7 Critical Changes in IT Operations Management Expected for 2025 From AIOps Integration to Zero-Trust Implementation
7 Critical Changes in IT Operations Management Expected for 2025 From AIOps Integration to Zero-Trust Implementation - Machine Learning Driven Alert Management Reduces False Positives by 40%
Intelligent systems using machine learning are transforming how IT teams deal with security alerts. By analyzing the flood of alerts, these systems can significantly reduce the number of false alarms, with some seeing a 40% decrease. This translates into a clearer view of real threats and allows IT teams to concentrate on genuine problems instead of being swamped by a sea of irrelevant noise.
As organizations become more complex and reliant on technology, employing AI-powered systems not only improves efficiency but also fits in with the bigger picture of changes expected in IT operations by 2025. We're talking about integrating AI into IT operations (AIOps) and stricter security models like zero-trust. The improvements in alert management are a good example of the broader changes occurring in IT management. We see a shift towards faster responses and improved security, but challenges exist in implementing these systems completely. The journey towards effective alert management represents a wider change within IT operations, where teams are trying to find the balance between reactivity and strong security measures.
It's fascinating how machine learning is being used to refine alert management. By examining historical data, these systems learn to differentiate between typical network behavior and genuine issues. Essentially, they build up a picture of what's "normal" and flag deviations as potential problems. This approach has shown promising results, with some organizations reporting a remarkable 40% decrease in false positives.
What's even more intriguing is the knock-on effect this has. Improved accuracy translates to a more efficient operational response. Teams spend less time sifting through irrelevant alerts and can focus on genuine issues, leading to faster resolution times and, reportedly, higher staff morale. It's logical: when you're not constantly bombarded with noise, you're more attentive and productive.
Moreover, the adaptability of these systems is remarkable. They don't just rely on past data; they leverage reinforcement learning to adapt to new threats and patterns as they emerge. This is crucial because the threat landscape is constantly changing.
We're also seeing a growing trend towards incorporating user behavior analytics alongside traditional monitoring. This more holistic approach further helps discern between unusual but benign activities and genuinely concerning anomalies, further refining the alert process. This is just one example of how machine learning is poised to transform IT operations management, allowing us to handle incidents more effectively and prevent disruptions. While the technology is still maturing, it’s clear that the impact on improving security response and operational efficiency is significant.
7 Critical Changes in IT Operations Management Expected for 2025 From AIOps Integration to Zero-Trust Implementation - Network Operations Teams Switch to Automated Root Cause Analysis
Network operations teams are increasingly dealing with complex IT environments, making automated root cause analysis a vital part of their approach. The use of AIOps is helping organizations better predict incidents and speed up their resolution. Automated insights, driven by AI, are not just shortening the time it takes to fix problems (MTTR), but are also improving collaboration across different teams, a big help in speeding up the development process (DevOps). This shift represents a move away from reacting to problems and towards anticipating them. It lets teams focus on fixing the real issues instead of getting lost in the manual work of figuring out what's wrong. Integrating AIOps into how network operations are managed is likely to fundamentally change how teams address technical problems, as they strive to be more efficient and flexible. While this automated approach to troubleshooting is proving useful, successfully implementing AIOps and reaping the full benefits will be a challenge and require careful planning and execution.
It's becoming increasingly common for network operations teams to rely on automated systems for figuring out the root cause of problems. These systems, often powered by AI (AIOps), promise a significant speed-up in problem resolution, potentially shaving off up to 70% of the time it takes to fix things. This quicker resolution is crucial for preventing disruptions to users and services.
One of the biggest challenges in modern IT is the sheer complexity of these environments. We're dealing with a mix of on-premises and cloud systems, making it difficult to pinpoint where exactly a problem is coming from. Automated systems excel here, taking in data from various sources like logs, network performance data, and application monitoring. This holistic view makes identifying the root cause more accurate than the old manual methods.
Interestingly, some of these automated tools use anomaly detection. They essentially build a profile of what "normal" network behavior looks like and then flag anything that deviates from that as a potential issue. It's a more proactive approach, helping teams anticipate issues rather than just reacting to them after they've already occurred.
The economic benefits are also appealing. Organizations that have adopted these systems have reported savings of up to 30% in costs related to incident management. This is largely because automated analysis reduces the need for manual troubleshooting and gets things back online faster. Less downtime equates to better efficiency and happier customers.
These automated systems are also designed to scale with the changing nature of IT. As organizations adopt new technologies or reconfigure their networks, automated RCA tools can seamlessly adapt. In contrast, traditional troubleshooting methods often struggle to keep up with this rapid evolution of technology.
By automating the root cause analysis, it becomes less dependent on human decisions, which, in turn, can decrease the number of errors due to oversight or fatigue. We've all been there – working long hours, analyzing complex systems, and possibly missing something obvious due to exhaustion. Automating the process removes that risk, leading to more precise and consistent diagnosis.
All this frees up the network operations teams to focus on more strategic work. They're less bogged down with constant firefighting and can instead use their skills to develop new solutions, improve system performance, and enhance the entire operational landscape. These automated systems are also able to provide real-time insights, giving teams a clear picture of the network health at any given time. This allows them to stay one step ahead of potential issues, ensuring the continuous delivery of services.
It's not surprising that the adoption of these automated tools is on the rise. By the end of 2024, more than half of large organizations were already implementing some form of automated root cause analysis. This trend suggests a significant shift in how IT operations teams are addressing problems – moving away from the reactive, manual approaches of the past and embracing a more proactive, data-driven future. It's fascinating to see this transition unfold.
7 Critical Changes in IT Operations Management Expected for 2025 From AIOps Integration to Zero-Trust Implementation - Edge Computing Integration Creates New IT Operations Standards
The rise of edge computing is bringing about a fundamental shift in how IT operations are managed. By processing data closer to where it's generated, edge computing enables faster decision-making, a crucial advantage for industries needing real-time insights, like manufacturing, healthcare, and retail. This decentralized approach to computing offers solutions to the latency and bandwidth limitations often associated with cloud-based systems, boosting an organization's ability to quickly adapt and react to changes.
However, edge computing's integration isn't without its own set of complexities. It necessitates the creation of new security protocols and requires careful integration with AI and IoT technologies to truly maximize its efficiency. We're essentially seeing a new paradigm for IT operations where central processing power is balanced with localized computing resources. This creates opportunities for streamlined operations and innovative business strategies, but the transition demands careful planning and implementation to ensure a smooth integration. The evolving nature of edge computing will likely lead to a rethinking of many current standards within IT, paving the way for new approaches to problem-solving and resource management.
The increasing use of edge computing is fundamentally changing how IT operations are managed. We're seeing a massive shift towards processing data closer to where it's generated, which leads to faster response times and a more responsive IT infrastructure. This is particularly important in industries like manufacturing, healthcare, and retail where real-time insights are crucial. By 2025, a large chunk of enterprise data will likely be processed at the edge, requiring a complete overhaul of traditional IT management strategies.
One of the biggest impacts is the need for speedier decision-making. Edge computing enables response times under a millisecond for some applications, which is a significant improvement over the latency we experience with centralized computing. This speed advantage is driven by processing data locally, reducing the need to send information back and forth to a central location. It's interesting to see how this shift affects business agility.
This localized data processing also has economic implications. Moving processing to the edge reduces the amount of data that needs to be transferred to centralized cloud resources, leading to substantial cost savings on bandwidth. We're talking about potential reductions of up to 50% in some cases, which is a major incentive for organizations looking to cut costs. However, this increase in the number of edge devices necessitates a heightened focus on security. We're likely to see an increase in security concerns as the perimeter of an IT environment becomes less defined.
Another major development is how edge computing influences the Internet of Things (IoT). With edge computing, the number of IoT devices in operation is expected to dramatically increase. This, in turn, requires IT teams to develop more robust management tools and protocols for the influx of data and device interactions. Managing such a large-scale, decentralized network of devices and the related data poses some serious challenges.
On the other hand, it's important to keep in mind that the increased usage of edge devices will increase the need for specialized security measures. Edge devices are often located in less secure environments and pose more risks compared to a traditional, well-protected data center. This increase in security risks makes it a high priority for IT teams to develop and integrate new, updated security protocols into their IT infrastructure.
As organizations shift to edge computing, they'll need to develop a unified management framework that integrates both traditional IT operations and the new edge infrastructure. We're seeing a trend toward creating integrated architectures that can streamline operations. These new, hybrid IT environments will require a significant rethinking of IT operational strategies and require IT teams to possess specialized skill sets to deal with this new reality. We're likely to see a significant increase in retraining efforts for IT professionals to deal with the changing demands of edge computing.
We can anticipate that AI will play a more central role in the management of edge computing. This necessitates an evolution of IT operations management and monitoring frameworks. There's also the evolving role of hybrid cloud, with edge computing setups increasingly relying on this architecture, leading to more complex IT environments that need more sophisticated management.
The overall trend points towards a decentralized computing environment, with organizations balancing centralized control and decentralized processing power. It's a significant shift in the way computing environments are being designed and managed, requiring new approaches to IT operations. It will be interesting to see how these trends evolve in the coming years and the challenges IT professionals face as they work to manage increasingly complex and distributed IT infrastructures.
7 Critical Changes in IT Operations Management Expected for 2025 From AIOps Integration to Zero-Trust Implementation - Zero Trust Security Framework Becomes Standard Practice in Enterprise IT
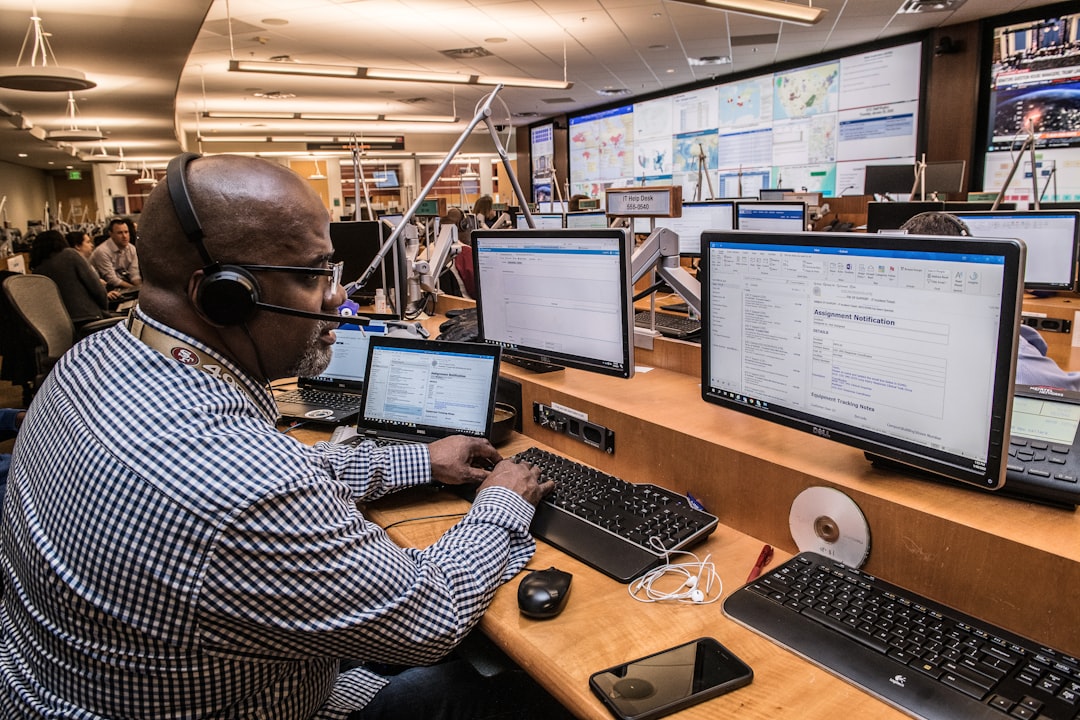
In today's enterprise IT landscape, the Zero Trust security framework is quickly becoming the norm. It's all about verifying every access request, regardless of whether it's coming from inside or outside the company's network. This shift away from older security models requires a major change within organizations. Leaders at all levels need to be on board and actively manage the transition if they want Zero Trust to be successful.
Zero Trust is built on a comprehensive, end-to-end security approach that constantly monitors and enforces security policies across the entire digital space, a crucial factor in the rise of remote and hybrid work setups. However, implementing Zero Trust is not a simple switch. Organizations must thoroughly evaluate their current security measures and develop robust management processes for devices and users. They have to be willing to move past traditional security methods and embrace a more vigilant, preventative security approach. This framework has emerged as a critical defense against the evolving threat landscape, prompting a deeper rethink of how companies protect their information.
The Zero Trust security model, which started gaining traction in the early 2010s, is becoming the standard in enterprise IT. It's a fundamental shift from the traditional "castle and moat" approach to security, where everyone inside the network perimeter was considered trustworthy. Zero Trust, in contrast, assumes no user or device is inherently trustworthy, regardless of their location. Every access request is verified, which is a major change in how organizations think about network security.
However, this shift doesn't come without its own set of challenges. Companies may find they need to allocate a significant portion – possibly 15% to 20% – of their IT budget towards adopting Zero Trust. This includes purchasing new technology, retraining staff, and restructuring processes to align with the Zero Trust philosophy. This can be a significant investment, but it's important to remember that the cost of security breaches can be far greater.
Interestingly, even with the added security layers, Zero Trust can actually lead to improved user satisfaction. Initially, there's a perception that it might add friction to users' interactions with IT systems. But, the increased security can foster greater trust in those systems, as users feel their data is better protected. This suggests that while security can sometimes be inconvenient, it's not necessarily a deterrent to usability if done correctly.
The adoption of Zero Trust is accelerating, driven by a more complex and dangerous cyber threat landscape and growing regulatory pressure. Estimates show that over 70% of large enterprises will have integrated some form of Zero Trust by 2025. This significant shift reflects a growing understanding that traditional security models are insufficient in today's digital environment.
Zero Trust security relies heavily on AI and machine learning. These systems analyze user behavior and network traffic patterns in real time to spot anomalies that trigger security protocols. This proactive, continuous monitoring is a significant enhancement over the reactive approach of traditional security.
Implementing Zero Trust successfully requires collaboration across multiple departments. IT, security, compliance, and even human resources all need to be involved in defining and enforcing policies. This holistic approach helps ensure that security is integrated into all aspects of the organization. It's not just a technology issue but one that impacts how an organization operates.
Organizations that don't implement Zero Trust face a significant financial risk. The average data breach can cost companies roughly $3.86 million, a cost that Zero Trust aims to minimize through better security. With the growing number of sophisticated cyberattacks – like ransomware and phishing – it's clear that Zero Trust is not just a trend but a necessity. This growing awareness is confirmed by surveys showing a majority of IT professionals feel safer with Zero Trust.
Beyond traditional authentication methods, behavioral biometrics is gaining ground as part of the Zero Trust approach. By analyzing unique characteristics like typing speed and mouse movements, behavioral biometrics adds an extra layer of protection that's very hard for attackers to replicate.
Transitioning to Zero Trust presents particular challenges for companies with large legacy systems. Updating old technology and integrating new, more secure solutions can be a significant undertaking, both operationally and financially. This highlights the trade-offs between embracing new technology and the effort required to modernize existing infrastructure.
The move toward Zero Trust highlights how security needs to be embedded into every aspect of an organization, not just treated as a separate layer on top of existing systems. It reflects the increasing awareness of cybersecurity threats and the need for more robust security solutions. The journey toward full adoption of Zero Trust will be complex, with both operational and financial challenges, but it is a necessary step towards creating more secure digital environments in an increasingly complex and threatening technological landscape.
7 Critical Changes in IT Operations Management Expected for 2025 From AIOps Integration to Zero-Trust Implementation - Predictive Analytics Takes Over Traditional Monitoring Methods
Within IT operations management, predictive analytics is swiftly becoming the preferred approach over traditional monitoring methods. This shift is driven by AI-powered forecasting capabilities that anticipate problems before they impact operations, transforming how organizations manage their systems. Instead of simply reacting to issues, they can now proactively address potential disruptions, leading to greater efficiency and smoother operations.
Traditional methods like statistical analysis often struggle with the immense and complex datasets that modern IT environments generate. Predictive analytics, powered by AI, excels in this area, providing a more nuanced understanding of system behavior and potential trouble spots. Organizations are increasingly embracing these capabilities because of the potential to optimize resource allocation and reduce error rates. This translates to a competitive advantage for those who adopt predictive analytics, while those sticking with traditional methods may be left behind.
This trend demonstrates a significant evolution in IT operations management. Organizations that adopt predictive analytics are choosing a more forward-thinking approach, recognizing that a reactive strategy is no longer adequate in today's complex environments. It's a clear indication that the future of managing IT effectively relies on being able to anticipate problems and adapt quickly.
The way we monitor IT systems is shifting from reactive, traditional methods to a more proactive, predictive approach powered by analytics. It's no longer just about seeing problems as they occur but forecasting them and acting ahead of time. This move towards predictive analytics is fueled by the increased complexity of IT environments and the growing volume of data we need to manage.
Traditional monitoring methods, like basic statistical analysis, have their limitations when dealing with the massive and complex datasets that modern IT generates. Think about the challenges of sifting through reams of logs from a wide range of systems to find the source of a problem. Predictive analytics, with its foundation in machine learning and AI, has the ability to analyze these complex datasets and uncover hidden patterns that traditional methods might miss. We are seeing vendors like DataDog and Dynatrace lead the way with solutions that use these advanced methods, effectively providing richer insights.
In a sense, predictive monitoring is like having an advanced warning system. It helps us better understand 'normal' system behavior and flag anything out of the ordinary. It's interesting how this approach can translate into cost savings – companies are seeing up to a 50% reduction in incident management costs by being more proactive. Moreover, the accuracy of predicting incidents is increasing significantly, some studies show over 80% accuracy, leading to fewer false alarms and a better understanding of real risks.
But it's not just about incident response. Predictive analytics can help forecast things like energy demands in industries like oil and gas, using historical data and seasonality to get a better idea of future requirements. Also, it's quite likely that the push toward zero-trust security will be deeply intertwined with predictive analytics in the future, as these systems can be invaluable in detecting anomalies in user behavior and network traffic, which helps to enforce security protocols more effectively.
One of the fascinating aspects of this shift is the integration of predictive analytics with the growing number of IoT devices. This gives us the potential for real-time insights and decision-making in situations where speed is crucial, like manufacturing or healthcare. Predictive models are becoming more sophisticated through continuous learning, which means their accuracy increases over time, allowing them to adapt to evolving environments and security threats.
Naturally, the implementation of such sophisticated systems has its challenges. Integrating predictive analytics requires a good understanding of how to leverage the available data, and there's the need to constantly refine these models to stay accurate. But the overall trend is promising and suggests a future where we can anticipate and prevent IT incidents with higher precision, leading to greater operational efficiency and a better understanding of the threats to our systems. The move toward predictive analytics seems like a logical next step in managing increasingly complex IT environments.
7 Critical Changes in IT Operations Management Expected for 2025 From AIOps Integration to Zero-Trust Implementation - Cloud Native Observability Tools Replace Legacy Monitoring Systems
The landscape of IT monitoring is undergoing a significant shift, with cloud-native observability tools rapidly replacing traditional legacy systems. These new tools offer a more flexible and scalable approach, particularly well-suited for the dynamic nature of cloud-based infrastructure. Instead of the hierarchical metrics found in older systems, cloud-native tools leverage tag-based metrics, enabling more granular insights and faster responses to incidents.
Furthermore, these tools are capable of integrating operational monitoring and observability data, offering a more holistic view of cloud-native applications. This is crucial given the dramatic increase in log data that these environments generate. While traditional IT management approaches are struggling to keep up with the demands of cloud infrastructure, cloud-native observability provides the necessary agility and responsiveness. This shift is especially important as we anticipate further changes in IT operations management, including a growing reliance on AI-driven systems and the implementation of stricter security frameworks, expected by 2025.
Adopting these advanced monitoring solutions provides numerous benefits. Real-time visibility into application behavior allows teams to address issues more efficiently and gives them the ability to keep up with the constant changes characteristic of cloud computing. In essence, it's a move from a reactive approach to a proactive one, better equipping organizations to deal with the growing complexity of their IT infrastructure.
Cloud-native observability tools are increasingly replacing legacy monitoring systems, especially in the dynamic environments of modern cloud infrastructures. Traditional systems, often designed for static, hierarchical setups, struggle to keep pace with the complexity of today's distributed applications and microservices architectures. They frequently rely on static thresholds and predefined alerts, which can miss subtle shifts in system behavior. In contrast, cloud-native observability tools analyze massive quantities of dynamic data from diverse sources, offering a far more nuanced understanding of how systems function.
This real-time insight is a key advantage of cloud-native approaches. Legacy systems, in contrast, often have substantial delays in processing data and generating alerts, leading to slower responses to critical situations. The ability to quickly identify problems is crucial for minimizing service disruptions and maintaining user satisfaction.
A notable difference is the capability of distributed tracing. While traditional tools may focus on single components, cloud-native observability uses distributed tracing to track requests across complex, multi-component systems. This capability helps pinpoint performance bottlenecks within microservice architectures, improving the overall efficiency of application monitoring. Furthermore, these tools are frequently incorporating automated troubleshooting features that can trigger corrective actions without needing human intervention. This contrasts with older systems, which necessitate manual investigation, slowing down resolution times and increasing the workload on teams.
Another compelling advantage of cloud-native tools is their capacity to improve collaboration and data sharing. Modern solutions are built with DevOps in mind, fostering shared access to observability data. Conversely, legacy monitoring systems often compartmentalize data, hindering seamless communication and coordinated responses to issues. The seamless flow of information across teams is crucial in agile development and operational environments.
Further, cloud-native observability tools often feature adaptive learning mechanisms, leveraging machine learning to automatically refine monitoring parameters as system behaviors change. This is a sharp contrast to legacy systems, which tend to operate on fixed parameters and may fail to adapt to evolving system dynamics. This ability to adapt is essential as infrastructure and applications are constantly updated and refined.
There are economic benefits too. Organizations moving towards cloud-native observability often report substantial cost reductions linked to more efficient resource utilization. Older systems, lacking deep visibility, can lead to over-provisioning of resources. In contrast, cloud-native solutions facilitate on-demand resource allocation based on actual performance data.
Cloud-native tools are also capable of tracking user interactions and behaviors in real time, offering valuable insights into user experience. Legacy systems typically lack this type of user-centric data, hindering efforts to optimize service delivery. This focus on user experience is particularly important as organizations increasingly strive to deliver seamless and intuitive services.
In addition, the flexibility of cloud-native observability supports continuous integration and continuous deployment (CI/CD) practices. Legacy monitoring tools struggle in these dynamic environments, leading to extended deployment cycles and potential downtime.
Lastly, cloud-native systems integrate security monitoring as an essential part of the observability platform. In contrast, legacy tools frequently isolate security monitoring from operational performance, resulting in potential security blind spots that can be exploited by attackers. As organizations increasingly prioritize proactive incident response, cloud-native observability offers a comprehensive security posture by combining operational and security monitoring.
The transition to cloud-native observability represents a major shift in how organizations manage and understand their IT environments. It's a clear example of how tools must evolve to adapt to the complexity and rapid pace of change in today's dynamic IT landscape. It will be fascinating to see how these tools and practices further evolve in the coming years.
7 Critical Changes in IT Operations Management Expected for 2025 From AIOps Integration to Zero-Trust Implementation - IT Service Desk Automation Reaches 60% Resolution Rate
Automation within IT service desks has reached a notable milestone, resolving 60% of support requests automatically. This advancement showcases how technology is reshaping the way support teams operate. Using AI and automation to handle routine tasks is allowing service desks to respond much quicker and, hopefully, leading to happier users. This is part of a larger shift where companies are employing AIOps and generative AI to automate and streamline various IT processes, improving efficiency and reducing manual effort.
While faster response times and a reduction in workloads are positive developments, managing and adapting to these emerging technologies is not without its difficulties. Organizations will need to carefully consider how automation integrates with their existing processes and be ready to adapt to the changing demands of these tools. As we look toward 2025, this trend of IT automation is likely to strengthen, creating new avenues for smarter, more effective IT operations. The ability to integrate these technologies well is likely to become a key differentiator for companies in the coming years.
IT service desk automation is making strides, with reported resolution rates reaching 60%. This isn't just about speeding up fixes; it suggests these automated systems are effectively handling a large chunk of standard support requests. This frees up human agents to tackle the trickier problems that require more nuanced judgment.
One surprising finding is that the implementation of these automated systems has led to a noticeable reduction in the number of repetitive incidents. Some organizations report reductions of up to 50%, which is fascinating. This shift in incident volume means IT teams have more time to focus on strategic projects instead of being bogged down with routine issues. It's an interesting example of how automation can shift the workload, allowing teams to concentrate on higher-value work.
This increased efficiency isn't just theoretical; it translates to noticeable boosts in staff productivity. Studies show that, with automated systems handling the bulk of standard inquiries, IT staff experience a productivity increase of around 30%. It's logical: less time spent on rote tasks means more time for meaningful work. While this sounds great for the IT team, I wonder if this impacts the role of IT staff over time.
Surprisingly, customer satisfaction scores have also seen an uptick in organizations that have integrated service desk automation. This is likely connected to faster response times; automated systems can often deliver instant feedback or resolve basic problems quicker than a human agent. It's noteworthy that this seems to translate to a better user experience, even though the process might be less "personal". This seems to suggest that for certain tasks, speed and efficiency trump personal interaction, at least to some degree.
These automated systems also offer inherent scalability benefits. During peak times – like after a system update or a large-scale outage – they can gracefully handle the increased influx of requests without needing additional staff. This is a huge advantage compared to traditional service desks, which often struggle to cope during times of high demand. However, I'd be interested in learning if this scalability benefit has any limitations.
It's not a static system either. Many automated service desks leverage machine learning. As these systems handle more incidents, they refine their processes and learn to anticipate future requests. This constant learning translates to both improved resolution rates and a greater accuracy in meeting user needs. This raises some interesting questions about the evolution of these systems, and if they'll develop capabilities we don't fully understand at this point.
The cost benefits are also worth mentioning. Organizations employing automated service desks are realizing savings of around 25% in operational costs. This is mainly due to the reduction in manual labor and the improved efficiency of incident resolution. This makes a strong business case for automation, but the implementation can be complex. We need to be aware of the potential downsides alongside these positive outcomes.
We also see a clear trend towards integrating these automated service desks with other IT management tools. This creates a unified platform for managing operations, allowing for better conflict resolution and more comprehensive system analysis, which, in turn, can further improve resolution rates. It seems like a logical step, as it creates a more cohesive approach to addressing issues across an IT environment, but I wonder how compatible different tools are.
Many automated systems provide self-service options, which allow users to resolve minor issues on their own. This directly contributes to that 60% resolution rate, as it empowers users to address their problems independently. This reduces the load on the service desk team, but does it lead to users becoming less reliant on the IT department?
Despite the impressive statistics, challenges still persist. There are situations, especially those requiring complex troubleshooting or human judgment, where automation still falls short. The ability to find that balance between automated solutions and human oversight will be crucial to maintain the quality of service while also ensuring complex issues are addressed effectively. It's important not to get carried away by the enthusiasm for automation and to ensure a healthy level of scrutiny is maintained. This area is full of potential, but also risks, and a measured approach is needed.
More Posts from specswriter.com: