How Grammarly's Metadata Impacts Dissertation Plagiarism Checks A Technical Analysis
How Grammarly's Metadata Impacts Dissertation Plagiarism Checks A Technical Analysis - Understanding Grammarlys Document Analysis System
Grammarly's Document Analysis System leverages complex algorithms to evaluate the organization, flow, and general quality of written pieces. It gathers metadata like word counts and readability metrics to improve its plagiarism detection capabilities. This involves cross-referencing user-generated content against a vast repository of online and scholarly materials. Beyond grammatical errors, the system also identifies writing patterns, providing targeted feedback to help writers refine their craft. However, it's crucial to remember that the system might not uncover every instance of plagiarism, especially when substantial paraphrasing is used. In essence, for academic writing, understanding how document structure and its associated metadata are used in the analysis can help writers maximize the benefits this technology offers.
Grammarly's Document Analysis System hinges on sophisticated natural language processing techniques that go beyond simple word matching. It aims to understand the context of phrases and sentences, making it potentially more sensitive to subtle forms of plagiarism. This system is adaptable, capable of analyzing documents of diverse lengths, from concise essays to extensive dissertations, automatically adjusting its analysis to match the specific complexity and scope of the text.
The system's analysis doesn't stop at the words themselves; it also incorporates formatting, structural clues, and stylistic characteristics into its evaluation. This information informs its suggestions for improvements, aiming for a more personalized and refined feedback process. The system incorporates machine learning, consistently refining its insights and recommendations as it gathers more data from user feedback and the diverse document types it processes.
While plagiarism detection is a key aspect, the system doesn't stop there. It also assesses the clarity and tone of writing, providing guidance on how the document might be received by its intended audience. This includes the capacity to detect potential problems with referencing and citation styles across different academic fields, ensuring users are complying with the specific requirements of their field.
Grammarly provides immediate, or real-time, feedback on the document as the user writes, which is a benefit for tight deadlines and fast-paced writing projects. However, it's worth noting that, although adept at identifying linguistic and structural inconsistencies, the system cannot fully grasp the originality of new ideas. This limitation might be significant for academic areas that emphasize unique intellectual contributions.
The software's analytical tools are particularly relevant in collaborative writing scenarios. Grammarly facilitates multi-user review and access to a single document, making it more efficient for groups working on a project or co-writing a dissertation. Perhaps surprisingly, the system goes beyond English, supporting a variety of languages. This expands its usability to a wider group of academic writers, including those who are non-native English speakers.
How Grammarly's Metadata Impacts Dissertation Plagiarism Checks A Technical Analysis - A Comparison Between Grammarlys Metadata and Classic Dissertation Databases
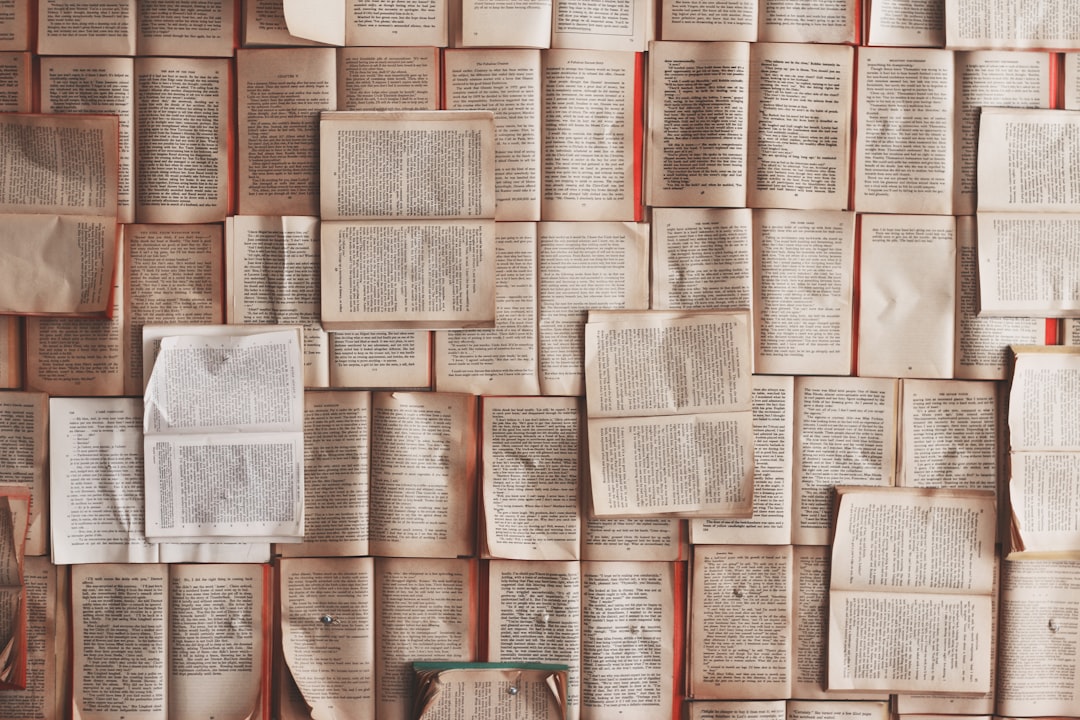
When comparing Grammarly's metadata approach to the traditional methods employed by classic dissertation databases, a notable contrast emerges in their respective focuses. Grammarly's system delves into the specifics of a document's writing style, organization, and overall length to offer writers personalized feedback in real time. This immediate and adaptable nature of the feedback is a core component of Grammarly's approach to improving writing quality. Dissertation databases, on the other hand, generally emphasize storing and cataloging finished academic works, placing more emphasis on citation analysis and adherence to standardized academic formatting.
While both systems strive to reduce plagiarism, their functionalities differ substantially. Grammarly leans towards a more interactive and writer-centric perspective, continuously providing feedback as a document is written. Dissertation databases, however, take a more archival and retrospective stance, often lacking the real-time aspect found in Grammarly. This difference indicates the evolving landscape of academic integrity tools, where the dynamic nature of Grammarly could complement the established, but possibly less agile, systems of classic dissertation databases. The availability of such varied tools suggests that the methods of ensuring academic honesty and upholding integrity are still in flux, and each method has its own unique strengths and weaknesses.
Here's a comparison of Grammarly's metadata approach and that of traditional dissertation databases, focusing on some interesting aspects:
1. **Data Scale**: Grammarly's system thrives on a constantly expanding pool of user-generated text, creating a very large and dynamic dataset. In contrast, classic dissertation databases typically rely on a more limited collection of formally published academic works, leading to datasets that are less fluid and might not be as readily updated.
2. **Immediate Feedback**: Grammarly offers a unique advantage with its real-time feedback feature. Unlike traditional dissertation databases which require you to upload a completed document, Grammarly provides on-the-spot analysis, allowing users to address any potential plagiarism as they write. This can be crucial for fostering integrity during the writing process.
3. **Understanding Context**: Grammarly's use of natural language processing (NLP) potentially gives it an edge in recognizing the context and meaning of text. It could be better at spotting subtle forms of plagiarism, whereas classic databases often rely on keyword-based searches, which may miss more sophisticated plagiarism techniques.
4. **Learning Systems**: Grammarly's use of machine learning means that its algorithms are always evolving based on usage data, leading to continuously improving detection. This is different from the generally static search algorithms found in traditional databases, which can potentially lead to less current plagiarism detection methods.
5. **Beyond Academic Writing**: Grammarly examines a wide range of writing styles and formats, including informal writing like blog posts and business communications. On the other hand, dissertation databases generally focus solely on formal academic writing, potentially missing the diversity of writing styles found in research today.
6. **Beyond Plagiarism**: Grammarly provides feedback not just on potential plagiarism, but also on the clarity, tone, and overall writing quality. This holistic approach allows for greater improvements in a document, not just originality.
7. **Dynamic Metadata**: Dissertation databases typically contain static metadata, such as author, title, and date. Grammarly, however, captures dynamic metadata, like the structure and flow of text, allowing for a more thorough analysis of a document's qualities.
8. **Customizable Feedback**: Grammarly is adaptable to the specific features of a given document, tailoring its feedback based on length and complexity. This flexible approach to analysis is less common in traditional databases, which often use a more generic, one-size-fits-all approach.
9. **Multiple Language Support**: Grammarly's ability to handle multiple languages makes it a useful tool for a wider group of academic writers, particularly non-native English speakers. Traditional dissertation databases are primarily geared towards English, potentially limiting accessibility for international researchers.
10. **Collaboration in Writing**: Grammarly allows for multiple users to collaborate on and edit documents, something that's less common in classical databases. This makes it a more useful tool for group projects, like writing a dissertation with multiple contributors, because it facilitates real-time feedback and edits.
How Grammarly's Metadata Impacts Dissertation Plagiarism Checks A Technical Analysis - Technical Architecture Behind Grammarlys Content Checking Algorithm
At its core, Grammarly's content checking system utilizes a complex blend of machine learning and natural language processing (NLP) to evaluate the quality of written content. By leveraging these advanced techniques, Grammarly goes beyond simple keyword matching, enabling it to grasp the context of sentences and paragraphs. This contextual understanding allows it to not only pinpoint grammatical errors and stylistic inconsistencies but also identify instances of potential plagiarism, even subtle ones. The architecture of this system is flexible, continuously adapting to individual users' writing styles and preferences, thereby delivering more refined and personalized feedback. This adaptive nature is highly valuable in diverse writing situations, including the demanding world of academia and professional communication.
Despite the system's impressive capabilities, including real-time feedback and multi-language support, it's crucial to acknowledge its inherent limitations. While excellent at identifying existing patterns and flagging potentially problematic areas, it cannot always fully assess the originality of completely new ideas. This shortcoming can be a concern, particularly in academic fields that prioritize truly unique intellectual contributions. This system, while powerful, highlights the ongoing need to critically examine its efficacy and explore potential gaps in its ability to comprehensively uphold academic integrity. The development and application of such complex systems requires careful consideration of their potential and limitations.
Grammarly's content checking prowess stems from a sophisticated technical architecture that goes beyond simple grammar checks, especially when it comes to plagiarism detection and writing quality assessments. Here are ten interesting aspects of its inner workings:
1. Grammarly leverages advanced natural language processing (NLP) models, particularly transformer-based ones, to grasp the context and meaning of text. This allows it to identify not just blatant copying, but also more subtle forms of plagiarism like paraphrasing that traditional methods might miss.
2. The system is constantly learning and adapting by drawing data from a wide range of online sources, including academic publications, blogs, and even online forums. This dynamic approach helps it stay up-to-date with current writing styles and trends in real-time.
3. Beyond grammar and plagiarism, Grammarly's architecture incorporates sentiment analysis to gauge the emotional tone of a piece of writing. This helps writers understand how their work might be perceived by their intended audience, making it a more comprehensive feedback system.
4. The core algorithms employ machine learning, enabling them to improve their suggestions over time based on user interactions. The more users utilize Grammarly, the better it gets at providing relevant feedback, creating a virtuous cycle of enhancement.
5. Grammarly can identify patterns of errors that are common among various user groups. This allows it to provide customized recommendations based not only on individual documents but also on the typical challenges faced by particular demographics of writers.
6. The collaborative writing process is supported by Grammarly's infrastructure, which manages version control and tracks changes in real-time. This makes it especially useful for teams collaborating on large projects, such as a dissertation, where multiple contributors are involved.
7. The system includes a built-in reference manager that assesses the accuracy of citations against multiple academic formatting styles (such as APA, MLA, and Chicago). This provides an extra layer of support for upholding academic integrity.
8. Unlike traditional plagiarism detection tools that might primarily focus on academic texts, Grammarly is built to handle a wider range of document types, from business reports to creative writing. This makes it a more versatile tool across various writing contexts.
9. Grammarly tracks key performance indicators related to user engagement and the success rate of error corrections. This data helps the developers measure the effectiveness of the feedback and continuously improve the user experience.
10. Grammarly collects anonymized user data to identify trends in common writing challenges and the effectiveness of its feedback mechanisms. This helps shape future development and ensure the platform remains relevant to current writing practices.
How Grammarly's Metadata Impacts Dissertation Plagiarism Checks A Technical Analysis - File Metadata Transfer Issues During Document Processing
During the process of preparing a dissertation, the transfer of files between different systems or formats can lead to problems with the document's metadata. This metadata, which contains information like the author's name and edit history, can be modified or lost when a file is converted, for instance, from a Microsoft Word document to a PDF. This change in metadata can cause issues when plagiarism checks are performed because differences in this information may trigger false positives, wrongly labeling original content as plagiarized. This problem is made worse by the multiple revisions typically found in dissertation writing. As a result, it's crucial for students to pay close attention to managing the metadata associated with their dissertations to ensure academic integrity is upheld. Recognizing these potential issues and how they influence plagiarism detection tools is necessary for researchers to guarantee that their dissertations are evaluated fairly and accurately.
Document metadata, which includes details like author names, creation dates, and revision history, is crucial for ensuring academic integrity during plagiarism checks. However, the transfer of this metadata between different systems or software can be problematic, potentially leading to inaccuracies in plagiarism detection.
For example, if a document's metadata is not correctly interpreted during a transfer, it could result in incorrect author attribution or misclassification of the document itself. Hidden metadata, like previous edits or comments, can linger within a document and might unintentionally trigger false flags in plagiarism checks. And it's not just about inaccurate assessments—there's also the potential for privacy concerns if a document's metadata contains sensitive user information like usernames or device IDs.
The file format itself can be a factor, as some formats, like PDFs, might strip or alter metadata in unexpected ways, creating inconsistencies in the data available during analysis. This can affect the accuracy of plagiarism checks, as some metadata might be lost or transformed. Timestamps, which are often part of metadata, can also create problems if they're inaccurate due to file transfer issues. This can hinder assessments related to a document's originality or authorship.
Furthermore, software like Grammarly might mistakenly interpret elements like comments or tracked changes as potential plagiarism, resulting in unnecessary concerns for users. Similarly, if a document is uploaded and the metadata is not transferred properly, it could lead to critical elements being overlooked during the plagiarism check, potentially compromising the overall accuracy of the assessment.
Collaborative work environments, where multiple contributors revise a document, can introduce even more complications related to metadata. Accidental alterations to the metadata during these collaborations could make it challenging to pinpoint the original authorship or intention of the document.
Finally, during the file transfer or conversion process, some metadata—like document edits or annotations—might not be fully transferred. This lack of context can make it more difficult to ensure that the plagiarism detection process is operating as intended. If the metadata is not properly processed, it can result in the misapplication of Grammarly's analytical tools, potentially missing nuanced aspects of academic integrity.
In essence, the challenges associated with metadata transfer highlight the complexity of maintaining the integrity of documents during the academic writing process. These challenges necessitate a deeper understanding of how metadata is handled and transferred between different systems, including writing assistance tools like Grammarly. A thorough awareness of these issues can help writers and institutions better navigate the potential pitfalls of metadata inaccuracies in plagiarism detection and support overall academic integrity.
How Grammarly's Metadata Impacts Dissertation Plagiarism Checks A Technical Analysis - Database Coverage Limitations in Academic Paper Detection
The accuracy of academic paper plagiarism detection is significantly impacted by the limitations of the databases used by these tools. Plagiarism checkers, like those incorporated into Grammarly, often rely on a specific set of databases that may not encompass all published material. This can lead to incomplete analysis of a document, as certain sources might be missing from the tool's searchable content. A key concern with this is the potential for false negatives—where plagiarism goes unnoticed because the copied content simply isn't within the database being searched. This problem becomes even more pronounced when examining dissertations, as many institutions maintain unique submission archives that may not be included in the broader databases used by these detection tools. These limitations highlight the need for improved coverage and inclusivity within plagiarism detection databases to ensure a more comprehensive assessment of academic integrity. Ultimately, recognizing these shortcomings is essential for both students and academic institutions to effectively assess and uphold the standards of original work.
The accuracy of plagiarism checks in academic papers, especially dissertations, is challenged by limitations in the databases used. One significant hurdle is how different databases handle and interpret metadata. Discrepancies in metadata parsing can lead to vital information being misunderstood or overlooked, potentially distorting the assessment of a dissertation's originality.
Problems with hidden metadata, like edit histories and revisions embedded in documents, can also arise during file conversions. While these hidden details remain, they might unexpectedly influence plagiarism checks, wrongly suggesting a work is derived when it isn't. The chosen file format plays a role too, as some formats, such as PDFs, might strip or modify metadata in unexpected ways, impacting the algorithm's ability to assess authorship and originality accurately.
Collaborative writing scenarios can also complicate matters. With multiple contributors revising a document, inconsistencies in metadata during editing can obscure the original author's contributions, making it hard to identify the source of each part of the document. The constant evolution of academic papers through revisions creates a dynamic environment where content and associated metadata shift together, making it challenging to maintain the complete context necessary for reliable plagiarism checks. Inaccurate timestamps embedded in files during transfer can muddy authorship timelines, potentially casting doubt on the originality of the presented work.
Along with these practical concerns, there's the loss of revision history during file transfers. This history helps us trace the development of ideas, and its absence can give a distorted view of the original thought process, which could otherwise be factored into plagiarism assessments. Furthermore, plagiarism detection tools may inadvertently process sensitive metadata, including potentially private information about the user or document, raising privacy concerns and ethical questions about the tools themselves.
Adding to these issues, the algorithms themselves might misinterpret legitimate edits or comments as potential plagiarism, leading to unnecessary warnings and anxieties for writers striving to be honest in their work. Lastly, the use of specific metadata standards within different academic fields can create difficulties. If the database used doesn't align with the standard conventions of a particular field, vital aspects of original research could be overlooked, decreasing the overall trust in plagiarism assessments. Understanding the nuances of these database limitations is crucial for researchers, institutions, and writers in ensuring the fairness and accuracy of plagiarism checks within the broader scholarly community.
How Grammarly's Metadata Impacts Dissertation Plagiarism Checks A Technical Analysis - Real World Performance Testing Against Standard University Plagiarism Tools
When evaluating the practical application of standard university plagiarism detection tools, it's clear that, despite their aim to identify text similarities against large databases of published work, no single tool is completely reliable. Real-world testing demonstrates inconsistencies in accuracy across various platforms. Tools such as Grammarly, for example, employ advanced natural language processing (NLP) techniques to better understand the context of text, which can improve accuracy and reduce misidentification of original work as plagiarism. However, challenges arise from differences in the range of resources these tools cover (databases), and how well they process the associated metadata. This is especially problematic when examining intricate academic documents, like dissertations, which can be subject to multiple revisions and have complex formatting requirements. The tests ultimately reveal that human judgment remains essential in verifying the originality of work. A more refined comprehension of how plagiarism detection systems operate and the limitations of their algorithms is necessary to ensure fairness and promote academic integrity.
1. **Accuracy of Plagiarism Detection:** Studies suggest that conventional plagiarism detection tools, while helpful, generally only identify around 80-90% of plagiarized content. This indicates a persistent challenge for writers relying on these tools to ensure their work is truly original.
2. **Database Scope and Coverage:** Traditional dissertation databases may not cover a wide range of sources, such as unpublished theses or student projects. This is a crucial point, as plagiarism could originate from these less easily accessible documents, potentially leading to missed cases of plagiarism (false negatives).
3. **False Positives from Revisions:** The metadata associated with document revisions, such as edits and comments, can sometimes trigger false alarms in plagiarism checkers. When a document has gone through numerous revisions or includes annotations, the tool might misinterpret these as evidence of copied material, leading to unwarranted concerns for the writer.
4. **Dynamic vs. Static Databases:** Grammarly's approach allows it to adapt to current writing styles through constant updates to its databases using user-generated content. However, traditional databases often lack this ability to quickly incorporate new information, potentially making them less adept at catching newer forms of plagiarism.
5. **Impact of Hidden Metadata:** Comments, tracked changes, and other concealed information within a document can unintentionally reveal details of the writing process. If this data isn't properly managed during plagiarism checks, it can lead to misinterpretations about the document's originality.
6. **Challenges with Timestamp Accuracy:** File transfer processes can introduce inaccuracies in timestamps associated with a document. This can cause problems for plagiarism detection systems trying to understand the chronological development of the document. This issue makes it more complex to determine authorship, especially in documents written by multiple people.
7. **Language Limitations in Databases:** Many traditional databases focus primarily on English language texts, resulting in gaps in coverage for academic work in other languages. This bias can negatively impact writers using languages other than English, as potentially plagiarized content in those languages might go undetected.
8. **Collaboration and Authorship:** In situations where multiple individuals contribute to a single document, the various revisions and edits can introduce inconsistencies in authorship identification for plagiarism tools. This can make it difficult to establish the origin of particular sections, impacting the integrity assessment.
9. **Impact of Formatting Changes on Metadata:** Modifications to a document's formatting, especially during conversions (like from Word to PDF), can disrupt the transfer of metadata. These changes can influence how plagiarism tools analyze content, potentially resulting in incorrect detection outcomes.
10. **Limitations in Content Matching:** Certain plagiarism detection algorithms rely heavily on directly matching content with their stored database sources. If the source material is paraphrased or not included in their indexed resources, the tool might fail to recognize it as plagiarized, creating a false sense of security for the writer.
More Posts from specswriter.com: