Transform your ideas into professional white papers and business plans in minutes (Get started for free)
The Science of Selling 7 Data-Driven Strategies to Optimize Your Sales Funnel
The Science of Selling 7 Data-Driven Strategies to Optimize Your Sales Funnel - Leveraging AI-powered lead scoring to prioritize high-value prospects
AI-powered lead scoring has become a game-changer for sales teams looking to focus on the most promising leads. By using machine learning and predictive analytics, businesses can shift from a quantity-over-quality approach to a more strategic lead management system. This shift emphasizes understanding how customers behave and what they are looking for, letting sales professionals concentrate their efforts where they're most likely to succeed. This approach, driven by data analysis, makes sales funnels more efficient and conversion rates higher because it focuses resources on the leads with the best chance of becoming customers. However, it's vital that businesses continually adapt and tweak these AI systems, making sure their sales strategy stays relevant as market conditions change.
AI-driven lead scoring offers a new lens through which we can view the sales process, moving beyond traditional, often subjective, methods. These systems, by analyzing vast quantities of data, can predict the likelihood of a lead converting with impressive accuracy, sometimes reaching up to 95%, outperforming older techniques that relied heavily on intuition. Furthermore, by meticulously examining how prospects interact with your content and website, AI reveals behavioral patterns that were previously hidden, enabling us to pinpoint high-potential prospects with greater precision.
AI's ability to cluster leads into distinct groups based on their behaviors and characteristics, such as purchasing habits and demographics, is particularly noteworthy. This capability empowers targeted marketing strategies that cater to specific segments, optimizing outcomes. Unlike conventional lead scoring approaches, which remain static, AI systems continuously refine their models in real-time, adjusting to changing behaviors. This adaptability allows sales teams to act quickly to shifts in prospect interest or needs.
A fascinating aspect is AI's ability to synthesize information from various sources, such as social media interactions, email replies, and website visits, creating a comprehensive picture of each lead. This ‘holistic’ view can reveal patterns that single-channel analyses miss. Moreover, these systems don't just focus on finding new customers; they can also anticipate which existing customers might churn, enabling companies to take preventative action.
Organizations using AI-powered lead scoring report significant efficiency gains, cutting the time spent on low-value leads by 20-30%. This allows sales professionals to focus their valuable time and energy on the most promising prospects. It's not just internal data that AI can incorporate; it can also consider external factors, like economic conditions and industry trends, providing a richer understanding of the context in which prospects make decisions.
The flexibility of AI-powered scoring systems allows businesses to tailor scoring algorithms to their unique needs and target markets. The systems also exhibit remarkable scalability. They can smoothly adapt to growth, maintaining accuracy as customer bases expand and data volumes increase, making sure the approach remains relevant over time. It's important to note that while these tools are powerful, they require ongoing monitoring and adjustments to maximize their effectiveness. The dynamic nature of customer behavior means continuous refinement of the AI models is crucial for ongoing success.
The Science of Selling 7 Data-Driven Strategies to Optimize Your Sales Funnel - Implementing dynamic pricing models based on real-time market data
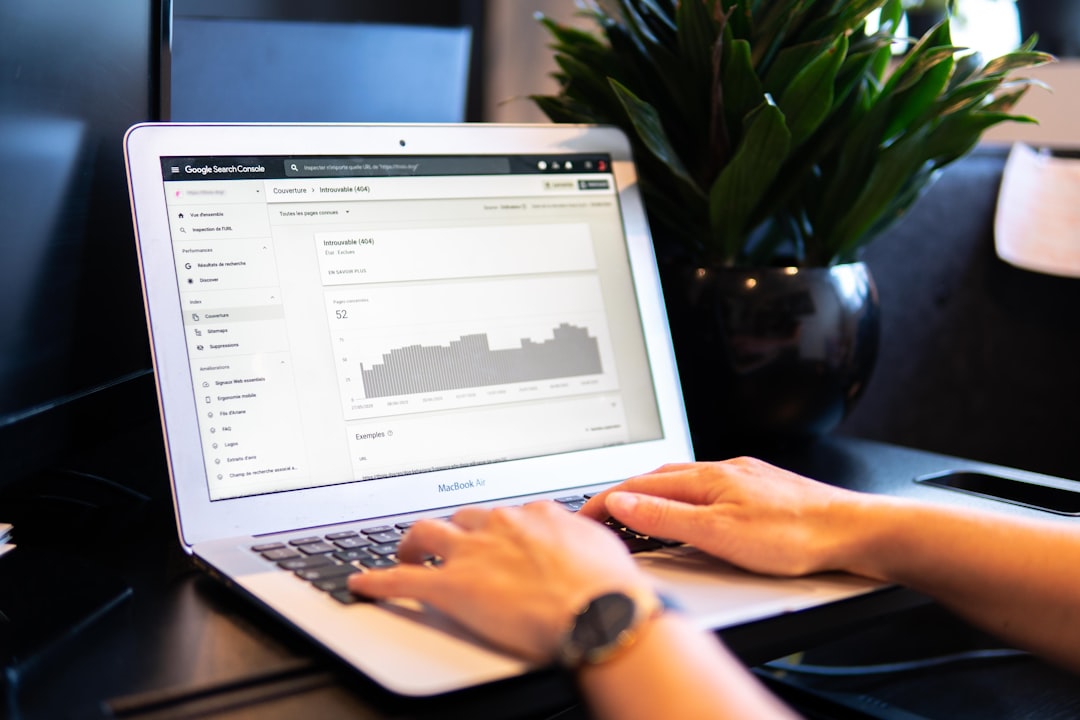
Implementing dynamic pricing models based on real-time market data offers a powerful way to refine revenue strategies. This approach, fueled by machine learning and big data analytics, allows businesses to adapt prices in response to fluctuations in market demand, customer behavior, and competitor actions. It's essentially a continuous process of price optimization, driven by data.
The success of dynamic pricing hinges heavily on the quality and scope of data gathered. Businesses must collect comprehensive data about their costs, market conditions, and customer interactions to effectively inform the pricing algorithms. Without this robust data foundation, these models can be prone to inaccuracies and poor decision-making. It's a delicate balance: effectively maximizing revenue without alienating customers.
The algorithms driving dynamic pricing, often using techniques like reinforcement learning, attempt to predict the optimal pricing strategy at any given time. While this offers the potential for increased revenue, it's vital to be mindful of customer perception. Overly aggressive price adjustments can harm the customer relationship and brand image.
Ultimately, businesses must approach dynamic pricing with a nuanced perspective. It's about finding a middle ground between maximizing revenue and ensuring the strategy remains customer-centric. A well-designed and carefully managed dynamic pricing model can unlock significant revenue potential in the face of constantly shifting market forces.
Implementing dynamic pricing models based on real-time market data is an intriguing approach to optimizing pricing strategies. Essentially, it allows businesses to adjust prices on the fly based on factors like current demand, competitor actions, time of day, and even how customers are behaving. The core idea is to capture more of the potential value from each sale by adapting prices to the specific circumstances.
The power of machine learning is central to these models. Algorithms can continuously learn from a wealth of data – from customer purchase patterns and social media trends to competitor pricing and even broader economic shifts. However, to be truly effective, it’s crucial to have high-quality data about your own costs and profit margins. This helps establish the boundaries within which prices can fluctuate while still remaining profitable.
To understand the impact of dynamic pricing, you can compare your sales performance before and after implementing these models. Using a baseline helps determine if the strategy is generating the desired results. Key technologies, like big data analytics, play a significant role by combining internal data with external sources to create a comprehensive picture of the market.
Dynamic pricing can employ various modeling techniques, including reinforcement learning and Q-learning, which help predict the best price adjustments under different conditions. The effectiveness of these strategies hinges on having comprehensive data about customer behaviour and market trends. The ultimate goal is to achieve flexibility and allow prices to adjust in real-time based on market dynamics.
The algorithms used are fundamentally important to any successful dynamic pricing strategy. They translate the real-time data into concrete price adjustments. While this approach offers the promise of optimized revenue, it’s important to acknowledge that it's not without challenges. There are ethical considerations about ensuring fairness and accessibility, as well as potential negative impacts on brand perception if not implemented thoughtfully. Essentially, the pursuit of profit optimization needs to be balanced with concerns about the broader societal impacts of these dynamic pricing strategies. It's a field with ongoing research, and the question of how to implement it ethically is a vital part of the scientific inquiry.
The Science of Selling 7 Data-Driven Strategies to Optimize Your Sales Funnel - Utilizing predictive analytics to forecast customer behavior and needs
Predictive analytics is becoming increasingly vital for understanding and influencing customer behavior. By using machine learning algorithms, businesses can analyze past data to spot patterns and predict how customers might act in the future. This allows for a more strategic approach to sales and marketing, where resources are used more efficiently and marketing is tailored to individual customers. This personalized approach not only improves the effectiveness of marketing and sales efforts, but can also contribute to stronger customer relationships and increased loyalty.
However, predictive analytics relies on accurate and relevant data. Companies need to carefully collect and curate this data, and continuously update and refine the machine learning models used to make predictions. This ensures that the models remain useful in a dynamic marketplace where customer preferences are constantly changing. As companies gain more experience with using predictive analytics, the potential to gain a detailed understanding of customers deepens, enhancing the possibilities for long-term business growth. The capacity to anticipate customer needs and behaviors is now a critical aspect of modern business strategy, particularly for organizations seeking sustainable growth and success.
Predictive analytics offers a way to peek into the future of customer behavior, essentially allowing us to anticipate their wants and needs. This capability stems from the use of machine learning algorithms that sift through data to spot patterns and trends in customer interactions. By understanding these patterns, businesses can make more informed decisions about where to allocate resources, leading to potentially better outcomes in sales and marketing efforts.
These algorithms can be trained on historical data to identify connections between customer actions and subsequent outcomes. This allows us to build models that can forecast future customer actions, such as the likelihood of a purchase or the potential for churn. These models, in turn, enable us to personalize marketing strategies, optimize resource allocation, and make decisions based on real-time data insights.
While this approach shows promise, it relies heavily on having a solid foundation of high-quality data. Data scientists need to carefully gather and prepare the data to build accurate and effective models. This involves extracting valuable information from a wide range of sources, from customer interactions on websites to social media activity and purchasing history. The quality of the input data directly impacts the quality of the insights we get from the models.
The insights derived from these analyses can have a positive impact on both customer satisfaction and business growth. By understanding customer behavior, businesses can anticipate their needs and tailor interactions accordingly. This type of personalized experience can potentially foster greater loyalty and lead to increased revenue. This ability to personalize interactions allows marketing to become more targeted, moving away from a 'spray and pray' approach to a more nuanced, data-driven system.
However, it's crucial to acknowledge that the world of customer behaviour is constantly in motion. To ensure predictive models remain relevant, ongoing monitoring and refinement are essential. As market trends shift and customer preferences evolve, the algorithms need to adapt to stay accurate. Essentially, the power of predictive analytics relies on continually learning and improving the models used to generate insights. It's a continuous cycle of gathering data, analyzing, making adjustments, and refining the models to stay ahead of the curve. Otherwise, the predictive power diminishes over time.
This method of utilizing data to make predictions about future customer behavior is a fascinating area of research and development. While it has the potential to significantly improve sales and marketing effectiveness, it's essential to recognize that the success of predictive analytics hinges on the quality of data used and the ongoing maintenance of these models. It's a dynamic field, and the process of continuous learning and adaptation is a necessary component of maintaining its effectiveness.
The Science of Selling 7 Data-Driven Strategies to Optimize Your Sales Funnel - Optimizing sales team performance with data-backed coaching and training
Data-driven coaching and training are crucial for maximizing the potential of your sales team. Instead of generic training, a focus on personalized coaching allows you to address the individual strengths and weaknesses of each salesperson. This targeted approach, when combined with ongoing training and a well-structured coaching program, not only improves the team's effectiveness but also creates an environment where constant improvement is valued. Tracking performance through key metrics is essential for understanding progress and making data-driven adjustments to sales strategies. By integrating automation tools, salespeople can spend less time on administrative tasks and more time nurturing customer relationships. This frees them up to do what they do best – build rapport and close deals. However, it is worth considering that over-reliance on metrics can sometimes stifle creativity and adaptability, potentially diminishing the value of more nuanced sales approaches. It’s a balancing act to ensure that data and insights enhance, but do not completely dictate, the sales process.
Harnessing data to guide coaching and training for sales teams offers a more scientific approach to improving performance, moving beyond conventional methods that often rely on guesswork. Research hints that this approach can lead to significant gains in various areas, from individual skill development to overall team effectiveness.
For instance, understanding how individual team members interact with prospects through call recordings and emails reveals behavioral patterns linked to success. This enables managers to tailor training to address specific areas where improvement is needed, making it more targeted and impactful compared to general training programs.
The integration of data analysis into feedback loops provides instantaneous insights into performance, enabling immediate adjustments and learning. This contrasts with traditional coaching cycles, where feedback can be delayed, potentially diminishing its value. Moreover, this approach allows us to test the impact of specific training elements on key performance indicators. For instance, it can reveal if specialized training linked to customer segments produces better results.
Interestingly, the influence of seemingly soft skills like emotional intelligence can be measured and quantified through analytics. Analyzing tone and sentiment during calls offers a way to measure and improve communication skills, leading to enhanced customer satisfaction. Furthermore, incorporating elements of gamification within training programs can boost engagement and foster a more competitive environment.
This data-driven approach also offers a path to increased employee retention. Salespeople who feel supported and are provided with opportunities for growth through targeted training are more likely to stay with a company. Additionally, it can help identify the specific training components most strongly correlated with long-term success, allowing for smarter allocation of resources and focusing on competencies that truly contribute to higher sales figures.
By automating certain administrative tasks, salespeople gain back valuable time that can be redirected to more productive activities, such as directly interacting with customers. Data-driven coaching practices are intertwined with fostering a culture of continuous improvement, encouraging salespeople to regularly refine their techniques and adapt to new information. This continuous learning cycle seems to contribute to higher overall sales productivity.
While still an evolving field, the use of data-backed coaching and training seems to show promise as a way to optimize sales performance. It presents a compelling alternative to traditional approaches by leveraging the power of quantifiable data and targeted training to help teams improve skillsets, better understand customer behavior, and ultimately drive stronger sales outcomes. The ongoing challenge remains to balance the desire for improved metrics with the potential for over-reliance on data, ensuring that the human element and individual skills are not overlooked.
The Science of Selling 7 Data-Driven Strategies to Optimize Your Sales Funnel - Streamlining the sales process using automated workflow analytics
Using automated workflow analytics to streamline the sales process offers a significant chance to improve efficiency and results for sales teams. Technology can automate routine tasks, like inputting data and ranking leads, so sales people can spend more time building relationships with potential customers. Not only does this make the sales process run smoother, but using data analytics helps pinpoint and fix areas where the sales funnel is getting stuck. Additionally, by using tools that analyze information in real-time, businesses can learn more about how leads behave and can separate them into groups, so they can reach out to them in a way that's more likely to be successful and allocate their resources better. However, it's crucial to remember that while automation is helpful, the ability of humans to adapt and be creative is also important. We want to use technology to help the sales process, not replace it entirely.
Automating the sales process using workflow analytics can streamline operations and potentially boost efficiency. By analyzing the flow of tasks and decisions within a sales process, we can pinpoint repetitive or time-consuming steps. Automating these steps could free up salespeople to focus on higher-value interactions with potential clients. Research indicates that such automation can lead to a notable decrease in sales cycle times, possibly by as much as 30%, as tasks are completed more quickly and accurately.
However, achieving accurate analysis is crucial. Automated workflow analytics can improve data accuracy compared to manual entry or record keeping, sometimes by a significant margin, up to 50% in some cases. This increased accuracy benefits sales forecasting and trend identification.
Further, automation can provide insights in real-time. Sales teams can get near-instantaneous data on customer interactions and market changes. This allows for swift responses to inquiries and shifts in demand. Faster response times can, in theory, enhance customer satisfaction and improve sales conversion rates.
Beyond just speed, these tools can segment customer prospects based on behavior instead of demographics. This behavioral segmentation approach leads to more relevant marketing messages tailored to specific actions or preferences of individual prospects. Such targeted efforts potentially achieve significantly higher engagement rates, up to 40% in some studies.
Additionally, these systems can seamlessly connect with a variety of data sources, like websites, email, and social media interactions, to build a holistic view of the customer journey. This comprehensive understanding enables the creation of better sales strategies targeted at specific touchpoints within the journey.
Automation can also improve the accuracy of sales forecasting, possibly increasing it by 20-30%. This greater precision provides better insights for inventory management and financial planning. Moreover, automated systems can create continuous feedback loops. The data from completed sales interactions can instantly inform future sales strategies. This lets organizations quickly adapt their approach based on the success or failure of previous actions.
Furthermore, it's worth noting that automating workflows can lead to operational cost reductions of up to 25%. This cost savings is due to decreased manual work and time spent on administrative tasks. Workflow analytics can also pinpoint sales performance metrics with high precision. For example, we can identify specific actions or interactions that correlate with higher closure rates. These insights guide targeted training and strategy adjustments.
Interestingly, the use of data in sales can positively impact employee morale. Studies show that teams leveraging data-driven insights may experience an increase in team engagement by up to 15%. This seems to be driven by clearer goals and more effective feedback on individual performance. However, while these benefits are promising, it's crucial to balance the use of automation with the need for human interaction and judgment. The relationship between data and sales remains a dynamic and evolving area of study.
The Science of Selling 7 Data-Driven Strategies to Optimize Your Sales Funnel - Enhancing customer retention through data-driven post-purchase engagement
Building lasting customer relationships requires more than just a successful initial sale. Enhancing customer retention after a purchase is crucial, and data can play a powerful role in this effort. By analyzing customer data, businesses can gain a deeper understanding of individual preferences and buying habits, enabling them to design tailored engagement strategies. This personalized approach to post-purchase communication is much more effective than a one-size-fits-all strategy, which can easily fall flat and fail to connect with customers.
The importance of timing in these efforts can't be overstated. Starting to engage with customers immediately after they make a purchase can significantly boost the likelihood of them returning. This early engagement creates a sense of ongoing value and strengthens the customer connection. Additionally, by segmenting customers into groups based on shared traits, businesses can refine their engagement efforts further. This allows them to craft messages and offers that are relevant and meaningful to individual customers, fostering a sense of loyalty and encouraging them to make future purchases.
Ultimately, success in customer retention hinges on the ability to leverage data to provide truly personalized experiences. It requires moving beyond basic interactions and delving into a deeper understanding of customer needs and behaviors. In the current business landscape, this approach is essential to building long-term customer relationships and ensuring business success.
Utilizing data to shape how we interact with customers after they've made a purchase is becoming a crucial aspect of building lasting relationships. By understanding customer behavior patterns and preferences, we can design strategies to re-engage them in a way that feels relevant and valuable. The idea is that a positive post-purchase experience can foster loyalty, leading to repeat business and potentially even stronger connections with the brand.
One of the key findings in this area is that a "one-size-fits-all" approach to post-purchase engagement isn't particularly effective. We've learned that customer segments – groups of customers who share similar traits or purchase patterns – need individual consideration. This means that simply sending a generic "thank you" email isn't always enough. Instead, we can leverage the insights we gain from data to tailor communications, offers, and interactions to the specific needs of each customer group.
Data becomes the foundation for creating these tailored strategies. By examining purchase history, browsing behaviors, and even how customers interact with our support teams, we can begin to build a nuanced understanding of what motivates them and how they prefer to engage with us. This detailed understanding becomes crucial for designing strategies that are truly relevant and likely to resonate with them.
The impact of post-purchase engagement can be substantial. We've seen evidence that it can significantly influence customer satisfaction and, consequently, loyalty. Businesses that create a positive post-purchase experience tend to see higher levels of repeat business and stronger relationships with their customers. However, it's vital to recognize that the timing and nature of this engagement are key. Research suggests that reaching out soon after a purchase, perhaps within a day or two, is more likely to be successful.
Another noteworthy point is the value of existing customers. It's often less expensive to keep a current customer than to attract a new one. Thus, focusing on post-purchase interactions can lead to more efficient use of resources. Businesses can leverage data analytics to identify customers at risk of churning and deploy strategies to retain them before they make the decision to leave. In essence, we can be more proactive about strengthening these valuable customer relationships.
While there's a growing body of evidence suggesting that data-driven post-purchase engagement can be effective, we still need to approach it with a critical and research-oriented mindset. The goal is to make the experience more positive, more personalized, and more efficient. It's a dynamic field with much yet to be understood, and constant vigilance about data privacy and ethical considerations is needed alongside this pursuit.
Transform your ideas into professional white papers and business plans in minutes (Get started for free)
More Posts from specswriter.com: