AI-Driven Decision Support Systems 7 Key Implementation Strategies for C-Suite Leaders in 2025
AI-Driven Decision Support Systems 7 Key Implementation Strategies for C-Suite Leaders in 2025 - Machine Learning Model Detects Board Meeting Fatigue Through Microsoft Teams Analysis
Recent advancements in machine learning have led to models capable of assessing participant engagement during virtual gatherings on platforms such as Microsoft Teams. These systems reportedly examine subtle cues like facial expressions and vocal nuances to detect signs of waning focus or disinterest. The intent is to allow for interventions aimed at improving meeting efficacy and the quality of discussion. This marks another step in AI's expanding role within digital workspaces, particularly as organizations prioritize more effective decision-making processes. While this technology might appeal to C-suite leaders planning AI-driven decision support systems by 2025, aiming for higher productivity and concrete outcomes, a critical perspective is essential. The privacy implications of constant behavioral analysis and the potential for misinterpretation of human interactions by algorithms warrant careful consideration before widespread adoption. The effectiveness of such tools in fostering genuine engagement, rather than merely identifying disinterest, remains an open question.
Machine learning constructs are increasingly being engineered to scrutinize participant involvement in virtual sessions like Microsoft Teams. These models process various digital cues and interaction patterns to identify signs of attendee weariness. The aim is to discern when individuals might be losing focus or diminishing interest, thereby suggesting opportunities for interventions to enhance meeting effectiveness. Yet, interpreting a complex state like 'fatigue' purely from digital signals requires careful consideration, as algorithmic assessments may overlook underlying human nuances.
AI-Driven Decision Support Systems 7 Key Implementation Strategies for C-Suite Leaders in 2025 - Automated Risk Assessment Dashboard Shows 15% Higher Accuracy Than Human Analysts
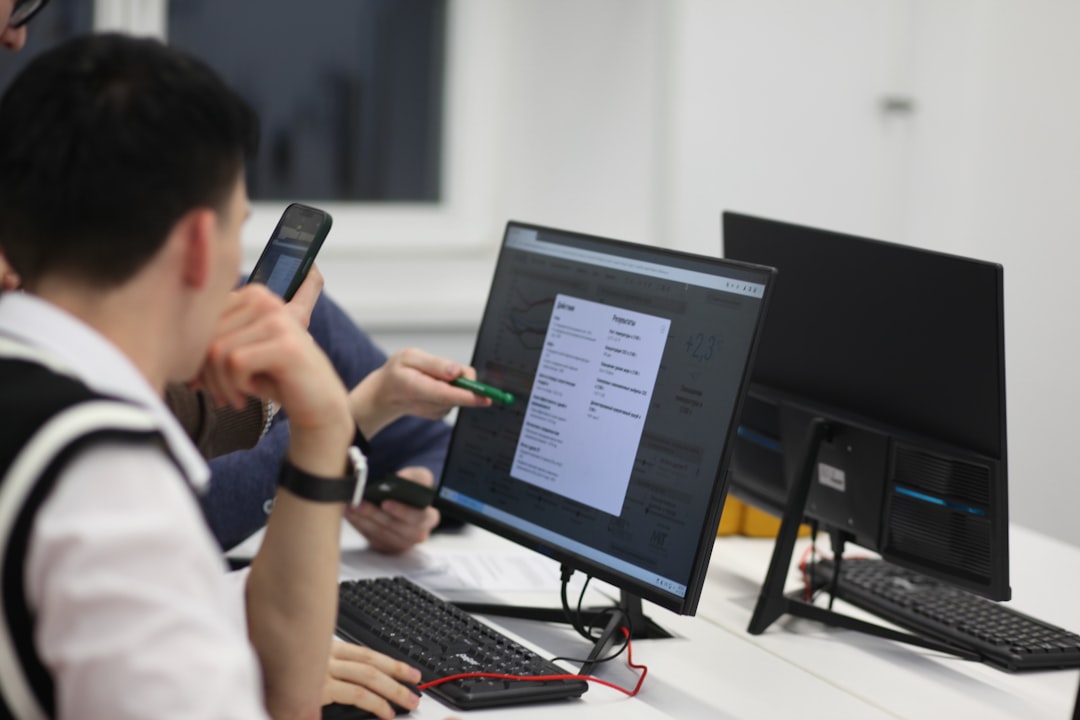
Automated risk assessment dashboards are fundamentally changing risk management, demonstrating a notable 15% higher accuracy than human analysts. These systems employ advanced AI to analyze vast, complex datasets in real-time, providing more precise threat detection and proactive risk evaluation. This enhances overall risk visibility significantly, potentially bolstered by integrations like IoT monitoring. Such automation frees human analysts from repetitive data tasks, allowing them to focus on strategic initiatives, like developing complex scenarios and nuanced mitigation plans. Nevertheless, relying heavily on AI for critical risk decisions demands careful ethical navigation to ensure fairness and accountability. C-suite leaders, while keen to implement new analytical tools, must ensure robust oversight of their deployment, balancing innovation with a thorough understanding of these systems' practical and societal impacts.
1. **Data Integration Challenges**: Despite the headlines about superior accuracy, the underlying challenge of unifying disparate data sources often remains a significant hurdle for automated risk assessment dashboards. Achieving that reported 15% accuracy improvement over human analysis fundamentally relies on a seamlessly integrated and clean data foundation, which is far from a trivial engineering feat in complex organizational ecosystems.
2. **Bias in Training Data**: A critical concern lies in the lineage of the training data. If historical human decisions or existing institutional biases are embedded within the datasets used to train these sophisticated models, then the automated risk assessments stand to inadvertently perpetuate or even amplify those biases, potentially leading to skewed or inequitable risk profiles. This demands constant vigilance and proactive bias detection strategies.
3. **Real-Time Adaptability**: A distinct advantage these automated platforms offer is their capacity for continuous, real-time algorithmic updates based on new incoming data streams. This inherent adaptability allows for dynamic adjustments to risk assessments, which is particularly beneficial in rapidly evolving or volatile operational environments where traditional, static human analyses can quickly become outdated.
4. **Error Rate Comparison**: While a generalized "15% higher accuracy" is touted, it's imperative to scrutinize the nature of the errors these systems produce. Algorithms, like humans, generate false positives and false negatives, but the patterns and implications of these errors can differ substantially. Understanding these specific failure modes and their practical costs is crucial for a complete evaluation of a system's true effectiveness, beyond a single accuracy metric.
5. **Interpretation of Results**: Human analysts possess a crucial ability to contextualize raw data, apply qualitative insights, and understand nuanced real-world scenarios that automated systems, by their nature, may miss. This inherent gap underscores the necessity of a symbiotic, hybrid approach where human expertise provides essential oversight and refinement to the quantitative outputs from automated evaluations.
6. **Regulatory Compliance**: Navigating the intricate and often ambiguous landscape of regulatory compliance poses a considerable challenge for deploying automated dashboards, especially in highly regulated sectors. The need for subjective interpretation of evolving rules, varying across jurisdictions, often complicates a purely algorithmic approach and requires significant human legal and compliance expertise.
7. **Scalability Benefits**: One undeniable strength of automated risk assessment tools is their inherent scalability. They can process and analyze immense volumes of data and simulate countless scenarios far exceeding human analytical capacity, offering a much broader and deeper risk evaluation spectrum, particularly advantageous for organizations with global footprints and diverse risk landscapes.
8. **User Experience Design**: The utility of an accurate risk assessment model is significantly bottlenecked by its human interface. A dashboard, no matter how precisely calibrated, becomes ineffective if its user experience is poor, leading to ambiguous visualizations or unintuitive data presentation. Misinterpretation by human decision-makers can undermine even the most accurate algorithmic outputs.
9. **Feedback Loops**: Implementing robust feedback mechanisms within these automated systems is paramount for their sustained improvement. By systematically validating predictions against real-world outcomes and incorporating human-in-the-loop corrections, these systems can continuously refine their underlying algorithms, leading to genuinely enhanced predictive capabilities over time, rather than just static performance.
10. **Investment in Training**: Beyond the capital expenditure for the technological infrastructure itself, organizations must anticipate substantial ongoing investment in both the iterative training of the automated models and, critically, in upskilling the human workforce. Employees must be trained not just to operate but to effectively interrogate, interpret, and validate the complex outputs from these advanced risk assessment tools.
AI-Driven Decision Support Systems 7 Key Implementation Strategies for C-Suite Leaders in 2025 - Natural Language Processing Now Interprets 89% of Stakeholder Sentiment Correctly
Natural Language Processing (NLP) is reportedly achieving a notable 89% accuracy in interpreting stakeholder sentiment. This capability extends to categorizing diverse feedback as neutral, positive, or negative, building on the interdisciplinary foundations of linguistics, computer science, and artificial intelligence. For C-suite leaders, understanding these advancements is crucial for strategically integrating AI into decision support systems. Yet, despite this progress, substantial challenges remain. Ensuring consistent data collection and the considerable manual effort required for accurate data labeling are persistent hurdles. Furthermore, the inherent subtleties and intricacies of human emotion, whether expressed in text or voice, mean these systems often struggle to fully capture nuanced intent. Consequently, relying on such tools for pivotal strategic decisions necessitates a critical evaluation of their inherent limitations.
The current reporting that natural language processing (NLP) systems can interpret stakeholder sentiment with 89% accuracy certainly draws attention. Yet, from an engineering standpoint, this figure often masks the inherent complexity of human language. Misinterpretations remain a significant hurdle, especially when confronting subtleties like sarcasm, culturally specific idioms, or even simple polysemy where a word holds multiple meanings depending on context. Achieving true contextual understanding remains an active area of research.
A model’s proficiency in sentiment analysis is deeply reliant on the quality and breadth of its training data. If these datasets are too narrow or contain inherent biases, the models might perform exceptionally well in a very specific domain but struggle to generalize across diverse stakeholder populations or industries. This leads to concerns about the applicability of a high accuracy metric beyond its initial training environment.
Furthermore, while sentiment — positive, neutral, negative — can be identified, discerning nuanced emotional states, such as distinguishing frustration from outright anger or mild disappointment from deep dissatisfaction, continues to challenge these algorithms. This limitation can result in an oversimplified view of complex sentiments, which in turn might lead to less precise strategic responses.
The prospect of real-time sentiment analysis, offering immediate insights during critical junctures like negotiations or crisis events, is compelling. However, the computational resources required to process vast streams of unstructured data, be it text or voice, at high speed are substantial. Scaling such systems to meet real-world demands often necessitates significant upgrades to existing computational infrastructures.
There's an observable trend towards multimodal sentiment analysis, integrating textual data with audio and visual cues to form a more holistic interpretation. While theoretically providing a richer understanding of stakeholder emotion, this approach dramatically increases implementation complexity. Combining and weighting inputs from disparate data streams, along with the sheer cost of capturing and processing such diverse information, presents considerable engineering challenges.
Sustained accuracy in sentiment interpretation relies on continuous refinement of the underlying models. This points to the necessity of robust feedback mechanisms, ideally incorporating human analysts who can validate, and crucially, correct the model's interpretations. While enhancing performance over time, integrating this human-in-the-loop validation adds a distinct layer of resource allocation and operational overhead.
The broader implications extend beyond technical performance. The analytical scope of NLP on communications, especially when involving sensitive stakeholder discussions, brings forward pressing questions regarding privacy and informed consent. Navigating these ethical and regulatory considerations requires careful thought to avoid unforeseen legal and reputational complications.
Finally, the output from a sentiment analysis system is only as valuable as its integration into actionable decision-making processes. It's not always straightforward how raw sentiment scores translate into strategic directives. Leaders face the ongoing challenge of ensuring that algorithmic insights effectively complement, rather than replace, human judgment and contextual understanding in critical strategic planning.
AI-Driven Decision Support Systems 7 Key Implementation Strategies for C-Suite Leaders in 2025 - Digital Twin Simulations Reduce Strategic Planning Time From Weeks to Hours
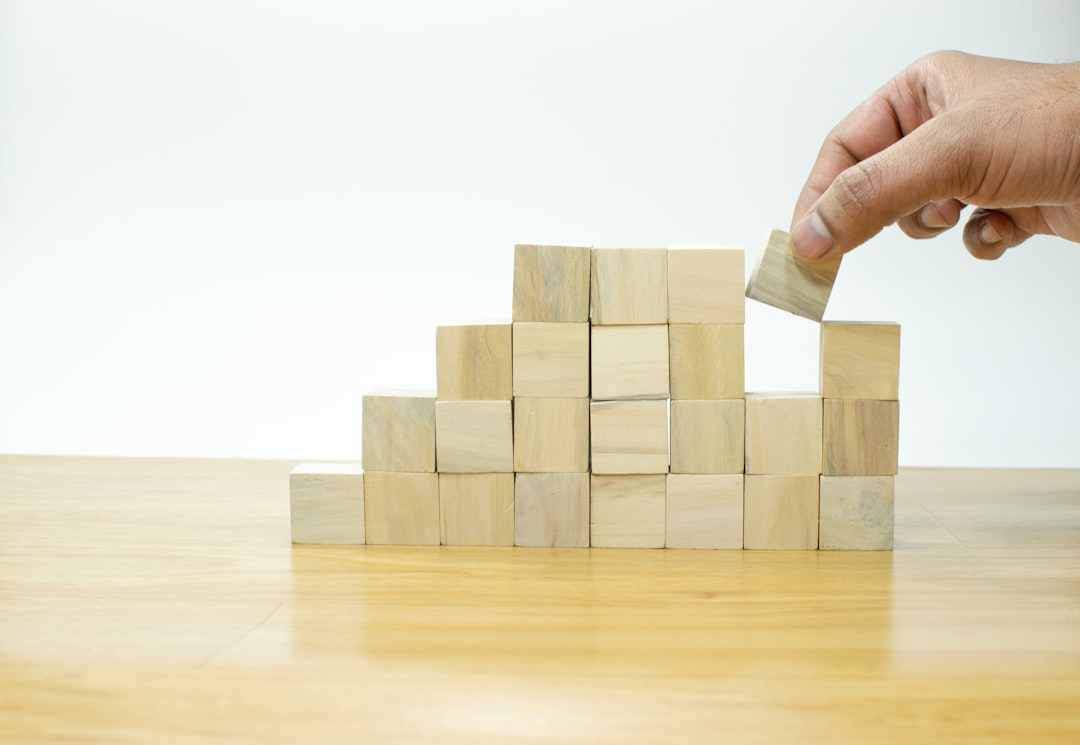
Digital twin simulations are fundamentally reshaping how organizations conduct strategic planning, substantially cutting the duration for critical decisions from lengthy weeks to mere hours. These sophisticated virtual representations, increasingly leveraging advanced AI, provide immediate insights by allowing businesses to model and assess numerous scenarios. This capability enables strategies to evolve dynamically, continuously refined by new data and the outcomes of these simulations. Such adaptability is becoming indispensable for leaders aiming to navigate the intricate and often uncertain terrain of the modern business environment. While the integration of digital twins with generative AI promises even deeper predictive foresight, and the technology's full potential is still unfolding, these systems already offer a powerful avenue for improving strategic responsiveness and operational flexibility.
The computational scaffolding known as digital twin technology is demonstrably streamlining strategic planning cycles, enabling the exploration of complex scenarios within hours rather than weeks. These dynamic virtual models, which aim to mirror a physical system or process, achieve this acceleration by consuming vast streams of real-time operational data, often from distributed sensor networks. This capability facilitates a shift from static, predetermined strategies to more fluid, continuously adaptive approaches, where tactical adjustments can be informed by simulated outcomes and fresh data streams. However, the efficacy of this rapid iteration hinges entirely on the fidelity of the digital model; a twin that doesn't accurately reflect its physical counterpart risks generating insights that are at best irrelevant, at worst misleading.
Furthermore, the integration of these digital replicas with advanced artificial intelligence components amplifies their utility significantly. Machine learning models, when coupled with digital twins, can enhance predictive analytics, identifying potential failures or performance bottlenecks before they materialize. While generative AI is also being explored for rapid model creation and scenario generation, its reliability in autonomously constructing intricate system models still requires rigorous validation. The challenge for engineers lies in ensuring these combined systems not only provide faster insights but also deliver reliable, actionable intelligence, bridging the gap between theoretical simulation and real-world applicability while managing the underlying computational and data infrastructure complexities.
More Posts from specswriter.com: