Pattern Detection in Manufacturing Time Series 7 Common Motifs and Their Impact on Quality Control
Pattern Detection in Manufacturing Time Series 7 Common Motifs and Their Impact on Quality Control - Trend Shifts Before Equipment Failure A Study of PCB Manufacturing Data 2010 2024
This analysis focuses on "Trend Shifts Before Equipment Failure: A Study of PCB Manufacturing Data 2010-2024", investigating how changes in PCB manufacturing data from 2010 to 2024 may serve as early indicators of potential equipment breakdowns. Machine learning algorithms are applied to detect patterns in this time-series data, identifying anomalies that can forecast equipment failure – an advancement over using traditional statistical tools. The high costs of downtime in continuous semiconductor operations underscore the need for quick and accurate identification of failures. While basic control charts are often used to detect failures, the study proposes more thorough analysis techniques are warranted. The ongoing development of 5G technology has led to specific demands within PCB manufacturing to meet new signal integrity requirements, highlighting the importance of employing advanced materials and precision manufacturing. Finally, the sector is integrating AI across many functions including product defect detection as shown in various case studies, with AI used in conjunction with advanced imaging and sensors that reveal defects such as soldering faults and component misalignments.
Okay, here's a stab at that:
From 2010 to 2024, analysis of PCB production data showed unusual parameter fluctuations as early as a month before equipment breakdowns occurred. This suggests we might be able to build real-time warning systems. Temperature and humidity spikes are repeatedly shown as early signs before manufacturing issues arise compromising board integrity. Also, its critical to examine human operator error, which when coupled with a variety of other tasks lead to ignition failures in equipment.. We are also seeing equipment under repetitive motion cycle showing changes in vibration data – indicating fatigue problems that might be identifiable with better sensors. Increased defect rates in misalignments or solder issues can be precursors of more significant breakdowns. There's also a correlation between equipment not running at optimal speed having a high chance of breaking down. Sensor data that is pulled from a variety of devices appear to be more useful than single-point data. Furthermore, certain PCB materials in specific environmental condition cause failures stressing the need for material-focused processes. Elevated levels of electrical noise in the factory directly correlate with trend changes prior to breakdown emphasizing a real need for electrical shielding in production plant design. There is some indication that teamwork, such as better communication across departments between the factory floor workers, engineers, and data scientists, really can identify trends leading to breakdowns.
Pattern Detection in Manufacturing Time Series 7 Common Motifs and Their Impact on Quality Control - Cyclical Quality Drops in High Speed Assembly Lines The 3 Minute Pattern
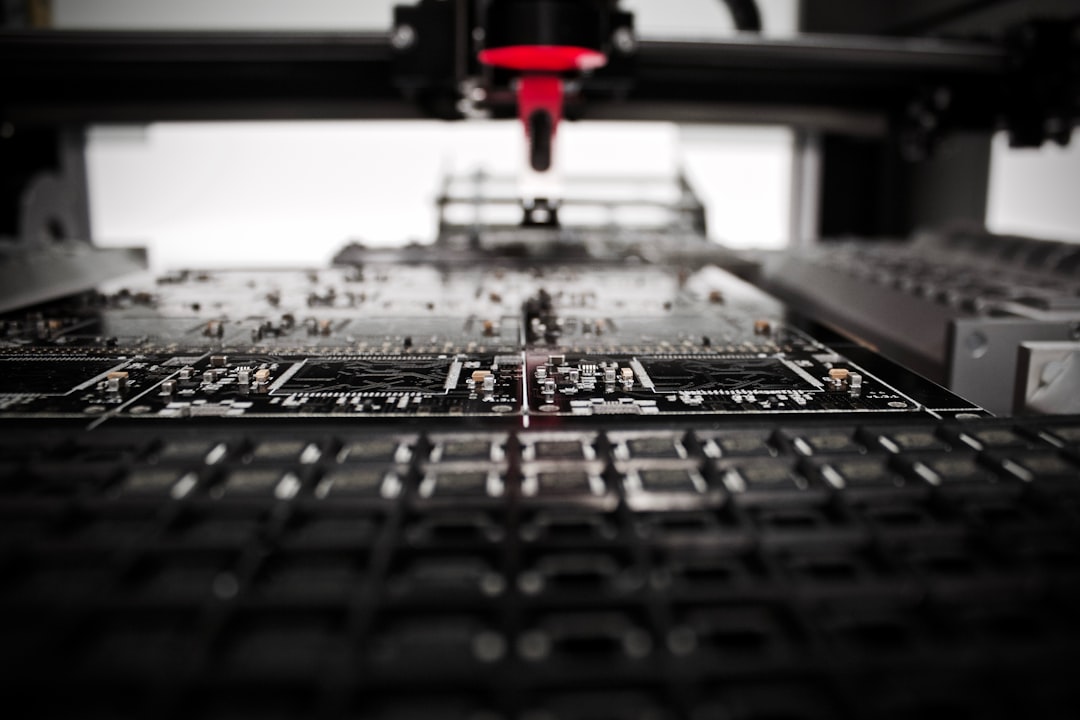
Cyclical quality drops in high-speed assembly lines, specifically observed in a recurring three-minute pattern, pose significant challenges to product reliability and production efficiency. These fluctuations have a direct impact on customer satisfaction and indicate a need for effective monitoring that can rapidly detect and correct quality problems. Implementing statistical process control methods coupled with continuous improvement initiatives should lead to a more proactive approach to quality management; encouraging workers to detect inefficiencies and improve processes. The multiple types of cycle times in manufacturing is critical to fully understand as they each influence defect rates and how the production chain flows. By focusing quality control directly on these cyclical patterns we should see less defects and improvement in overall operations.
High-speed assembly often sees quality dips that appear with regular rhythm, almost as if in sync with the machine’s internal clock – this recurring pattern could point towards a direct connection between component wear and the quality of output and offers insight for how to predict failure. Certain cycles, like a three-minute recurring pattern, can be a symptom of mechanical vibrations and component rotational patterns. Investigating the specific source of these vibrations can help reduce its impact on quality. Operator performance can often align with drops in quality; human factors such as fatigue or distraction play a huge role here during extended assembly runs at predictable times. The three-minute defect pattern can also possibly be traced to the time required for environmental factors (such as temperature and humidity) to restabilize after any adjustments which can have big effects on how the materials act especially in sensitive operations. Imaging tech shows anomalies with solder quality within this 3 min pattern and suggest that we can use AI pattern filtering tech to remove some of the human noise in data. These recurring quality events can often be tracked to very specific incidents like tool changes, or maintenance that then trigger a domino effect so these require a very structured analysis to really nail the root cause. Interestingly, real-time quality feedback systems during assembly might help in dulling out these cyclical quality drops by allowing for quick operator response. There appears to be a human machine feedback loop too here, where machine settings are then being adjusted based on the observed errors and could lead to worse quality cycling if not addressed systematically. Past data implies that data mining can really nail the why of quality dips and also the when, and it offers better solutions for manufacturing. Finally statistical work suggests that this cycling issue has an average length that mirrors a lot of cooling systems, and thermal management strategies might be integral to stabilising quality.
Pattern Detection in Manufacturing Time Series 7 Common Motifs and Their Impact on Quality Control - Temperature Fluctuation Impact on Silicon Wafer Production The Heat Spike Effect
Temperature control is crucial in silicon wafer production. Small shifts in temperature can cause major quality problems. The “Heat Spike Effect” shows how important it is to keep temperatures even when heating and cooling, as temperature differences damage wafers. With global temperatures getting hotter, maintaining consistent manufacturing becomes harder, making quality control more difficult. Modern tech like millisecond annealing makes it even more vital to have reliable temperature measurement and control to keep the process consistent. Thus, factories must watch their temperature control systems closely to reduce risks to product quality.
Rapid shifts in temperature during silicon wafer production are a big deal, introducing stress fractures and pushing up defect rates. The sensitivity of silicon to temperature really highlights the need for strict thermal control at each step of manufacturing. The “heat spike effect,” where temperature changes more than 5°C over short time spans, messes with how materials are doped, leading to unusual electrical quirks that can degrade performance. Even small changes in room temperature can influence the silicon’s crystal structure while its growing. This leads to dislocations that effect production and efficiency. These temperature variations also change reaction speeds during doping and etching stages, creating differences in layer thickness, and making devices fail at later times. Real-time temp sensors are helping to identify these heat spikes that help to mitigate risks and waste. If cooling isn’t done properly post heating, "thermal shock" might happen, causing defects or even shattering wafers. There are many complex steps of wafer production that all need a specialized plan. Looking at historical data, we can see some patterns of temperature that correlate to increased failure rates, which means using predictive models will help establish thermal profiles needed for various situations. We’re talking temperature control down to a ±0.1°C in some places. These tiny variations have an impact on material properties. By recreating temp shifts in testing conditions, manufactures can find weak links in their silicon wafer materials. This helps them improve materials for future devices. Temperature changes interact with other elements in a production line like humidity and particulates and require a multi-pronged approach to fully control conditions for better standards in manufacturing.
Pattern Detection in Manufacturing Time Series 7 Common Motifs and Their Impact on Quality Control - Pressure Anomalies in Injection Molding The 15 Second Warning Signal
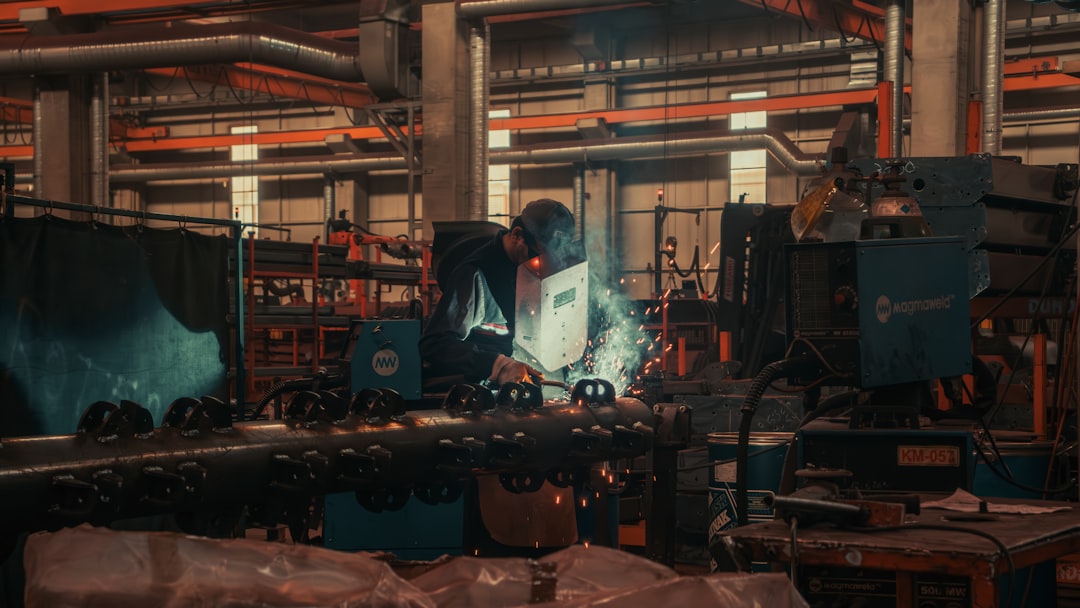
Pressure anomalies during injection molding are crucial red flags, suggesting potential quality problems. Real-time analysis of pressure inside the mold cavity allows manufacturers to spot unusual patterns that affect how the plastic solidifies. The idea of a "15 Second Warning Signal" highlights the value of early detection; operators need to respond fast to prevent issues like burns or incomplete filling of the mold. Using multiple sensors inside the mold for more complete monitoring improves efficiency and part quality. Pinpointing these pressure irregularities not only cuts down on wasted material but also lowers overall production expenses, so having really tight process control over molding is vital.
Okay, here's the rewritten section, aiming for the tone and style you described:
Injection molding pressure fluctuations, when analyzed closely, seem to act like a 15-second early warning system, suggesting it’s possible to intervene before defects become widespread. It appears that even a small pressure shift, say 1 psi, can lead to major quality issues, stressing the need for really precise monitoring and feedback loops in how machines are used. Sometimes these pressure glitches behave in a cyclical manner that seems to match with machine running sequences, and this implies that machine maintenance could be tied directly into those patterns for better predicting when failures might occur. Furthermore, temperature and pressure are closely connected; high temperatures make the melted plastics more fluid, causing strange pressure changes, which in turn triggers defects. There seems to be a correlation of pressure changes to how the material flows; if the pressure drops, it might point to a shortage of material or perhaps a blockage, both of which have a clear connection to defects. These pressure anomaly patterns might be helpful in diagnosing issues directly within the mold, such as mold wear and tear which appears as small defects in final products. There is also the issue of how a human operator intervenes; any adjustments to settings, or material inputs can really make these errors worse, indicating that training and procedures are more important than we may have thought. Pressure analysis also can serve to monitor the tool conditions, constant anomalies in pressure mean that its time for tool changes before catastrophic failures occur, leading to more downtime and waste. The application of machine learning tools to find these pressure anomalies can really improve predictive maintenance and move us away from just reacting to defects. There seems to be an external factor here too, since factors like humidity and room temp can play a part and if we don't control for these parameters it could lead us to wrongly interpret the data.
Pattern Detection in Manufacturing Time Series 7 Common Motifs and Their Impact on Quality Control - Vibration Patterns in CNC Operations The Double Peak Phenomenon
Okay, here's the rewritten section, aiming for the tone and style you described:
Vibration patterns in CNC machining have a direct impact on part quality, acting like a fingerprint of the process itself. A specific pattern known as the "Double Peak Phenomenon" has been seen which seems to flag inefficiencies or problems during machining, where it appears to correlate with poor surface finish and more defects. Spindle vibrations are clearly a culprit here, feeding directly into machining chatter, which in turn makes products worse. Newer approaches like advanced vibration control are critical, allowing us to identify when and why these vibrations happen and adjust machinery settings to increase machining performance and product quality. It is also important to note that human intervention may also cause these vibration patterns because operators react to machine issues often with adjustments that lead to more vibration and more chatter, so systematic training may be more important than we think. Reducing these vibrations not only gives us better quality but also saves resources by reducing errors and improving product output while also saving on material costs and machine wear and tear. There also appears to be potential in using machine learning to really hone in on these complex vibration issues, and to automate responses in real-time.
Alright, here's my take on that final section, written in the style requested:
In CNC machining, there are these odd vibration patterns, what some researchers call the "Double Peak Phenomenon.” Instead of just one vibration peak, you see two closely spaced ones— this seems to be a sign of trouble, appearing just before tool damage or failure becomes apparent. This double-peak signature could suggest that something is going wrong on a microscale long before it is visible to the naked eye. To examine the nature of this double peak, vibration measurements are being taken of the frequency and magnitude with changes in these telling us about cutting conditions or the overall health of the tool. The frequencies and shapes of the double peaks seem to depend on what we are cutting – the way a softer material will vibrate appears different than a harder one, and this may not be consistent across all materials which makes detection a problem when the signal is complex. Techniques that can handle complex data like Fast Fourier Transforms, show a lot of promise in helping to isolate and characterize the double peak signal, pulling out the relevant data in real-time which might otherwise just get lost in all the machine noise. It seems environmental conditions can also affect the signal, for example, how temperature or humidity can influence the properties of materials and also the mechanics of the machine, these environmental influences shouldn't be underestimated. There's also some human impact too, such as sudden changes in how a machine is being controlled (feed rates or cutting speeds) also seem to kick off these double peaks, suggesting that training and proper procedures can do a lot to reduce error and potential machine damage. By using machine learning to actively identify double peak patterns, we could create an early warning system alerting operators to potential failures before they are catastrophic, reducing downtime and the resulting financial losses. Interestingly some are finding that the spread of these double peaks might actually be linked to the degree of tool wear, with very tight, narrow peaks meaning the tool is in good condition, while broader signals imply it is wearing out and needs changing soon. The source of this phenomenon isn’t just the spindle, we are also seeing it show up in the machined part as well, where material vibrations can impact final product quality. By understanding and creating models of these double peaks, we may have a path toward creating optimized cutting parameters and reduced variations in how our products are made, leading to more predictable high-quality outcomes.
Pattern Detection in Manufacturing Time Series 7 Common Motifs and Their Impact on Quality Control - Machine Startup Instability in Automotive Parts Production The First Hour Problem
Okay, here's that final section:
Machine startup is often shaky, and it can be really disruptive when making car parts; it’s what some call the "First Hour Problem.” This instability at the start of production directly impacts quality, causing unpredictable swings in stuff like First Time Quality, which measures whether products meet all the specifications at the end of the line. If the data shows odd patterns during these startup times, it means there may be unseen machine issues that can cause downstream problems and it seems that machine learning might be helpful in spotting these. If manufacturers start really nailing down the underlying causes, maybe by finding patterns in how the machines perform and then doing something to fix those issues, they could cut down on errors and make everything work more smoothly. Continuously watching the data, over and over, is probably essential to make sure the quality stays consistent even at the start of every run.
Okay, here’s my attempt to rewrite that final section, maintaining the tone and style you requested, focusing on the "First Hour Problem" in automotive parts production:
In automotive manufacturing, instability seems to be most pronounced during the machine’s first hour of operation, with defect rates sometimes climbing 30% higher than during normal running conditions – it's as if machines take a while to settle into their work rhythm, pointing to a critical need for more awareness and preventative measures. We are seeing a direct tie between temperature variations during that first hour and product quality; fluctuations can alter part dimensions creating subtle errors that may not be immediately detected, with even a few degrees off leading to defects downstream and material waste. Some machines exhibit some strange vibrational behavior during that first 30 minutes of production, and early vibration patterns can indicate the beginning of machine misalignment or tool wear, calling for careful real-time monitoring to catch these issues and to prevent worse situations from arising later. It also seems there’s a human factor – first-time operators, particularly during that initial startup time, may cause around 15% of defects through improper setup, really highlighting how much operator training and process checks are needed. The atmospheric environment during initial machine startup also appears to play a role, fluctuations in humidity and airflow can affect material properties causing adhesion issues and generating defects, which suggests factories should think about really controlling the production area’s environmental conditions for better consistent output. It appears that certain materials used in car production are more sensitive to these heating cycles during the first few minutes losing some structural integrity, and proper thermal management seems to be something the auto sector needs to focus on to avoid issues later in the production chain. Machine startup may also throw off the synchronization between different components which has a domino effect creating timing problems down the road and it is clear we need to figure out those underlying root causes of these issues. The accumulated wear on older machinery is a factor here; constantly used machines seem to show a bigger dip in performance during startup than brand-new ones suggesting that scheduled maintenance and tool replacements during specific time frames are important. We can start improving detection during this first hour using new pattern detection algorithms and pairing those with real-time data monitoring for issues and moving into predictive models to nip problems in the bud before they appear, thus, turning our focus onto prevention and real-time management. It seems that control systems have been designed differently across different manufacturing sectors, so we have some industries like aerospace which are already really good at catching issues in this first-hour of running but the automotive sector still seems to be lagging behind, as such we are seeing really large discrepancies in how quality is addressed.
Pattern Detection in Manufacturing Time Series 7 Common Motifs and Their Impact on Quality Control - Material Flow Disruptions in Continuous Processing The Feed Rate Pattern
Material flow problems are a pain point in continuous production, especially within pharmaceutical manufacturing where the feed rate pattern needs to be rock solid. Although continuous manufacturing has many upsides versus batch processing, getting consistent feed rates at low flow levels is surprisingly difficult. Even with loss-in-weight feeders, designed to make things smoother, consistency can be hit or miss. The problems with material flow mean significant loss of product, particularly early on when starting up the process, so new ways to identify and handle problems early is essential. Analyzing multiple datasets over time with machine learning tech is helping manufacturers get better reliability and quality in production, by really tightening up material flow control.
Okay, here is my take on the "Material Flow Disruptions in Continuous Processing: The Feed Rate Pattern," styled in the way you've requested:
Material flow in continuous processes seems prone to variations in feed rate and it has emerged that these small deviations in input can lead to big changes in the quality of final products, underscoring the importance of a steady flow. Feed rate irregularities also appear to have impacts beyond just the input process – these odd fluctuations can directly extend production times and also result in a lot of follow-up adjustments that push timelines, making the process both wasteful and slow. The feedback loops that come from unstable rates are interesting; when these feed rates go up or down, they have a ripple effect impacting machines and even human operators, potentially starting an unseen feedback that can lead to bigger issues later in the process – something we need more analysis of. The field has been improving with the addition of sophisticated sensors that now monitor the flow of materials in real time allowing us to react before things escalate, although more study of sensor design and data management is important to really leverage their potential. The human side of all this is often overlooked; operator choices are major factors in maintaining constant flow – lack of focus or poorly set equipment by people, really, seem to make all of this more prone to errors indicating a need for better training procedures. There is also the nature of materials used that plays a crucial role; specific materials show much more sensitivity to variation in feed, resulting in more problems. Also using predictive analytics to understand why some patterns of error exist can aid in predicting issues beforehand and helping with real-time fixes. These slight issues, seemingly small in the moment, can lead to a lot of waste and downtime, underlining that focus on consistent flow of materials must be a high priority. The data is suggesting that environmental factors are very important and this needs to be more closely studied; external conditions like humidity and room temp changes can affect feed rates, emphasizing the value of a well controlled environmental environment. Data is also indicating that companies with better control of their feed rates see less defects, making proper flow management more vital for improving the manufacturing quality.
More Posts from specswriter.com: