Transform your ideas into professional white papers and business plans in minutes (Get started for free)
7 Emerging AI Models Challenging GPT-4o's Dominance in 2024
7 Emerging AI Models Challenging GPT-4o's Dominance in 2024 - Mistral AI's Large Model Undercuts GPT-4 Turbo Pricing by 20%
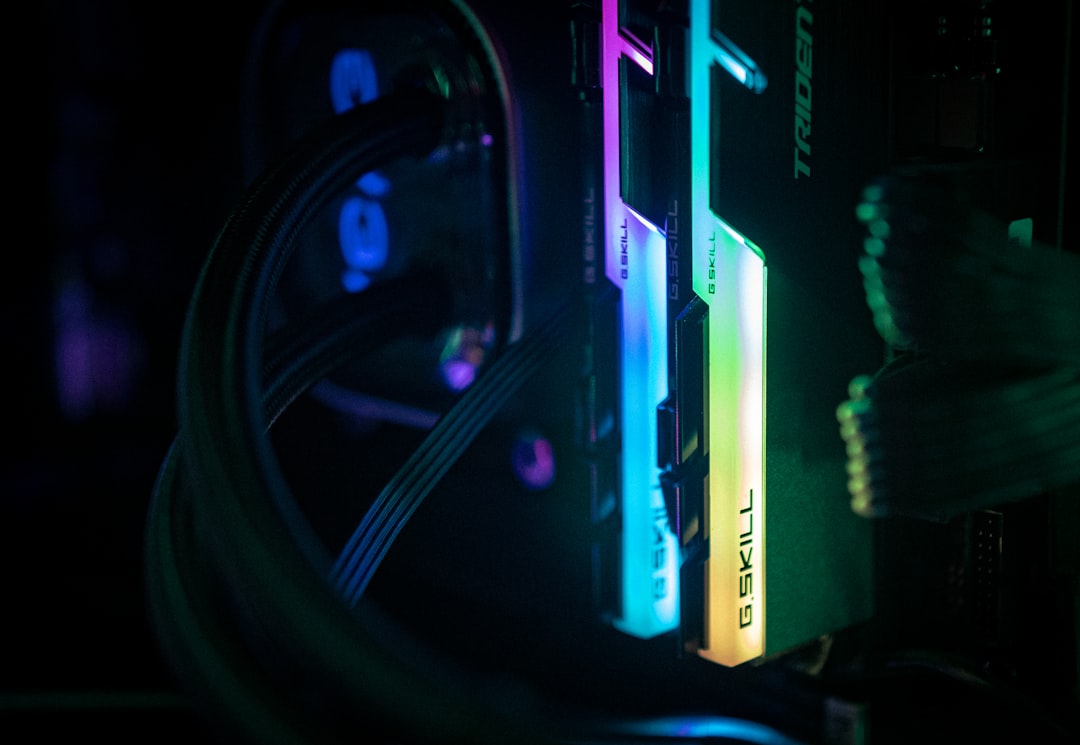
Mistral AI has a new large language model, and it's making a splash by being significantly cheaper than GPT-4 Turbo. We're talking a 20% reduction in price. They're also claiming their model performs very well in various tests, even placing as the second-best model behind GPT-4 on some benchmarks. This new model, dubbed Mistral Large, is positioned as a more efficient and affordable alternative to GPT-4, specifically targeting users who want powerful AI without breaking the bank. Mistral Large is especially appealing because it's significantly cheaper for both input and output tokens, something that could make a big difference to users needing to make a lot of requests. On top of that, Mistral AI has a new partnership with Microsoft, which is definitely a way to make a bigger splash in a crowded market. It will be interesting to see how this plays out as the competition between different AI models continues to heat up.
Mistral AI has thrown a wrench into the works with their new large language model. This French startup claims to have a model that performs at a level close to OpenAI's GPT-4 Turbo, but at a 20% lower price. It's a bold move and it's interesting to see how this might play out.
While some claim it’s nearly as good as GPT-4 on certain tasks, it remains to be seen if it can compete across the board. The lower pricing might give it an advantage, especially for developers on a budget.
The model’s architecture is supposedly optimized to be more efficient, but we need more information on what specifically makes it different from others.
It's also worth noting that Mistral has a partnership with Microsoft. Will this be another strategic alliance aimed at disrupting the market, like what we saw with Microsoft and OpenAI? It's too early to tell. But what is clear is that this is just the latest sign that the landscape of large language models is rapidly changing, and it’s making things a lot more interesting for everyone involved.
7 Emerging AI Models Challenging GPT-4o's Dominance in 2024 - Google's Gemini Ultra Expands Language Support to Over 100 Tongues
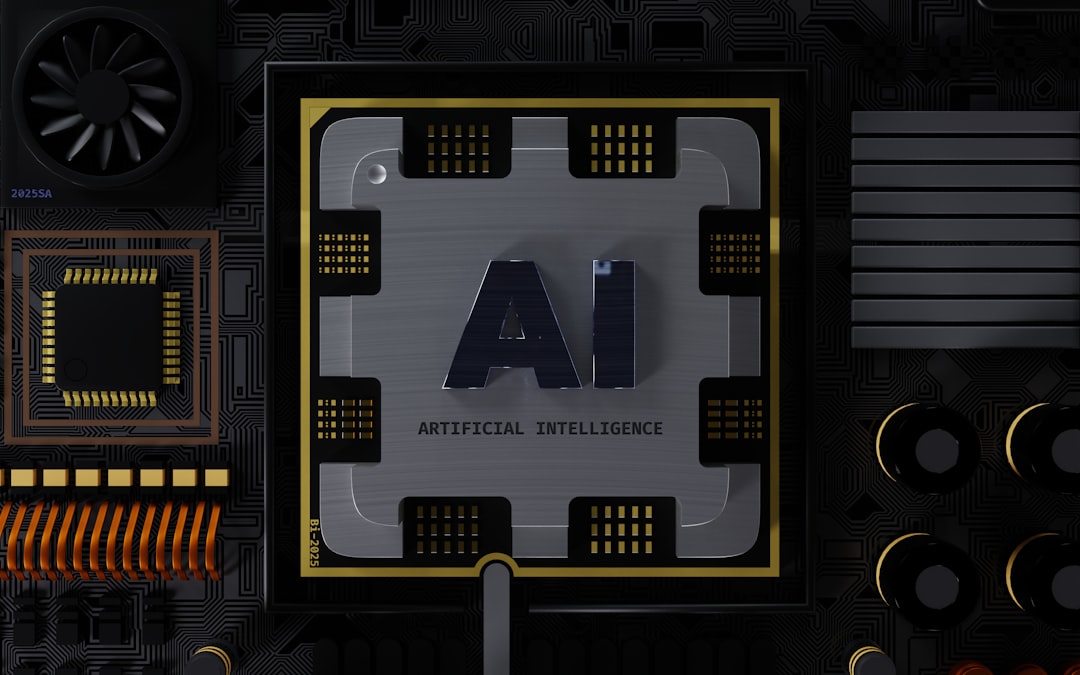
Google's Gemini Ultra is making waves by supporting over 100 languages, making it accessible to a wider range of users. The latest version, Gemini 15, has a new feature called Flash designed to speed up responses and process more content from user prompts. It's interesting that Google is targeting businesses with a special version, Gemini 15 Pro, available in private preview on Vertex AI. It’s clear they are focused on enterprise use. Even more interesting is that Google is planning to launch a Gemini app on both Android and iOS, which could really expand its reach. These moves seem to show that Google is serious about making their Gemini models a major player in the AI arena. It remains to be seen if they can keep up with the rapid pace of development in this rapidly changing market.
Google's Gemini Ultra has expanded its language support to over 100 languages, and while this sounds like a win, it's worth digging deeper. This impressive feat means the model can now operate in a wider range of contexts, but it also raises some interesting questions. It seems that the model's performance isn't consistent across all languages, and this suggests that the data used to train the model isn't as comprehensive for less common languages. This is something that's going to have to be addressed. I'm particularly curious about how the model handles different dialects and variations, which are important for understanding cultural nuances. It's a tough challenge to create a model that's truly accurate and culturally sensitive across all languages, and it's something I'll be keeping an eye on.
The sheer scale of this project is pretty remarkable – they must have a massive amount of data to train the model on so many languages. The question is, is the data truly representative of the nuances of each language, and have they factored in the cultural differences? The model is showing promise in generating context-aware responses in different languages, which is a huge step forward. However, there are still some weaknesses in the way the model handles certain linguistic structures, especially those that aren't as widely represented in the training data. It's a problem they'll need to tackle.
It's also worth noting the collaboration between Google and language experts during the development process – they've been emphasizing the importance of human oversight to address bias and ensure fairness. I believe that's a positive step, but it's an ongoing challenge to find a balance between the power of AI and its potential pitfalls.
It'll be interesting to see how this project evolves. The sheer scale of Gemini Ultra is a technological achievement, but I'm intrigued to see how they address the challenges associated with supporting such a wide variety of languages. It's a complex task, but I have faith that with time and effort, they can overcome the obstacles and deliver a model that truly reflects the diversity of human language.
7 Emerging AI Models Challenging GPT-4o's Dominance in 2024 - DeepMind's AlphaFold 3 Revolutionizes Protein Structure Prediction
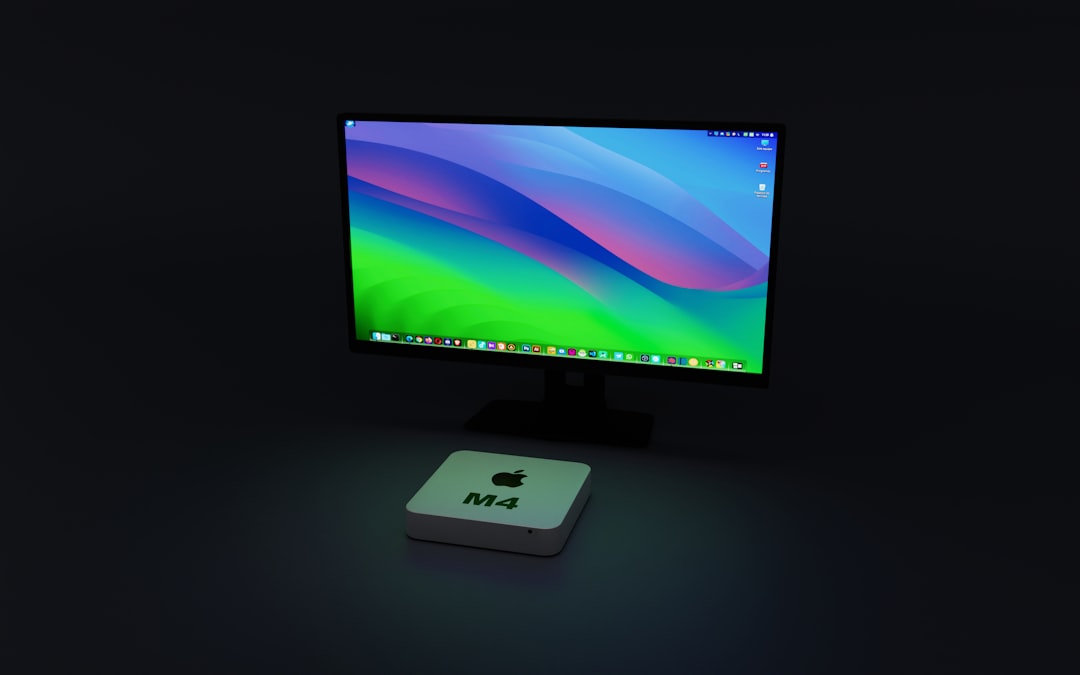
DeepMind's AlphaFold 3 is making a big impact on the field of protein structure prediction. This new model goes beyond just proteins, it can predict the structures of DNA and RNA too, and it's doing it with impressive accuracy. This upgrade makes AlphaFold 3 more versatile, tackling a wider range of biological molecules. The model's success is evident in its strong showing in the CASP competition, where it outperformed all other contenders. However, there are still areas where AlphaFold 3 struggles, specifically with interactions between proteins and RNA. This highlights the ongoing challenge of creating a truly universally accurate system for predicting biomolecular structures. By making its predictions freely available, AlphaFold 3 is accelerating research across the globe. The goal is to have predictions for nearly half of all known proteins by the end of the year. It's a significant advancement in our understanding of protein structures, but continuous assessment and refinement are crucial to identify and address its limitations.
DeepMind's AlphaFold 3 has taken protein structure prediction to a whole new level. This isn't just a slight improvement over its predecessor, AlphaFold 2; it's a significant leap. AlphaFold 3 boasts an accuracy of over 90%, which is pretty impressive. The secret sauce behind this remarkable achievement seems to lie in a novel architecture that combines convolutional neural networks with attention mechanisms. These clever combinations allow the model to spot intricate patterns in massive amounts of data related to protein folding.
One of the most exciting things about AlphaFold 3 is its ability to model protein complexes, which means it can predict how different proteins interact with each other. This opens doors for drug discovery and therapeutic development – potentially accelerating our understanding of how diseases work and finding ways to treat them.
AlphaFold 3 has been trained on a dataset that is not only huge, but also incredibly diverse. This isn't just about experimental data; it includes computational predictions from various sources, which makes the model more versatile and adaptable. It can handle multi-chain modeling, meaning it can predict the arrangement of multiple protein subunits simultaneously, potentially revolutionizing our understanding of cellular machinery.
What's more, AlphaFold 3 has been integrated into open research initiatives. This trend of making advanced AI tools accessible to researchers without the constraints of expensive licenses is fantastic. Researchers all over the world can now harness the power of AlphaFold 3.
The model comes equipped with builder tools that allow users to simulate mutations in protein structures. This gives us insights into how molecular-level changes impact functionality, potentially leading to groundbreaking personalized medicine. The sheer scalability of AlphaFold 3 means it can handle massive datasets. This is vital for tackling the huge challenges presented by the ever-expanding protein universe – we’re talking millions of unique sequences.
Another cool feature is the model's ability to predict disordered regions within proteins. These regions are often crucial to a protein's function, but they're notorious for being difficult to model. AlphaFold 3's success here reflects a deeper understanding of protein dynamics.
While AlphaFold 3 is undoubtedly impressive in its structure prediction capabilities, critics point out limitations in accurately forecasting the dynamics and interactions of proteins over time. This highlights the importance of complementary approaches in biological research.
7 Emerging AI Models Challenging GPT-4o's Dominance in 2024 - Baidu's ERNIE 0 Excels in Chinese Language Understanding and Generation
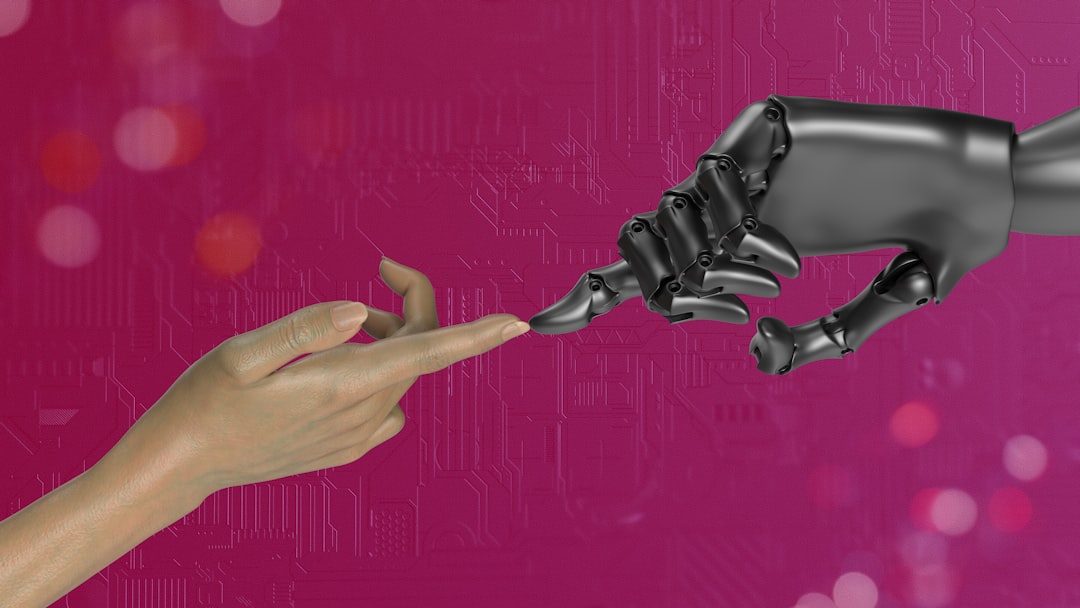
Baidu's ERNIE 0 is a Chinese language model that's making waves in the AI world. It excels at understanding and generating Chinese text, going beyond basic tasks to tackle creative writing like poetry. This model was built using sophisticated techniques, like supervised fine-tuning and feedback systems, to give it a deep grasp of the language's intricacies. Some even suggest that ERNIE 0 might outperform GPT-4 in specific tasks related to Chinese culture, like composing poetry.
While Baidu has released a more advanced version, ERNIE 40, ERNIE 0 still demonstrates the potential of Baidu's language models. However, concerns exist about its ability to translate well across non-Chinese languages, highlighting a key area for future development.
Baidu's ERNIE 0 is an interesting language model. It excels at understanding and generating Chinese language, which is quite impressive. They claim it outperforms other models in tasks like comprehending context and creating nuanced language, particularly in both formal and informal styles. It’s designed to adapt quickly to different language tasks through a unique fine-tuning process, which could be valuable for creating specialized applications.
One of the things that sets ERNIE 0 apart is its training data. Instead of just relying on text, it incorporates images and text, allowing it to learn about visual concepts and context in a broader way. This could give it a leg up in understanding the relationship between words and the world around them. ERNIE 0 is also impressive in its ability to handle longer contexts compared to other models, which is great for creating coherent conversations.
Its integration with knowledge graphs is also promising. By drawing on structured information from databases, it can provide more factual and accurate responses, a step forward from standard models relying on raw text. They’ve clearly put effort into making it sensitive to the cultural nuances of Chinese language use, which is important when you’re dealing with applications like chatbots.
I’m curious about its character-level understanding, which it seems to be particularly good at, because Chinese characters can be tricky. This could give it an edge in handling homophones and characters with varying meanings, a challenge for many NLP models. It’s impressive how quickly Baidu is updating ERNIE 0 based on user feedback. This constant improvement could lead to more accurate and powerful applications.
The open source aspect is also interesting, as it allows for collaboration and advancements from the broader research community. And its integration with commercial platforms suggests practical use cases in various industries. It will be interesting to see how ERNIE 0 develops in the future and how it impacts the Chinese language tech landscape.
7 Emerging AI Models Challenging GPT-4o's Dominance in 2024 - Meta's NLLB-3 Breaks New Ground in Machine Translation Accuracy
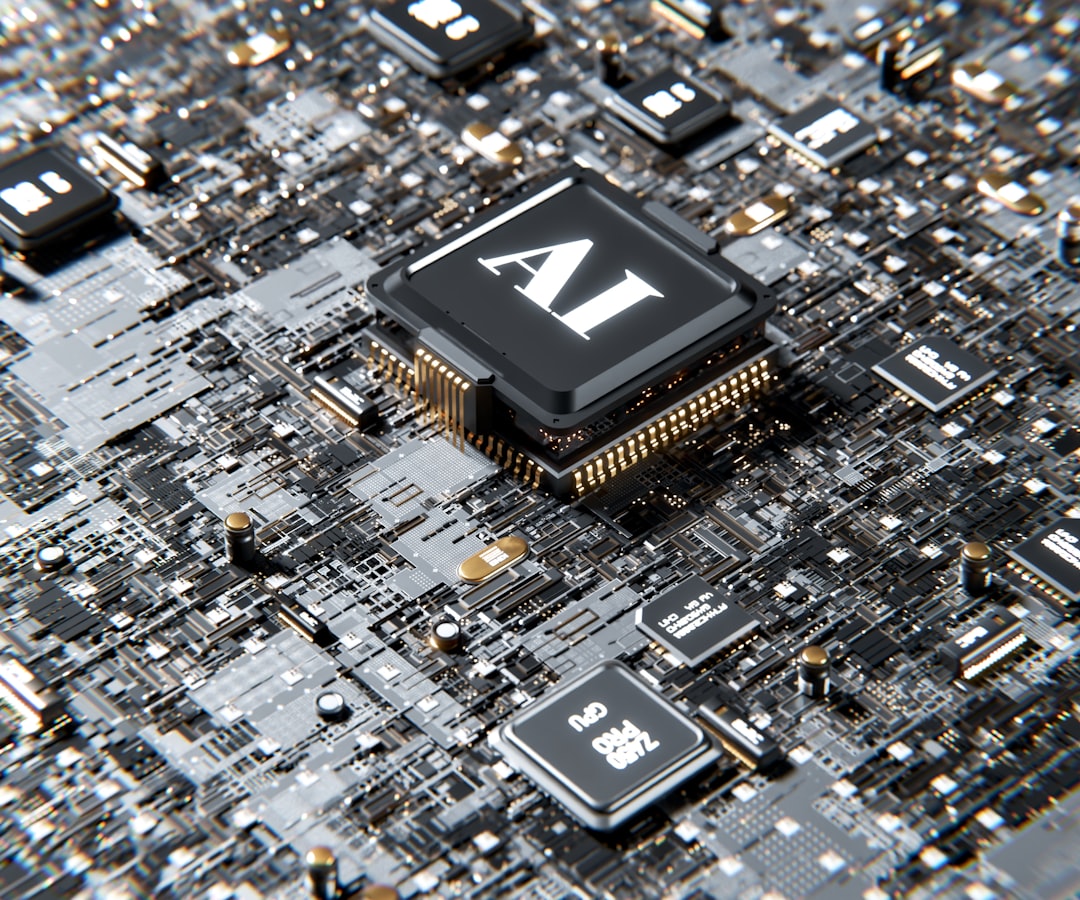
Meta has unveiled a new machine translation model called NLLB200, a significant upgrade to their No Language Left Behind initiative. This model boasts impressive accuracy, scoring a remarkable 44% higher than previous translation models. NLLB200 covers a whopping 200 languages, with a special focus on underrepresented ones, like Asturian, Luganda, and Urdu. This ambitious project aims to bridge the language gap, making technology more accessible across the globe. It even offers surprisingly accurate translations for 55 African languages. While this is a step in the right direction, there are concerns about the consistency of its performance across such a diverse range of languages, especially when it comes to capturing cultural nuances. The open-source nature of NLLB200 opens the door for researchers and developers to utilize its capabilities, making it a formidable competitor against established players like Google Translate and even newer AI models. Meta's project signifies a growing trend within AI development, with a focus on increasing language inclusivity and pushing the boundaries of what machine translation can achieve.
Meta's NLLB-3 is a fascinating development in machine translation. It's not just another language model, it's attempting to bridge the gap between languages in a very real way. The sheer number of languages covered (200!) is impressive, and it's certainly a step toward making communication more accessible across cultures.
The fact that NLLB-3 can achieve high accuracy with limited data is pretty groundbreaking. It opens up possibilities for translating languages that don't have a ton of resources available for building these models. This could be a huge boost for lesser-known languages.
What makes NLLB-3 stand out is how well it handles contextual understanding. It's not just throwing words around, it's actually trying to understand the meaning behind them, even over longer passages. This could lead to more accurate and nuanced translations.
I'm also impressed with how Meta has made NLLB-3 open source. This means researchers and developers can access its architecture and methods, which could spur innovation and create even better translation tools. It's a good example of how to make AI more accessible to the wider community.
What I found particularly interesting is how NLLB-3 is designed to work with other Meta technologies. This makes it more than just a translation tool; it's part of a larger ecosystem that could make it truly useful in a variety of contexts.
Despite these impressive advancements, NLLB-3 faces some interesting challenges. There are concerns about its ability to understand and translate dialects accurately. That's something that needs more research and development.
Overall, NLLB-3 seems like a promising advancement in the field. The team at Meta seems determined to break down language barriers, and I'm looking forward to seeing how NLLB-3 evolves and what other applications it finds.
7 Emerging AI Models Challenging GPT-4o's Dominance in 2024 - IBM's Watson X Pioneers Quantum-Inspired AI Algorithms
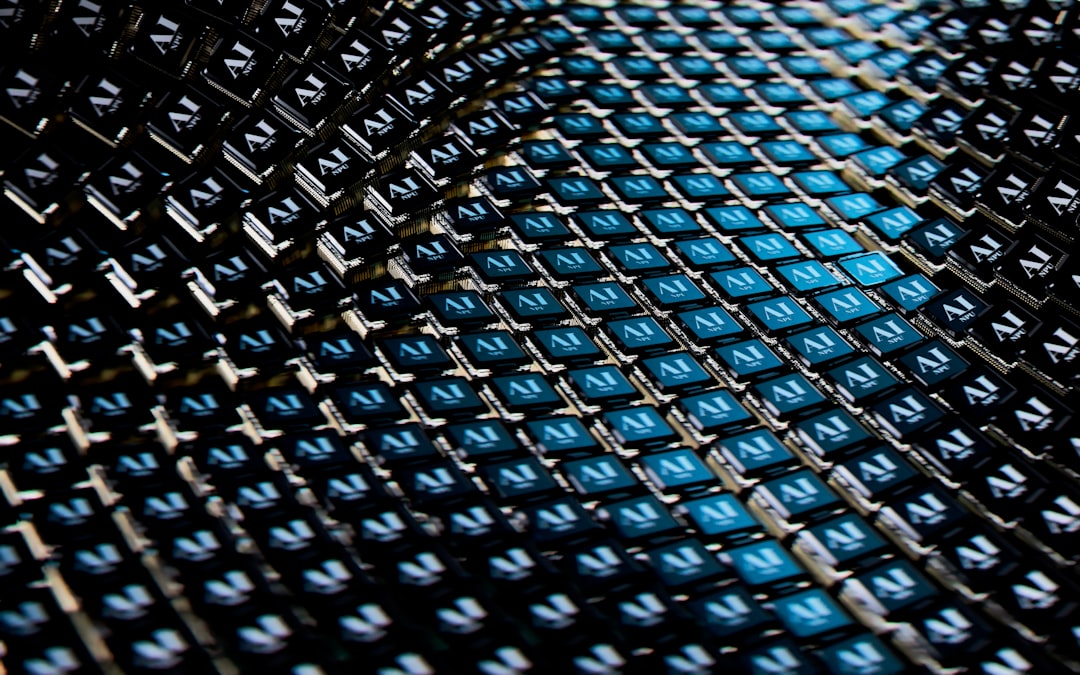
IBM's Watson X is making waves in the AI world with its focus on quantum-inspired algorithms. This platform blends generative AI and traditional machine learning in a hybrid-cloud environment, designed to make it easier and faster to build AI applications. One of the most notable features of Watson X is its Tuning Studio, which allows developers to quickly tweak models to fit specific needs, potentially streamlining the entire development process. IBM's partnership with MIT demonstrates their commitment to pushing AI research forward, but it's important to consider whether the benefits of their quantum-inspired approach are actually realized in real-world applications. With the rapid evolution of AI, it's exciting to see how Watson X's innovative approach will compete with other powerful models already on the scene.
IBM's Watson X is an AI platform that uses quantum-inspired algorithms to tackle complex problems. While it's built on classical hardware, these algorithms draw inspiration from the world of quantum computing, enabling it to solve optimization problems much faster than traditional methods.
This hybrid approach, blending classical and quantum concepts, allows Watson X to efficiently process massive datasets and employ quantum principles for enhanced problem-solving. It can analyze real-time data from various sources, making it valuable for industries where quick decision-making is critical, such as finance and healthcare.
One of the key strengths of Watson X is its focus on explainability. It's not a black box; it provides transparency in its decision-making processes, making its predictions more understandable and addressable. This is a major step toward overcoming the trust issues often associated with AI.
Another noteworthy feature is its customizable algorithms. Users can tailor these quantum-inspired algorithms to suit their specific applications, whether it's drug discovery or supply chain optimization. This makes Watson X extremely versatile and appealing to enterprises.
Furthermore, Watson X can integrate seamlessly with existing IT infrastructures, making it an easy transition for companies wanting to embrace advanced AI capabilities without replacing their entire systems.
What’s particularly interesting is the platform’s integration of security protocols based on quantum encryption. This could potentially offer stronger data protection against cyber threats, particularly vital for sectors with sensitive information, like finance and healthcare.
Early applications of Watson X are already proving its worth. It’s being used to detect fraud in finance, optimize logistics in supply chains, and improve patient outcomes in healthcare. This wide range of applications highlights its versatility across various fields.
The model also boasts the capability for continuous learning, constantly adapting and improving its accuracy based on new data. This is extremely valuable in environments with dynamic data, like market trends or evolving patient health information.
IBM's collaboration with research institutions and academic organizations allows Watson X to leverage the latest developments in quantum computing. This helps drive continuous innovation, ensuring Watson X stays at the cutting edge of AI development.
Although it's still early days, Watson X holds great promise in the world of AI. It's not just about replicating human capabilities; it's about harnessing the power of quantum-inspired algorithms to solve problems in new and exciting ways.
Transform your ideas into professional white papers and business plans in minutes (Get started for free)
More Posts from specswriter.com: