Transform your ideas into professional white papers and business plans in minutes (Get started for free)
How Distributed Computing Powers Modern Weather Forecasting Systems in 2024
How Distributed Computing Powers Modern Weather Forecasting Systems in 2024 - GraphCast Desktop Computing Transforms Weather Forecasting Speed
GraphCast is reshaping how we approach weather forecasting, leveraging desktop computing to achieve previously unimaginable speeds. This new AI-driven model, trained on a vast repository of historical weather information, excels at predicting severe weather events with remarkable accuracy within a mere minute. Unlike traditional forecasting systems, GraphCast's advanced machine learning algorithms not only deliver results faster but also consistently outperform established benchmarks like the ECMWF models. The speed and precision improvements offered by GraphCast could drastically change how communities prepare for and mitigate weather-related issues, representing a noteworthy shift in meteorological science. Its ability to adapt to changing weather patterns positions it as a potentially invaluable tool for informed decision-making across numerous fields, bolstering society's resilience to climate fluctuations. While the model's performance is impressive, concerns about its long-term reliability and potential biases remain an area requiring ongoing research.
GraphCast's design hinges on desktop computing, which has radically altered the speed of weather forecasting. It's been trained on a massive dataset of historical weather patterns, allowing it to generate highly accurate forecasts in a remarkably short time. While traditional weather models rely on intricate numerical computations that consume substantial computational resources, GraphCast employs advanced machine learning, enabling predictions in under a minute. This speed has proven invaluable, particularly for anticipating severe weather events, where swift responses are crucial.
Tests against established models like those used by the ECMWF have shown GraphCast achieving superior results across a wide range of weather metrics, including temperature, pressure, wind, and humidity. This leap in accuracy has brought about a paradigm shift in the field of weather prediction, pushing the boundaries of our ability to understand and anticipate weather systems.
Interestingly, the model's inherent ability to learn from past data means it constantly adapts and refines its forecasting capabilities, becoming more precise over time. This adaptability is vital as weather patterns shift and change, presenting a challenge for traditional models. Though GraphCast showcases significant potential, challenges remain in integrating it seamlessly with existing tools and ensuring clear and consistent communication of its outputs to users. It highlights a broader trend—the growing reliance on distributed computing, rather than centralized supercomputers, to handle computationally intense tasks like weather forecasting. This paradigm shift will likely impact the landscape of meteorological services in years to come. GraphCast, ultimately, represents a substantial stride forward, demonstrating the growing role of AI and desktop computing in revolutionizing our understanding and forecasting of the world's weather.
How Distributed Computing Powers Modern Weather Forecasting Systems in 2024 - Parallel Processing Networks Enable 15km Grid Resolution
The use of parallel processing networks is a crucial factor in enhancing weather forecasting by enabling a 15km grid resolution. This finer resolution level significantly improves the accuracy of localized weather predictions. By capturing more detailed atmospheric patterns, forecasters can better anticipate severe weather events and prepare accordingly. This advancement is largely due to the increased power of high-performance computing systems, which handle vast amounts of data at incredible speeds, mitigating the limitations imposed by both high computational demands and inherent uncertainties within the atmosphere.
As weather forecasting techniques become more sophisticated, the role of parallel computing continues to grow. This is especially true given the growing integration of machine learning into traditional models, which constantly refine forecast accuracy over time. The shift towards distributed computing architectures within the field highlights a broader trend, with these networks set to reshape the future of weather prediction, offering a more comprehensive and responsive forecasting process.
The ability to leverage parallel processing networks has been a game-changer for weather forecasting, particularly in achieving higher resolutions in weather models. We can now generate simulations with a 15km grid resolution, which is a significant leap forward. This improved resolution is crucial because it allows meteorologists to capture more localized weather patterns, like thunderstorms, which were previously too detailed for traditional models. These advancements were not possible without significant developments in how computers work together.
While traditional models relied heavily on centralized supercomputers, the shift towards distributed computing, including cloud-based solutions, has opened up new possibilities. These architectures allow computing resources to be allocated dynamically based on the needs of the forecast. This flexibility can lead to improved efficiency and significantly reduced lag time, which is crucial for fast-changing weather conditions. It means that instead of waiting hours for a forecast, models can generate results in a matter of minutes, and potentially have a wider impact on the public and emergency response teams.
Parallel processing also allows us to ingest massive amounts of data from various sources, including satellites, radars, and ground stations. This data fusion, if you will, leads to a much more accurate representation of current weather conditions, making the forecast more reliable. It's not just about faster computation, though. Parallel processing also increases the amount of data that can be processed. This enables more comprehensive analysis of weather patterns and potentially reveals previously unseen insights.
Further, with the power to run thousands of simulations concurrently, we can now apply ensemble forecasting techniques more readily. These approaches consider a range of possible weather scenarios, creating more robust and resilient forecasts that are less likely to be thrown off by unforeseen events or uncertainties within the atmosphere. However, it is important to note that even with a 15km grid resolution, it can be challenging to fully capture very small-scale events, which we often refer to as subgrid phenomena. These limitations can introduce some uncertainty in localized predictions.
The potential implications of using parallel processing in weather forecasting stretch far beyond just improved predictions. The success seen here highlights how similar techniques could be adopted in other computationally intense fields, including seismology and oceanography. It could be a crucial step in fostering the next generation of powerful analytical tools. It's a testament to the innovative ways we are employing technology to deepen our understanding of complex natural phenomena. It's also a reminder that while parallel processing brings remarkable capabilities, further development and refinement of techniques are needed to continually improve forecasting accuracy and address remaining uncertainties.
How Distributed Computing Powers Modern Weather Forecasting Systems in 2024 - Machine Learning Integration Reduces Forecast Processing Time by 40 Percent
The integration of machine learning into weather forecasting has led to a notable reduction in forecast processing times, with some systems achieving a 40 percent decrease. This improvement is linked to the increasing reliance on more advanced computational methods that augment traditional forecasting approaches. The acceptance of machine learning within the meteorological community, along with its ability to quickly process large datasets, has boosted operational efficiency, especially when responding to rapidly evolving weather situations. However, the successful implementation of machine learning models in weather forecasting requires substantial computational resources and ongoing refinement of the algorithms themselves to guarantee accurate and dependable results. This growing trend of incorporating machine learning represents a substantial change in how weather predictions are made and used, underscoring the need for continuous research and adaptations within the field to optimize performance. While promising, there are also lingering concerns regarding long-term reliability and potential biases within these new systems.
The integration of machine learning into weather forecasting systems has demonstrably reduced forecast processing time by a remarkable 40%. This acceleration in processing is particularly crucial during severe weather events, where swift responses are paramount. It's a testament to how machine learning can not only enhance the speed of predictions but also contribute to their overall accuracy. Traditional forecasting approaches, while robust, can often take hours to generate and update forecasts. In contrast, the optimized machine learning algorithms can refine and deliver near-instant updates, which can drastically influence community response times.
This 40% reduction in processing time is largely attributed to the ability of machine learning models to efficiently manage the vast and complex datasets inherent in weather forecasting. By streamlining computational tasks, these algorithms can navigate intricate atmospheric patterns and variable interactions much more effectively than traditional methods. However, it's worth noting that this speed enhancement doesn't compromise the models' adaptability. Machine learning systems are inherently designed to continuously learn from new data, enabling them to dynamically adjust predictions and adapt to the rapidly changing nature of weather systems.
The capability to analyze extensive historical weather data has led to significant improvements in forecasting accuracy. Machine learning models excel at identifying subtle patterns within this data, patterns that might otherwise be missed by traditional models due to the complexity of the interrelationships between atmospheric variables. This suggests a potential for uncovering previously unknown forecasting insights. Moreover, these systems can seamlessly integrate real-time data from diverse sources, creating a more comprehensive picture of current weather conditions. This holistic approach leads to more reliable forecasts overall.
One aspect of maintaining machine learning models' effectiveness that requires careful consideration is ongoing training with updated datasets. Without continuous retraining, the models risk becoming stagnant and failing to adapt to evolving weather patterns, diminishing the value of their predictions. While the promise of rapid processing speeds is enticing, challenges remain in integrating these models with existing meteorological infrastructure. Developing robust data interpretation techniques is crucial to ensuring user understanding and fostering trust in the machine learning-generated outputs.
A key point of discussion revolves around the underlying principles of these machine learning models. They primarily rely on identifying correlations within historical data rather than explicitly representing the physical processes governing atmospheric dynamics. This raises some legitimate concerns about whether these models might produce seemingly accurate predictions that don't accurately reflect the underlying physical mechanisms. It is important for researchers to continuously assess whether there are inherent biases in the data, or if assumptions embedded in the models can lead to inaccuracies in certain scenarios.
As machine learning continues to become increasingly integrated into weather forecasting, it's essential that the field prioritizes ongoing validation and testing against established models. This approach can help ensure the reliability of machine learning forecasts and address any potential biases or unforeseen limitations. Maintaining a balanced perspective, where the strengths of machine learning are leveraged while being aware of its limitations, will be key to fostering more accurate and robust weather forecasting in the years to come.
How Distributed Computing Powers Modern Weather Forecasting Systems in 2024 - ECMWF Data Distribution Network Spans 65 Countries in 2024
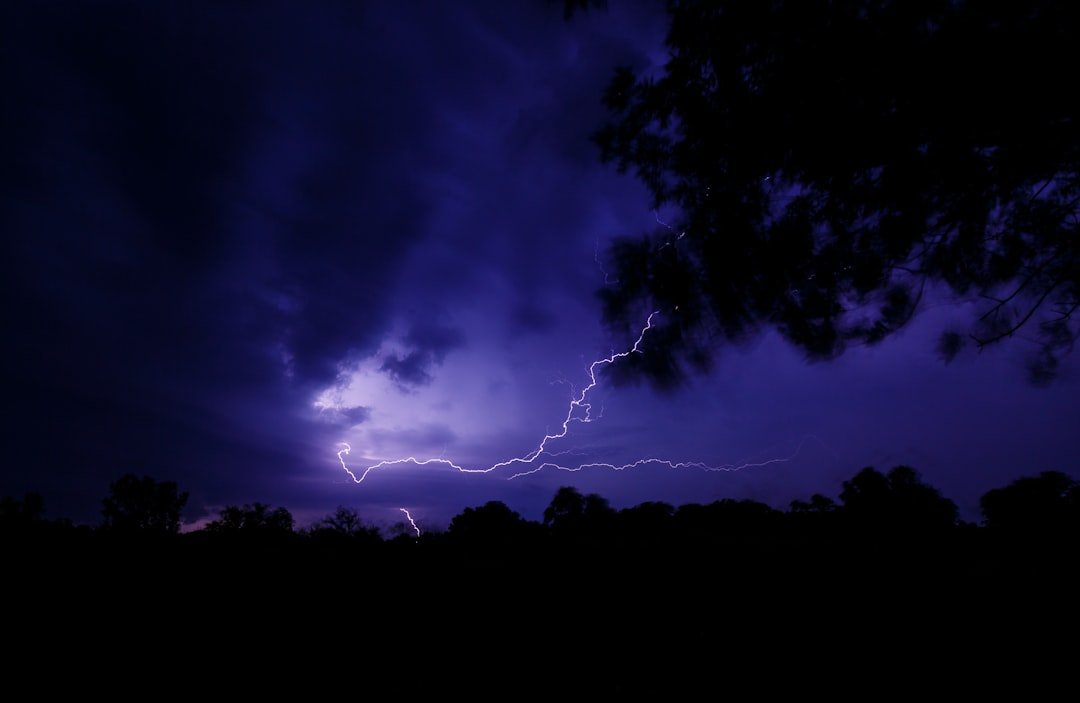
The ECMWF's data distribution network now reaches 65 countries in 2024, highlighting its growing importance in global weather forecasting. This network provides access to a massive repository of weather data, fueling both operational weather predictions and ongoing research. The ECMWF's data archive, MARS, is one of the largest globally, containing over 505 petabytes of weather information. The continuous expansion of this archive, along with advancements in supercomputing, underscores the ECMWF's commitment to enhancing its forecasting capabilities. The increased reliance on distributed computing within weather forecasting, exemplified by the ECMWF's advancements, hints at a possible shift towards more decentralized meteorological services. While promising, the increasing use of machine learning and the scale of data handling present challenges to ensuring the continued accuracy and reliability of future weather predictions. This necessitates a careful consideration of the potential biases and limitations inherent in these approaches.
The ECMWF's data distribution network, active in 2024, connects weather services across 65 countries. This broad reach allows for the sharing and synchronization of meteorological data, which is crucial for improving the accuracy of global weather forecasts. It's quite a feat to coordinate data from so many different sources, and hopefully it improves both forecasting quality and timely data access for everyone in these nations.
This extensive network facilitates the rapid transmission of large datasets, contributing to real-time weather monitoring across diverse regions. I wonder if the varying data rates across countries create any hurdles in managing this flow. One hopes they have taken that into account.
The network's upgrades in 2024 have notably reduced latency in data transfer times. This is critical for generating frequent forecast updates, particularly important when handling sudden shifts in weather conditions. I'd be keen to learn about the actual latency reduction achieved and how it impacted forecast update rates.
The ECMWF network employs a blended infrastructure using both traditional data centers and cloud-based solutions. This hybrid approach aims to enhance both reliability and scalability for handling the massive amounts of weather data being generated. While it likely enhances their resilience, I am curious if managing a mix of older and newer systems causes any operational complexities.
Each participating nation contributes unique local weather data to the network, fostering a collaborative approach to understanding regional weather patterns. This collaborative approach could offer improvements to localized forecasts, but it raises questions about data privacy and potentially who has access to which datasets.
ECMWF's network has expanded in 2024 to incorporate more advanced satellite data integration. This improved integration facilitates more detailed atmospheric profiling, potentially enhancing the prediction of severe weather phenomena like hurricanes and tornadoes. This is a big development but one needs to assess how robust this new data source is in different geographical regions.
This decentralized ECMWF network forms the backbone for developing more sophisticated ensemble forecasting. Meteorologists can now simulate multiple potential weather scenarios with increased efficiency. Having said that, the real question is how well the system performs in producing high-quality ensemble forecasts that account for all the variables involved.
One of the obstacles they face in this vast network is the standardization of data formats and protocols across the participating countries. This can occasionally lead to integration challenges and make it tricky to consistently utilize the data for forecasts. It's one of the challenges inherent in collaborative efforts.
The ECMWF prioritizes interoperability between different computing platforms, demanding improved communication protocols. This ensures that all member states can seamlessly participate in the shared network. While promoting standards is critical, it also comes with the risk of stifling the innovation that might be possible from using more diverse platforms.
The network's success also underscores a broader shift in the field of meteorology. This interconnected global forecasting community has transitioned away from being reliant on a single forecasting source. A welcome shift in many regards, but how effectively this shift can manage forecasting inaccuracies remains to be seen.
How Distributed Computing Powers Modern Weather Forecasting Systems in 2024 - Weather Prediction Models Run on 15000 Processing Cores Simultaneously
Modern weather prediction models are now leveraging the power of distributed computing, running concurrently on as many as 15,000 processing cores. This substantial increase in processing power significantly boosts the capabilities of numerical weather prediction (NWP) models, allowing them to perform intricate calculations necessary for accurate forecasting. These advancements are vital for improving the reliability of forecasts, particularly during severe weather events, where rapid and precise information is crucial for public safety.
The enhanced computational power not only leads to higher resolution models, capturing more detailed atmospheric information, but also facilitates the incorporation of machine learning and other artificial intelligence techniques. These AI approaches help refine prediction accuracy and potentially reduce the time it takes to generate a forecast. However, the considerable computational demands of these complex models raise questions about long-term sustainability and the potential for biases to creep into the forecasts generated by automated systems. As these models become increasingly complex and resource-intensive, concerns about equitable access to such advanced forecasting technologies and their ability to capture the full range of atmospheric complexities become prominent. The field must carefully consider how to balance the benefits of these advancements with potential limitations and ensure their effective use in a rapidly changing climate.
The scale of modern weather prediction is truly remarkable. Utilizing as many as 15,000 processing cores simultaneously, these models handle an immense volume of data—ECMWF alone boasts an archive exceeding 505 petabytes. This massive data flow, encompassing real-time observations from satellites, radars, and ground stations worldwide, enables forecast models to adapt in real-time to changing atmospheric conditions. However, despite this incredible computational power, predicting weather remains a challenge due to the inherent complexity and chaotic nature of the atmosphere itself.
The distributed nature of these systems also brings about benefits in terms of resource allocation. Forecasters can dynamically adjust the processing power based on the urgency of a particular event, like an approaching severe storm. This dynamic resource management helps optimize efficiency and ensures computational resources are efficiently deployed. Furthermore, the ability to run numerous simulations in parallel has become a crucial tool for ensemble forecasting. This approach, where multiple potential weather scenarios are simulated, allows for better risk assessment and aids in understanding the range of probable outcomes.
Reduced latency in data transfer is a significant development within these systems, particularly noticeable with ECMWF's network enhancements in 2024. This capability is crucial for delivering timely forecast updates, especially when rapid changes are observed. However, a challenge that remains is achieving uniform data quality across the vast geographical reach of the forecasting networks. Some regions might lack the technology or consistent data collection standards, which can impact the overall quality and reliability of the forecasts in those areas.
Another interesting issue lies in the way many of these models function. Several, especially machine learning-based ones, tend to emphasize correlations in historical data, rather than directly simulating the physical processes of the atmosphere. This reliance on correlations raises questions about whether they can accurately capture rare but crucial events.
Finally, fostering a seamless flow of data between 65 different countries is no small feat. The need for standardized data formats and communication protocols is a constant challenge, and inconsistencies in data handling can affect forecast reliability. The ongoing refinement of machine learning models is also essential to ensure their accuracy over time. Continuous retraining using the most up-to-date data is critical for maintaining the value of these forecasts and minimizing biases. As weather forecasting relies increasingly on complex distributed systems and AI, the need for ongoing research and algorithm development to improve accuracy and reliability remains paramount.
How Distributed Computing Powers Modern Weather Forecasting Systems in 2024 - Local Grid Computing Networks Track Micro Weather Events at 1km Resolution
Localized weather forecasting is experiencing a significant leap forward with the use of local grid computing networks. These networks can now track micro weather events at a remarkable 1km resolution, a level of detail that was previously unattainable. This finer resolution allows meteorologists to pinpoint and model small-scale weather phenomena like localized thunderstorms or unexpected frost events, which would be missed by traditional weather models using larger grid sizes. These advancements are due to improvements in high-performance computing that allow weather simulations to be customized for specific areas, such as cities or provinces. The increased detail allows for more accurate forecasts at a localized level.
The future of weather forecasting appears to be headed in the direction of higher resolution models. Researchers are actively investigating the feasibility of running global weather and climate simulations at 1km resolution, potentially within the next decade. If successful, this development would revolutionize our understanding of extreme weather events and improve the ability to predict them. However, the move toward a more distributed approach to weather forecasting is not without its challenges. Integrating data from diverse local networks can be problematic, and the reliance on AI and machine learning for processing and interpretation raises concerns about potential biases in forecast outcomes. Addressing these challenges will be crucial to ensure these advanced forecasting tools lead to more reliable and accurate predictions.
Local grid computing networks are proving quite useful for tracking localized weather events at an impressive 1 kilometer resolution. This level of detail allows for extremely specific forecasts, which could be invaluable for situations like flash floods or severe storms, potentially helping to improve public safety. These systems rely on collecting a wealth of atmospheric data from a variety of sources, including ground stations and satellites, which builds up a detailed picture of the atmosphere that traditional methods might miss.
One intriguing aspect is the capability to analyze these networks in real-time. This allows for alerts and warnings to be issued within a short timeframe of detecting hazardous weather conditions, which is crucial for immediate responses during emergencies. By distributing computational workloads across local networks, these systems lessen the load on large, centralized supercomputers, leading to a potentially more efficient and cost-effective use of resources while also boosting processing speed. The modular nature of the networks is another positive, as it allows for easy expansion by simply adding more processing units if the demand for more data increases.
The ability to run a larger number of simulations in parallel allows ensemble forecasting to become more effective at predicting the various possible outcomes of local weather occurrences. This gives decision-makers a better way to assess the potential risks. However, challenges remain in coordinating the local networks across various locations, and inconsistencies in data formats and protocols between regions might make sharing meteorological information seamless. Machine learning algorithms are being actively developed and refined within these networks, which in turn allows for weather prediction models to improve their accuracy based on past weather data.
But there are hurdles. While the speed of computation is faster due to this approach, network latency remains a concern. If the data doesn't flow smoothly, alerts and predictions can be slowed down, potentially delaying crucial information. Another thing to ponder is that the reliance on these decentralized networks raises concerns about the possibility of bias in the input data. Making sure that a diverse range of weather patterns are represented in the training data sets is important to ensure forecasts remain reliable. In summary, the use of local grid computing networks is a promising advancement in localized weather prediction, but some hurdles remain regarding the synchronization and data flow across these networks, the potential for biases, and the impact of latency on real-time updates.
Transform your ideas into professional white papers and business plans in minutes (Get started for free)
More Posts from specswriter.com: