How 7 AI Tools Transform Raw Data into Searchable Digital Content in 2024
How 7 AI Tools Transform Raw Data into Searchable Digital Content in 2024 - Polymer Transforms Excel Data into Interactive Web Dashboards with Zero Code
Polymer presents a way to move beyond static Excel spreadsheets by automatically converting them into dynamic web dashboards. The platform uses AI to guide users, suggesting visualizations and simplifying the process of designing and building these dashboards. You can leverage pre-built templates or craft your own from scratch, and with over 20 visualization types and data connectors, you have a solid toolkit for exploring your data. One notable feature is the ability to keep your dashboard live with real-time updates, ensuring your information is always current. This makes Polymer appealing because it lowers the barrier to creating interactive dashboards, whether you're a data whiz or simply need a better way to share insights from your spreadsheets. While the promise of easily creating these web apps is attractive, the real impact depends on how effectively Polymer's AI can guide users towards genuinely insightful presentations, and whether the resulting dashboards are truly effective tools for exploring and understanding data.
Polymer presents a novel approach to transforming Excel data into interactive web dashboards, achieving this without any coding required. It's intriguing how the platform uses AI to suggest suitable data visualizations, streamlining the process of building insightful dashboards. This automated guidance simplifies data analysis, making it more accessible to a broader range of users.
While users can leverage pre-built templates, the ability to craft custom dashboards is also available. Polymer's smart chart suggestions are particularly noteworthy, potentially leading to better interpretations of data patterns. The tool's impressive array of data connectors and visualization options allows users to explore their data quite comprehensively.
One of the most striking aspects is the real-time updating functionality. With a simple click, dashboards can become dynamic displays reflecting the latest information, enhancing the value of dashboards for dynamic data scenarios. Polymer seems focused on making data easier to access and use, contributing to a shift towards more collaborative and secure data management practices.
The intuitive interface is designed for all skill levels. Regardless of technical proficiency, users—from data experts to novices—can effectively leverage Polymer's features. Polymer's embedded AI capabilities enable easier extraction of insights, encouraging deeper dives into data and exploration of the suggested trends.
The tool's success lies in its effort to simplify complex BI procedures. It effectively removes the challenges typically associated with steep learning curves, making it more approachable than traditional BI platforms. Users have reported that Polymer successfully transforms static spreadsheets into live web apps, enhancing not only the visual aspect but also the interactivity of data. This capability can prove valuable for any field dealing with large data volumes and requiring dynamic visualization. It'll be interesting to see how this type of technology impacts the way data is presented and analyzed in the near future.
How 7 AI Tools Transform Raw Data into Searchable Digital Content in 2024 - Power BI Adds Natural Language Analytics for Raw Data Processing
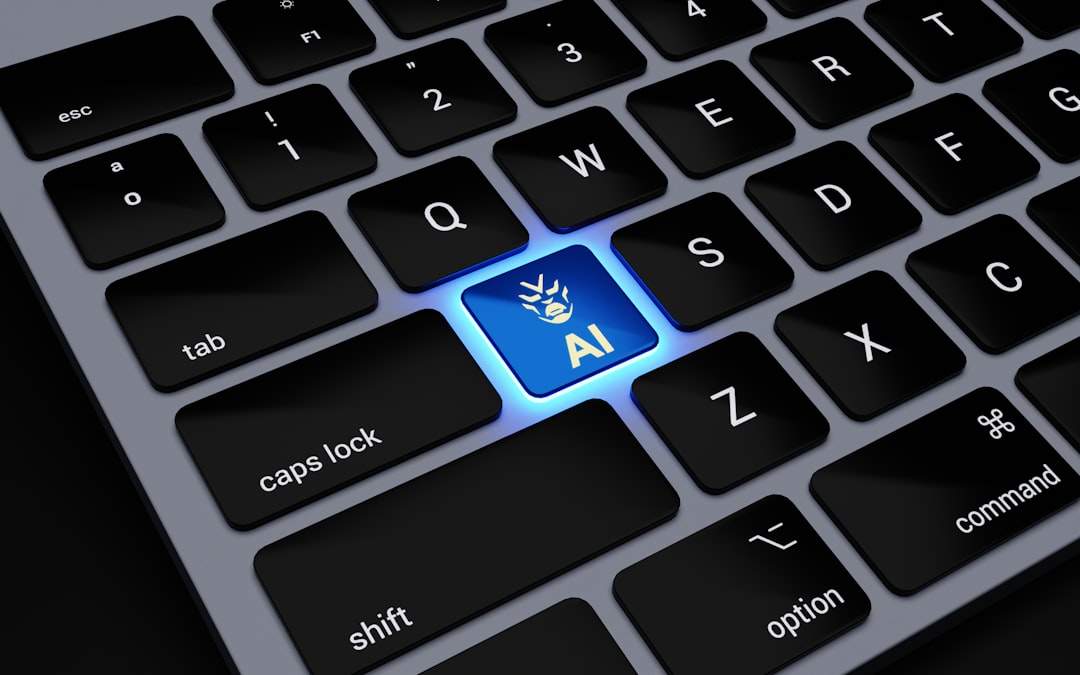
Power BI has added a feature that lets you ask questions about your data using everyday language. You can now type in a question in plain English and get the answer in a visual format like a chart or table. This feature, often called Q&A, aims to make data exploration easier for people without a strong technical background. It essentially bridges the gap between users and complex data sets.
Microsoft has also integrated this natural language capability into Office 365, which could improve the workflow for many users who already rely on those tools. While this approach of using natural language for data analysis is becoming more common in tools like Tableau, the real question is how well Power BI translates your questions into insightful answers. We'll have to see whether this addition truly enhances data understanding or just adds another step in the process. It might prove useful, but it's not yet clear if it represents a genuine advancement in making data accessible or if it's more of a surface-level feature.
Power BI's recent addition of natural language capabilities allows users to explore data by asking questions in plain English, a major step towards making data insights accessible to a broader audience. It's intriguing how it utilizes natural language processing to understand the context and meaning behind these queries, effectively bridging the gap between human language and complex datasets. This lets users interact with the data in a conversational way, rather than needing to learn specialized query languages.
The system's ability to analyze massive datasets in a fraction of a second is remarkable. It's quite impressive how quickly it can translate a question into insightful charts or graphs. You can ask something like "sales in 2023" and the system can often infer what specific metrics you need, even if you haven't explicitly defined them, showing some degree of intelligent interpretation.
One thing that stood out is how Power BI continuously learns from how users ask questions. This suggests that, over time, the quality and relevance of insights will improve as it adapts to individual user preferences. However, it's important to note that if users aren't careful with how they phrase their questions, they may get incorrect or misleading results. It seems you really need to think through what you are asking to maximize accuracy.
Furthermore, it's not limited to simple queries. You can perform more sophisticated analysis by including simple commands in your questions, such as asking it to calculate trends or compare different metrics. This versatility is quite useful for exploring data in greater depth.
It's clear that Power BI is taking part in the wider movement towards "data democratization." The idea is to make data-driven decision making accessible to everyone, not just data specialists. This trend means that people across different departments and skill levels can use data for informed decisions. As these intuitive tools become more common, knowing how to craft good questions will be crucial in extracting the maximum value from data. Ultimately, these types of AI-driven tools could make data analysis more interactive and engaging for everyone.
How 7 AI Tools Transform Raw Data into Searchable Digital Content in 2024 - Jasper AI Converts Unstructured Text into SEO Optimized Content
Jasper AI has become a popular tool for creating content that's optimized for search engines. It takes unstructured text and transforms it into content that's designed to rank well online. This AI-powered tool uses sophisticated machine learning to generate content quickly while also incorporating keyword strategies to improve search engine performance. It's compatible with other SEO tools like SurferSEO, enabling on-the-fly content refinements. Because it supports many languages, Jasper AI is attractive to businesses targeting international audiences. The effectiveness of Jasper AI, like any AI tool, depends on how it's integrated into the wider marketing scheme. While it seems to be a capable content creation tool, it's important to remember that it's only as good as the strategies and tactics employed around it.
Jasper AI employs sophisticated natural language processing techniques to transform unstructured text into a more structured format that's primed for search engines. This process aims to improve the discoverability and relevance of content when people search online. It's like taking a jumbled pile of words and organizing them in a way that search engines can easily understand and present to the right people.
The tool can analyze the intent behind a search query, meaning it goes beyond just using keywords. It tries to figure out the overall meaning and context of the keywords, leading to more relevant content. This contextual awareness is crucial in creating content that truly addresses what people are looking for, which helps maximize the impact of the generated content.
Jasper can connect to different content management systems (CMS). This feature allows for a smoother workflow by publishing SEO-optimized content directly from the platform, reducing the need for manual steps and improving efficiency. How effective it is with the various CMS platforms varies, though, and that would be interesting to investigate further.
Jasper also includes built-in tools for assessing how the generated content is performing in search engine rankings. This lets users make adjustments on the fly to their strategies, which is useful for continuously refining their approaches. While having this feedback loop is helpful, it remains to be seen how effective it is in consistently improving rankings in practice.
One interesting characteristic of Jasper is its ability to adapt to user interactions. Over time, it can learn not only how to generate text better but also what kind of content and writing styles individual users prefer. This personalization element could be helpful, although the long-term implications of systems learning in this manner will need further exploration.
During the content generation process, Jasper analyzes the frequency of keywords to optimize their use. It strives to find a balance between SEO effectiveness and readability, avoiding keyword stuffing—a common pitfall where content is overly saturated with keywords. It's notable how this aspect of the tool tries to prevent issues like keyword stuffing, as this is a crucial element of making content look and feel natural.
From a single piece of input, Jasper can generate a variety of content versions. Users can experiment with different tones, styles, and formats without having to start each time from scratch. This feature is helpful for marketing tasks, particularly in A/B testing. The potential this offers is apparent, although it's unclear how much variation Jasper can realistically provide and if it leads to genuinely meaningful results in A/B testing scenarios.
Jasper also supports creating content in multiple languages, which expands its usefulness for international marketing efforts. However, there are sometimes nuances between cultures and languages that it may not always get quite right. This aspect of its capability will require more refinement to ensure accuracy and cultural sensitivity across a broader range of languages.
Jasper AI can also integrate with predictive analytics, which helps anticipate trends in content consumption. This feature could help content creators to stay aligned with popular topics and audience interests. It's a compelling idea to predict future content preferences and trends, but it's unclear how accurate these predictions are in practice and how frequently they provide genuinely useful insights.
Although Jasper automates the writing process, it's essential for humans to review the content it generates. Relying solely on AI-produced content can sometimes lead to inconsistencies in a brand's voice or style. It's worth noting this caveat because it emphasizes that humans still play a critical role in the creation and evaluation of online content, even when using AI tools. While AI can accelerate certain aspects of the process, careful editing and human judgment remain necessary.
How 7 AI Tools Transform Raw Data into Searchable Digital Content in 2024 - Claude 0 Processes Complex PDF Documents into Machine Readable Text
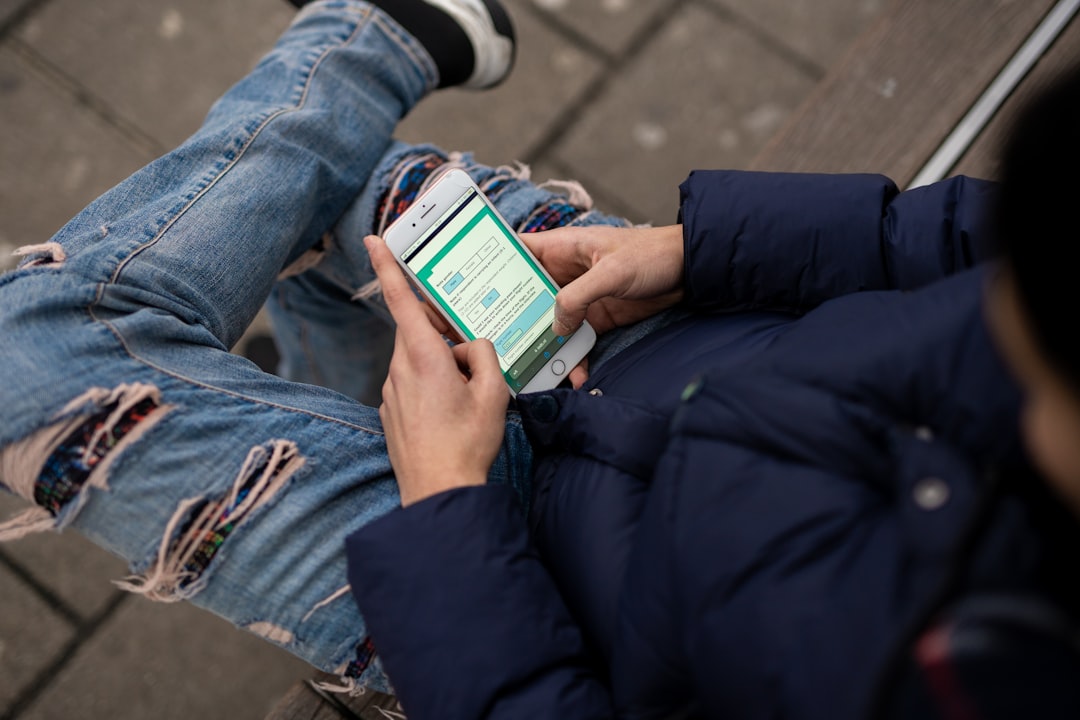
Claude 0, from Anthropic, stands out for its ability to handle intricate PDF documents and transform them into text that computers can readily understand. This AI leverages OCR and natural language processing, essentially unlocking the information trapped within often-unwieldy PDF files. While the quality of OCR has improved, issues with complex formatting or low-quality scans can still hinder its accuracy. Claude 0 goes beyond basic OCR, though, offering features like summarizing lengthy documents and interactive Q&A sessions. This provides a richer and more dynamic way to engage with the content of a document. As AI evolves, tools like Claude 0 are at the forefront of making it easier to access and manage critical information, a development that could have a significant impact on how we deal with digital documents. There's still room for improvement, particularly in addressing the remaining OCR accuracy challenges, but the potential for AI to transform how we interact with documents is quite apparent.
Claude 0, an AI model, is designed to process complex PDF documents, transforming them into a format computers can easily understand. It does this by employing a layered approach that delves deeper than simple text extraction. It attempts to discern not only the text itself but also how the different parts of a PDF relate to each other. This capability is significant because it can potentially extract more meaningful information than a standard optical character recognition (OCR) tool.
While standard OCR focuses on converting images of text into digital text, Claude 0 tries to capture the structure of a PDF. It can differentiate between sections, headings, and subheadings. While this sounds basic, it can greatly improve how humans later interact with the data. For instance, you might find that the output is more organized and easier to understand. The reliability of extracting text from images, however, always depends on the initial quality of the PDF. Low-quality scans or very complex page layouts can present difficulties for any OCR system.
AI-based PDF readers like Claude 0 can do more than just basic OCR. They use machine learning and natural language processing (NLP) techniques. This allows them to potentially go beyond simple text conversion and attempt to summarize lengthy documents. Essentially, Claude 0 tries to get at the meaning or gist of what a PDF is communicating. The success of this depends greatly on how well the algorithms are designed. It's worth mentioning that algorithms are typically trained on a massive amount of data, and this training can introduce biases, which might not be readily apparent in the output.
Claude 0 goes a step further by incorporating features that can automatically extract important information from PDFs. Think of it as extracting specific data points rather than simply providing a copy of the text. The other potentially useful feature it offers is the ability to interact with PDFs through a chat-like interface. You can ask questions about the content of the PDF. The accuracy of this approach remains a concern. The system must accurately interpret the question and extract relevant answers from the text.
NLP capabilities, like those used by Claude 0, are constantly evolving. The models are designed to learn and adapt from experience. As more users employ the tool, the model should improve. The challenge is how to ensure that this continuous learning process is well-managed and does not accidentally develop unwanted biases in the interpretation of PDFs.
One of the strengths of Claude 0 is its ability to handle various languages. It's not just confined to English. This aspect makes it suitable for international datasets and research where PDFs may be created in a variety of languages or contain mixed language text. It's notable that accurately handling non-Latin based languages like Chinese or Arabic within PDF formats remains a challenge. There may be characters or formatting issues that the system may not always handle perfectly.
Claude 0 is well-suited for handling complex types of documents like legal contracts or scientific papers. These documents are often dense with information and have precise formatting. There are times when maintaining this precise formatting during the conversion process is essential. Successfully interpreting and converting such documents depends on a combination of both sophisticated natural language processing techniques and a deep understanding of document structure.
Since Claude 0 is not an isolated tool, it's designed to interface with other systems. This ability to fit within larger frameworks is critical for organizations that need to process PDFs as part of a larger data flow. It can easily export data in multiple formats, which provides flexibility to users in different work environments.
An essential element of Claude 0 is its ability to check for errors in the extracted text. It's designed to potentially spot inconsistencies and flag anomalies. This feature is vital for maintaining data integrity, which is crucial for applications where PDFs contain sensitive or critical information.
Users have the flexibility to configure how they want Claude 0 to extract data. This level of customization allows for more tailored outputs. This is helpful for various industries that require specialized data extraction. The effectiveness of the tailoring depends on the user's ability to appropriately set these parameters. It requires some understanding of how the tool functions to configure it properly.
Claude 0 offers a promising approach to analyzing PDFs, but its true efficacy remains to be fully tested. We'll need to see how it performs with a wider variety of PDF formats and data structures to see if it truly delivers on its intended promise. The continued development of these tools is likely to be an area of focus for researchers in the near future.
How 7 AI Tools Transform Raw Data into Searchable Digital Content in 2024 - OpenAI's Whisper Converts Audio Files into Searchable Transcripts
OpenAI's Whisper is an AI model designed to transform audio files into searchable text transcripts, making audio data more accessible across a range of uses. Trained on a wide variety of audio, it can transcribe different file formats like MP3 and WAV without requiring specific adjustments for each type of file. Its capabilities extend beyond basic transcription, including the ability to translate audio from multiple languages into English. Whisper also excels at accurately including punctuation and grammar in transcripts, making it useful for tasks like creating written content from podcasts or YouTube videos. The model also handles real-time transcription, which is helpful in generating text from live audio events. In addition, it can be used to analyze sentiments within the transcribed audio and pull out important keywords. Whisper has garnered considerable attention, with over two million uses reported. This signifies its reliability for transcribing audio across various fields such as media production, education, and law. However, it is crucial to continually assess its accuracy, especially in complicated audio scenarios, as it continues to improve and develop.
OpenAI's Whisper is an AI model that's quite impressive in its ability to take audio files and transform them into searchable text transcripts. It was trained on a huge and diverse range of audio data, which makes it remarkably versatile, capable of handling different accents, dialects, and even noisy recordings without needing to be specifically tuned for each situation.
Whisper can handle a variety of audio formats, including common ones like MP3, WAV, and FLAC. It's worth noting that while it's designed for a broad range of tasks, for purely English language work, specialized models like "tinyen" and "baseen" often perform slightly better. It also has a neat trick—you can give it a command to translate audio in different languages directly to English.
Whisper is designed to produce transcripts that are more than just text – they are easily searchable. This is a great tool for industries like media, education, and law where navigating large collections of audio or video is essential. Not only that, it shows surprising accuracy when it comes to things like punctuation and grammar, which is helpful for folks who work with things like podcasts or YouTube videos and need cleaner, more polished transcripts. And it's not just good for pre-recorded material. It can handle real-time transcription, allowing for live audio capture and conversion to text.
It's not just about transcription, either. It can be used for tasks like trying to discern the general feeling or tone of what's being said (sentiment analysis), and extracting important words or phrases (keyword extraction) from the transcripts. It's gaining popularity – they've clocked over two million runs of the model, which shows its reliability and utility. While still being refined, it’s shown considerable promise. While it's not perfect, it holds much promise for making audio data easier to work with in different fields, demonstrating that AI is becoming increasingly useful in converting complex data into more readily accessible formats.
How 7 AI Tools Transform Raw Data into Searchable Digital Content in 2024 - Neo4j AuraDB Creates Knowledge Graphs from Scattered Databases
Neo4j AuraDB is tackling a common issue: the fragmentation of data across various databases and unstructured formats. It offers a managed graph database solution that allows users to construct knowledge graphs, which are essentially visual representations of interconnected data points. These graphs effectively capture complex relationships hidden within the data, handling massive datasets with billions of nodes and connections. This flexibility caters to diverse needs, ranging from small projects to large enterprise-level applications. Moreover, its ability to integrate with other AI tools streamlines the process of turning raw data into accessible, searchable digital content. This combination is gaining traction as businesses are increasingly seeking ways to easily extract insights from their many data sources. Given the rise in complex data environments, Neo4j's graph-based approach seems poised to play a significant role in helping organizations make sense of their information and utilize it more efficiently. However, like any relatively new technology, there are still questions regarding its long-term impact and whether it can consistently deliver the promised benefits in various scenarios.
Neo4j AuraDB is a managed graph database that has become interesting because of its ability to create knowledge graphs from a wide variety of data sources. This is in contrast to traditional database systems that store data in tables. These knowledge graphs emphasize how things are related, which is really powerful when trying to explore complex topics and ask complicated questions. One notable feature is the speed at which these graphs can be created. This is a significant improvement over the older, more manual processes.
The way that Neo4j works is a bit different. It’s designed to work with relationships between different things, which helps uncover connections that might not be obvious in table-based data. You can ask questions using natural language, which makes it easier for folks who aren't experts in database management to explore the information. They also use a query language called Cypher, which is quite intuitive and allows you to simply say what you want rather than focus on how to get the data, which can speed up research.
This platform also integrates nicely with machine learning. That means you can apply a variety of machine learning techniques directly to the data within the knowledge graph, making it possible to perform more sophisticated analysis like predictions, which can be incredibly valuable for a variety of projects.
One of the big challenges with conventional database systems is scaling as you add more data. Neo4j, though, is built from the ground up to handle these types of challenges effectively. The architecture allows for large amounts of data to be efficiently managed without experiencing performance slowdowns.
Furthermore, this system is very adaptable. The database doesn't require a rigidly defined structure up front. This means that you can adjust and expand the graph as you learn more about your data, which is important for projects that evolve over time. It also has great tools for visualizing the graph data. This can be important for exploring the connections between different parts of the data, and also helps with communication to others when explaining complex patterns.
Neo4j has the ability to draw data from various sources and build a knowledge graph, which can be extremely useful in situations where data might be stored in multiple systems. Bringing these diverse data sources together into a single knowledge graph can reveal connections and insights that were previously not evident. Also, the strong community and extensive resources provided by Neo4j make it easier for users to learn about how to make the most of the platform and help with solving various challenges that come up during projects. It’s a collaborative environment that promotes development.
It’s an interesting technology to keep an eye on, especially as we see how it performs in increasingly complex applications. As with any technology that leverages AI, it’s important to consider its limitations and potential biases. Still, the ability to quickly create complex knowledge graphs from scattered data using intuitive tools is a capability that has the potential to accelerate discovery and insight generation across various disciplines.
How 7 AI Tools Transform Raw Data into Searchable Digital Content in 2024 - Azure Cognitive Search Builds Custom Search Engines from Corporate Documents
Azure Cognitive Search (formerly known as Azure AI Search) allows you to create custom search engines specifically designed to handle the wealth of documents found within a company. It uses clever techniques like identifying key pieces of information (entities) and integrating with advanced language models (through Retrieval-Augmented Generation or RAG). This enables organizations to organize and access the large portion of their data currently trapped in formats like PDFs and Word documents. The system offers tools to tailor how search results are presented, giving users more control over the relevance of what's found.
While Azure Cognitive Search has the potential to significantly improve how companies search through their own data, its success relies on how well the data is structured and prepared for the system. Accuracy when dealing with language can still be a problem, a common issue for many AI tools in this area. Despite these limitations, Azure Cognitive Search represents a notable step forward in making it easier to locate and utilize corporate knowledge, a vital aspect of operating in the current data-focused environment.
Azure Cognitive Search, previously known as Azure AI Search, is a cloud-based service that aims to make searching within large collections of corporate documents more efficient and insightful. It's designed to work across various platforms, including web and mobile applications. It's specifically optimized to work with Retrieval-Augmented Generation (RAG) approaches that integrate directly with Azure's OpenAI service. One interesting feature is its 'Custom Entity Lookup' skill, which lets you identify and categorize specific things within documents (like names, dates, or product numbers) based on custom lists you create.
It relies on something called an indexer to connect with different data sources. A common scenario is pulling documents from Azure Blob storage. The indexer then processes the content, making it ready for searching. This tool offers extensive control over the search process, allowing for fine-tuning ranking and scoring methods, and including advanced linguistic analysis. It seems this tool is geared towards handling the majority of business data, which is often stuck in formats like PDFs and Word documents, by using AI to essentially make those documents more readily searchable.
Azure Cognitive Search is responsible for handling both the initial intake of content and the management of subsequent search requests. It creates a searchable index of your data, essentially making it easier to find what you need within a vast repository. It offers developers a set of tools (like APIs) to create specialized search experiences tailored to specific needs and data. One of its noteworthy capabilities is semantic search, an approach aimed at improving search accuracy by understanding the intent behind a user's query, moving beyond simple keyword matching.
Azure AI Search is positioned as a solution for handling search within complex and diverse data within an organization. It's adaptable, allowing for customization to meet various needs. While it seems promising, the true success of such a system depends on its ability to effectively handle the intricate nuances of unstructured data, which can sometimes be a challenge. As it's part of the larger Azure ecosystem, the potential for it to interact with other tools and data sources adds to its potential. However, it's unclear if it can genuinely improve information retrieval in a significant manner, or if it's just another complex tool that needs to be mastered.
More Posts from specswriter.com: