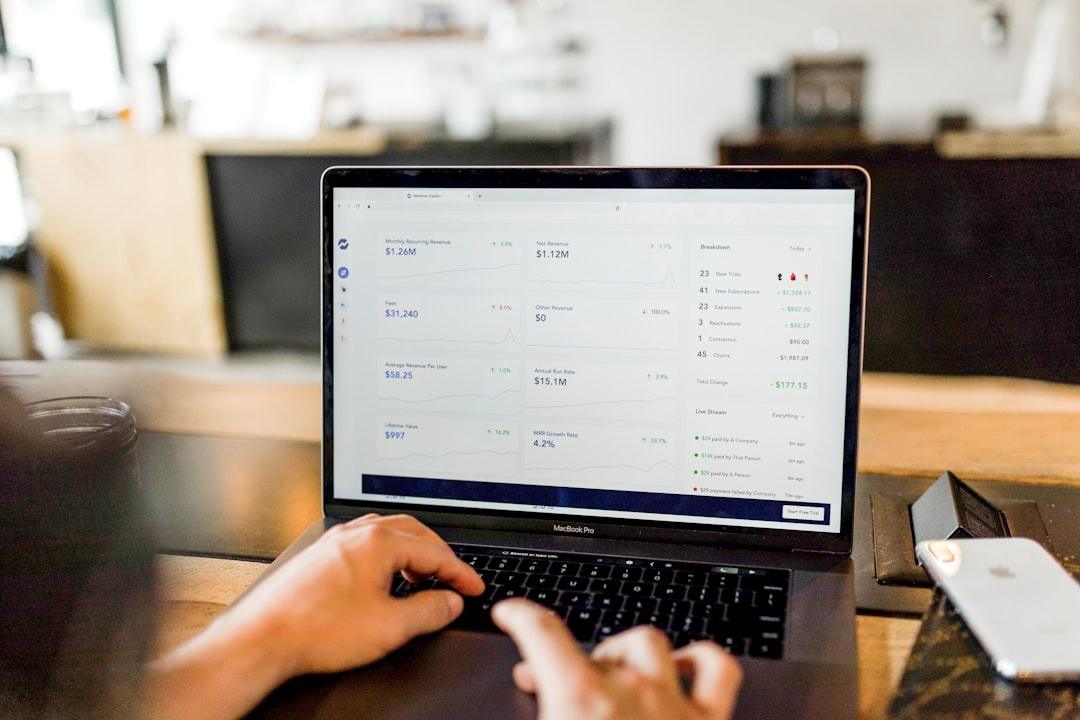
The Jaccard Index serves as a valuable tool for gauging how similar customer purchase patterns are. It works by comparing the overlap of two sets of products purchased, essentially measuring how many items are common between the purchases compared to the total number of unique items purchased across both. The higher the Jaccard Index score (up to a maximum of 1), the more similar the buying habits are. This can be useful for retailers wanting to understand customer segments and anticipate what products might be bought together. It can also reveal hidden associations between products which can inform decisions about product placement, promotions, and inventory management. While useful, it's best practice to consider the Jaccard Index in conjunction with other metrics to fully grasp the complexity of customer choices within a retail context.
The Jaccard Index offers a way to evaluate how alike two sets of purchase data are. Essentially, it calculates the overlap between the two sets and divides it by their total unique items. This makes it particularly useful for gauging the frequency with which product pairs are purchased relative to all the other unique purchases in the dataset.
One intriguing aspect of the Jaccard Index is how well it performs with datasets that have lots of missing data, also known as sparse datasets. Unlike some other metrics, it's specifically designed for situations where many combinations of products simply don't appear in the transactions. This makes it quite useful for digging into those uncommon, potentially unique, customer buying habits.
Furthermore, the Jaccard Index seems to handle noisy or outlier-filled datasets with more ease. Its focus on shared purchases minimizes the influence of less-relevant transactions, thus offering a clearer depiction of actual consumer behavior.
Interestingly, its design makes the Jaccard Index rather sensitive to subtle shifts in purchase patterns. Even a small change in the number of transactions that include a particular combination can significantly change the index value. This responsiveness to change is beneficial for retailers trying to stay on top of emerging trends and adapt quickly to consumer shifts.
Retailers may also find the Jaccard Index particularly useful for understanding how consumer purchasing habits can differ based on their location. It effectively mirrors the distinctive purchase combinations of customers in different places. This opens up the potential for creating more tailored marketing efforts that align better with local preferences.
It's important to note that how we interpret the Jaccard Index depends a lot on the specific threshold we set for what we consider a significant similarity score. We have to be very careful about the interpretation of those thresholds because using arbitrary ones can potentially lead us to incorrect conclusions.
Comparing the Jaccard Index to traditional methods like support and confidence highlights its unique ability to reveal the relative relationships between pairs of products rather than just their individual frequency of purchase. This perspective can be quite valuable.
Despite being a strong analytical tool, the Jaccard Index is also fairly simple to understand and use. This makes it accessible even to retailers who don't have a large data analysis team.
It's also important to remember that a high Jaccard Index doesn't automatically imply that there's a cause-and-effect relationship between two products—it just shows that they're often purchased together. Retailers need to keep in mind other variables like customer motivations and external factors when analyzing results.
Finally, we can leverage the Jaccard Index not only for individual product pairs but also for comparing whole groups of products or even customer groups. This opens the door to getting a better understanding of how markets are segmented and to designing more insightful product bundles that appeal to specific groups of customers.
Transform your ideas into professional white papers and business plans in minutes (Get started for free)
More Posts from specswriter.com: